학술논문
물질의 상태 분류에 대한 과학교사와 머신러닝 모델의 분류 결과의 비교 분석
이용수 79
- 영문명
- Comparative analysis of classification results between science teachers and machine learning models about classifying state of matter
- 발행기관
- 학습자중심교과교육학회
- 저자명
- 최정인 백성혜
- 간행물 정보
- 『학습자중심교과교육연구』제23권 4호, 363~379쪽, 전체 17쪽
- 주제분류
- 사회과학 > 교육학
- 파일형태
- 발행일자
- 2023.02.28
4,840원
구매일시로부터 72시간 이내에 다운로드 가능합니다.
이 학술논문 정보는 (주)교보문고와 각 발행기관 사이에 저작물 이용 계약이 체결된 것으로, 교보문고를 통해 제공되고 있습니다.
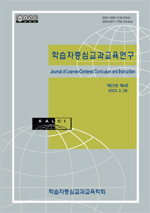
국문 초록
목적 본 연구는 물질의 상태 분류에 대한 초⋅중등 과학교사들인 연구 참여자들과 개발된 머신러닝 모델의 분류 결과를 비교 분석하여 분류의 결과가 불일치하는 상황의 원인을 확인하고, 그 결과를 토대로 물질의 상태 분류 학습에 줄 수 있는 교육적 함의를 찾고자 하였다.
방법 이를 위하여 중부권 소재 사범대학의 교육대학원에 재학 중인 초⋅중등 과학교사 31명을 대상으로 물질의 상태 분류 활동을 수행하고 의사결정 트리 알고리즘을 적용한 머신러닝 모델을 구축하였다. 그리고 정확도, F1-score, 정밀도, 재현율 등 모델의 성능 평가를 실시하였다.
결과 개발된 물질의 상태 분류 머신러닝 모델의 분류 정확도는 0.820, F1-score는 0.820, 정밀도는 0.826, 재현율은 0.820으로 나타났다. 또한 과학교사들이 분류한 결과와 머신러닝 모델의 분류 결과가 불일치하는 정도는 순물질이나 균일 혼합물보다 불균일 혼합물에서 크게 나타났다. 이러한 불일치는 연구 참여자들이 물질의 상태를 분류할 때 거시적 관점과 미시적 관점의 분류기준을 일관적으로 적용하지 않거나, 특정 물질은 특정 상태라는 개념을 미리 가지고 물질의 상태를 분류하기 때문에 나타나는 현상으로 분석되었다. 그리고 의사결정 트리 알고리즘의 시각화를 통해 학습 상태를 드러내는 도구로서의 유용성을 확인하였다.
결론 연구 결과를 토대로, 선행연구에서 지적한 학생들의 물질의 상태 분류 과정에서 드러나는 혼란의 원인을 찾아볼 수 있었으며, 머신러닝은 효과적인 학습상태 진단도구가 될 수 있으므로 이를 활용할 수 있도록 교사교육이 필요함을 제안하였다.
영문 초록
Objectives This study compares and analyzes state classification results of matter between developed machine learning model and research participants who are elementary and middle school science teachers, identifies the cause of the situation in which classification results are inconsistent. And based on the results, we tried to find educational implications that can help learn state classification of matter.
Methods For 31 elementary and middle school science teachers enrolled in the Graduate School of Education at the College of Education located in the central region, matter classification activities were performed and a decision tree algorithm was applied to the machine learning model. And the effectiveness of the program was confirmed through model performance evaluation such as accuracy, F1-score, precision, recall.
Results The classification accuracy of developed machine learning model for classifying state of matter was 0.820, the F1-score was 0.820, the precision was 0.826 and the recall was 0.820. In addition, the degree of discrepancy between the classification results of science teachers and the classification results of the decision tree algorithm was larger in heterogeneous mixtures than in pure matters or homogeneous mixtures. This discrepancy was analyzed as a phenomenon that occurs because science teachers do not consistently apply the classification criteria from the macroscopic and microscopic perspectives or do have the concept that a specific matter is a specific state in advance when classifying the state of matter. Based on the results of these studies, the cause of confusion revealed in the process of classifying the state of matter pointed out in previous studies was found.
Conclusions Based on the research results, it was possible to find the cause of confusion revealed in the process of classifying the state of matter of students pointed out in previous studies, and since machine learning can be an effective tool for diagnosing learning conditions, it is suggested that teacher training is needed to utilize it.
