학술논문
의사결정나무의 최적 분할 및 가지치기 옵션
이용수 184
- 영문명
- Optimal Splitting and Pruning Option in Decision Tree Classification
- 발행기관
- 인문사회과학기술융합학회
- 저자명
- 이지현(Jihyun Lee) 김기태(Gitae Kim)
- 간행물 정보
- 『예술인문사회융합멀티미디어논문지』9권 5호, 907~914쪽, 전체 8쪽
- 주제분류
- 사회과학 > 사회과학일반
- 파일형태
- 발행일자
- 2019.05.31
4,000원
구매일시로부터 72시간 이내에 다운로드 가능합니다.
이 학술논문 정보는 (주)교보문고와 각 발행기관 사이에 저작물 이용 계약이 체결된 것으로, 교보문고를 통해 제공되고 있습니다.
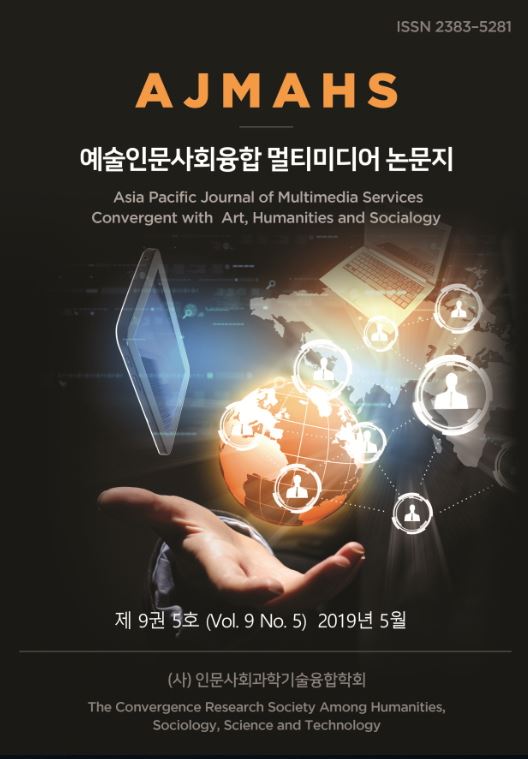
국문 초록
의사결정나무 분류는 변수 즉 속성(Attribute)을 노드로 하여 나무를 형성해 가면서 데이터의 패턴과 규칙을 찾는 방법으로 속성인 노드에서 가지를 뻗어 나가는 분할(Splitting)방법과 속성들의 순서를 정하는 것으로 알고리즘이 구성된다. 또한 과적합을 방지하기 위해 의사결정나무의 크기를 줄이는 가지치기(Pruning)를 수행한다. 분할방법에 따라 의사결정나무의 알고리즘이 CART, CHAID, C5.0으로 나뉘고 가지치기를 하기 위한 다양한 옵션이 존재한다. 의사결정나무 분류 방법은 분할방법과 가지치기의 방식에 따라 성능에 차이가 존재한다. 또한 데이터의 종류에 따라 성능이 달라진다. 우리는 본 논문에서 어떠한 분할방법과 가지치기 옵션이 데이터의 형태에 적합한지를 비교 분석하여 데이터 형태에 따른 최적의 분할방법과 가지치기 옵션을 제시한다.
즉, 데이터 및 변수를 크기별로 분류하여 어떠한 분할 알고리즘 및 가지치기가 최적조건인지 분석한다. 또한 불균형 데이터에 대해서도 조사하여 불균형 정도를 네 단계로 구분하여 불균형 정도별로 분할 알고리즘과 가지치기에 대한 분석을 실시하여 최적의 조건을 찾는다.
본 연구결과는 어떠한 형태의 데이터가 주어질 때 유형에 따라 어떤 분할방법과 가지치기를 사용하는 것이 가장 적절한지 제공할 수 있어 다양한 문제와 분야에 응용될 수 있는 중요한 자료로 사용될 수 있다.
영문 초록
Decision tree classification is a method of finding patterns and rules of data by forming a tree by using variables(attributes) as nodes. In this method, an algorithm is formed by splitting a property that extends a branch at an attribute node and determining the order of attributes. Also, pruning is performed to reduce the size of the decision trees to prevent overfitting. The algorithm of decision tree is divided into CART, CHAID, and C5.0 according to the partitioning method. Also, performance varies depending on the type of pruning and the type of data.
In this paper, we propose the optimal splitting and pruning conditions for types of data set by analyzing the performances of splitting and pruning combinations to solve various types of data set. For the analysis, the data type is categorized by small, medium and large sets in terms of size of records or size of attributes (number of variables or dimensions) or continuous or nominal data sets. We also investigate the optimal options of algorithm and pruning for imbalanced data set with classifying into four categories.