목차
Ⅰ. 서론
Ⅱ. 연구 방법
Ⅲ. 연구 결과 및 논의
Ⅳ. 결론 및 제언
참고문헌
키워드
해당간행물 수록 논문
- 아동의 행복과 협동심의 종단적 상호관계
- 키워드네트워크 분석을 통한 창의적 교수법 개발 프로그램 내용 특성 비교 분석
- 간호대학생의 임상실습 교육 유형에 따른 문제해결능력, 의사소통능력, 자기주도적 학습능력 비교
- 초등학교에서 역할놀이의 도덕교육적 의미 연구
- 지역 정체성을 통해 바라본 초등 음악교과서 분석
- 국내 일반대학 강의평가 선행연구에 대한 문헌 고찰
- 기혼 여성 상담자의 전문성 발달 경험에 관한 현상학 연구
- 초등학교 교사의 학부모 상담 관련 국내 연구동향 분석
- 수직선 및 수체계, 실수의 성질 의미와 관계에 대한 중학생의 오류와 그 오류 사이의 관계
- 종합병원 간호사의 소명의식, 긍정심리자본이 그릿에 미치는 영향
- Effects of Explicit Reading Strategy Instruction on High and Low English Proficiency Learners
- 상담심리 전공 대학생을 위한 핵심역량 증진 집단상담 프로그램이 공감 능력과 대인관계 유능성에 미치는 효과
- 영아교사의 자아탄력성, 정서지능 및 사회적 지지와 민감성 간의 구조분석
- SBAR를 활용한 인수인계 교육 프로그램이 간호학생의 비판적 사고성향, 자기효능감, 의사소통능력에 미치는 영향
- 원불교 수행자의 ‘마음공부’에 나타난 성찰경험 내러티브 탐구
- 청각장애인의 읽기 이해력 관련 연구 동향
- 미디어중독 수준에 따른 아동의 교사-아동 간 친밀감과 갈등, 사회적 위축, 삶의 만족도 간 구조분석
- 아동 간호 시뮬레이션 실습교육이 간호학생의 임상수행능력, 자신감, 학습만족도, 학업적 효능감에 미치는 효과
- 태권도 지도자 유형에 따른 여가만족 및 여가활동 제약에 대한 탐색적 분석 연구
- 사진을 활용한 시 쓰기 교육 연구
- 보육교사의 교사효능감과 행복의 관계에서 사회적 지지의 매개효과
- 지역교육과정 정체성 논의
- 대학생의 행동억제가 사회불안에 미치는 영향
- 정서표현 양가성이 외로움에 미치는 영향
- 청소년의 내현적 자기애와 스마트폰 중독과의 관계
- 뷰티 전공 청소년의 학습동기, 스마트 미디어 활용의 학업적응 간의 집단별 군집분석
- 필자의 텍스트 반성에 대한 화용론적 접근
- 초중고 학생들의 학교생활만족 관련 변인들 간 구조적 관계
- 대학생의 메타인지와 소명의 관계에서 학업성취도와 진로준비행동의 매개효과
- 코로나19 시기 대학생의 학습경험 유형 및 특징 분석
- 인터넷 시대 한국어 교재 연구에 대한 일고찰
- 대학생의 온라인 수업만족도 및 학습성과 영향 요인 분석
- 초등영어 CLIL 수업을 위한 비계 지원 전략
- 예비영어교사들을 위한 과정 중심 평가 기반 전공 교과목 수업 방법 실행연구
- 상담수퍼바이저 역량모형 개발을 위한 연구
- 생태전환교육에 관한 체계적 문헌 고찰
- 기술변화에 따른 산림 분야 종사자가 인식하는 일자리 및 업무변화
- 초등교사 스키마니아 가족의 첫 번째 겨울나기
- 자기회귀 교차지연 모형을 적용한 사회적 상호작용 불안과 우울의 관계
- 물질의 상태 분류에 대한 과학교사와 머신러닝 모델의 분류 결과의 비교 분석
- 영국 엘리자베스 2세 여왕의 성장기 교육에 대한 연구
- 복잡한 데이터를 활용한 모델 기반 탐구에서 나타난 예비교사의 과학적 상상력 발현 및 인식
- 한국어 교육을 위한 의성어⋅의태어 선정과 교육방안 연구
- 중학생의 프라이버시 리터러시 향상을 위한 최신 개인정보 유출 사례 기반의 정보보호 교육 자료 개발
- 초등 도덕 교과서 삽화에 드러난 ‘성역할 및 성 형평성’ 연구
- 코로나19(COVID-19) 장기화로 인한 대학생의 피로, 우울, 삶의 질이 여가만족도에 미치는 영향
- ‘바른소리’ 교육프로그램 참여 노인의 언어기능 발달과 학습경험에 관한 연구
- 간호대학생의 플립러닝을 적용한 지역사회간호학 교육이 학습동기, 학습관련 자기주도성, 자기효능감, 비판적 사고성향, 문제해결력 및 학습만족도에 미치는 효과
- 과학 교사의 PCK 국내 연구 동향 분석
- Biesta의 실천적 상호주관성 개념에 비추어 본 기술기반 미래학교 알트스쿨의 비판적 탐색
- 실생활 맥락의 조건 명제에서 보이는 고등학생의 추론에 관한 연구
- 변성암 분류 과정에서 정보 제공 정도에 따른 지구과학 교사들의 시선 특성과 암석 판별의 정확성 분석
- 초등예비교사의 교직선택동기, 자기주도적 학습이 대학생활만족도에 미치는 영향
- 자폐스펙트럼 자녀 장애를 기르며 성장하는 아버지의 경험
- 미용 학습자가 지각한 교수자의 훈육, 신뢰와 학습참여동기의 관계 연구
- OECD 학습 나침반 2030에 기반한 청소년 기업가정신 교육 프로그램 개발
- 학습자중심교과교육연구 제23권 4호 목차
- 일터학습개념의 전문대학 적용사례 효과성 검토연구
- 여성 자살생존자의 삶에 대한 내러티브 연구
참고문헌
교보eBook 첫 방문을 환영 합니다!
신규가입 혜택 지급이 완료 되었습니다.
바로 사용 가능한 교보e캐시 1,000원 (유효기간 7일)
지금 바로 교보eBook의 다양한 콘텐츠를 이용해 보세요!
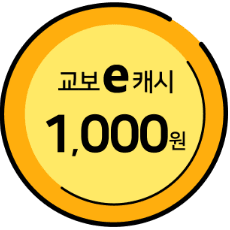