Contributions of this paper are three folds. First, we present a comprehensive survey on the splitting and pruning methods for decision tree classification. Second, this paper provides optimal conditions of splitting and pruning methods for any data set in decision tree classification. Lastly, we show the viability of applying for many problems or fields.
목차
1. 서론
2. 기존연구 고찰
3. 데이터 유형별 분할 및 가지치기 최적조건
4. 결론
해당간행물 수록 논문
- 성별에 따른 근로자의 삶의 질에 영향을 주는 요인
- 대학생의 당 관련 영양지식과 구강건강행태와의 관련성
- 장기요양기관(시설급여) 평가의 효과성 검증
- 변혁적 리더십이 조직구성원의 혁신행동에 미치는 영향 - 직무열의의 매개효과 -
- 데이비드 오번의 증명 에 나타난 여성수학자의 광기분석
- “과학과 현대”의 시: 월트 휘트먼과 속도기계
- 국가 소방자격관리의 다양화 방안
- 팀 기반 학습에서 유아교육과 학생의 그릿과 정서지능 문제해결력의 관계
- 사이버공간에서 심리사회적요인 및 이용자태도와 온라인 활동을 매개로 한 사이버폭력에 관한 연구
- 리좀의 특성을 이용한 남북 접경지역 일대 복합문화공간 디자인
- 중학생 ‘소확행’척도 개발 및 타당화 연구
- 이공계 대학 유학생을 위한 수학교육 프로그램 설계 및 적용
- 대학 일반영어 플립드 러닝 수업 방식의 탐색적 사례연구
- 간호 대학생의 첫 임상실습 경험에 관한 현상학적 연구
- 공감 커뮤니케이션을 위한 인터랙티브 라이팅 유도사인 시스템 개발 연구
- 키스트로크 다이나믹스가 적용된 볼륨버튼 기반의 패스워드 인증 기법
- 관광자원 개발정책으로서 관광구조물 건설의 경제적 효과에 관한 연구
- 초등학교 고학년의 언어적 구강건강문해력에 관한 조사연구
- 다문화가정의 삶의 질 향상을 위한 다문화지원 서비스 정책 발전 방안 연구
- 충북지역 장애아동 보호자의 특수학교 구강보건사업에 대한 인식 조사
- 의사결정나무의 최적 분할 및 가지치기 옵션
- 남성 육아휴직에 대한 간호학생들의 인식 : Q 방법론
- 서울특별시 노후 건축물의 트래킹에 의한 전기 시설물의 전기화재 증가 추이 가능성 2020년 이후 예측분석
- 한국어교육 정책의 발전방향 고찰
- 대학생의 인성교육을 위한 교양교육과정 개발 : E대학을 중심으로
- 노인종합복지관을 이용하는 고령자의 심리상태가 삶 만족도에 미치는 영향
- 모바일 폰의 관성 센서를 사용한 에너지 소모량 추정 모델
- 부부친밀감과 가족지지가 노년기 배우자 부양의식에 미치는 영향 중년세대와 노년세대의 비교를 중심으로
- James Ensor(1860-1949)의 예술 작품과 치료적 요인 분석: C. G. Jung의 분석심리학을 중심으로
- 우울경험 대학생을 위한 사티어변형체계치료 집단프로그램의 효과
- 영재 선발의 심층면접에서 인성에 대한 현장 교사들의 인식 분석
- 간호대학생의 자기주도학습능력 영향요인
- 다문화가정 귀화 이주민 대상 한국사 교육과정 성취기준 개발을 위한 탐색적 연구
- 제4차 산업혁명과 이러닝 콘텐츠의 효과적인 품질관리 시스템 구축
- 교양전공 교육과정 개발 연구: 경남대학교 사례를 중심으로
- 발레공연 관객창출을 위한 마케팅 효과 검증 연구: 소셜 라이브 스트리밍을 중심으로
- 텍스트마이닝 방법론을 활용한 미래교육 키워드 분석
- 대학생의 의사소통과 생활스트레스가 대학생활적응에 미치는 영향
- 연예인과 대중의 갈등구조와 균형 전략 비협조적 게임모형을 통한 접근
-
스토리텔링과 롤 플레이 기법을 통한 비즈니스 중국어 말하기 교육방안 탐색 -드라마
를 중심으로- - ICT가 산업별 경제성장에 미치는 영향: 사하라사막이남 아프리카 지역을 중심으로
- 교육감 소속 지방공무원 심사승진제도 개선방안에 대한 사례연구
- 여대생의 성적 자기주장이 임신인식에 미치는 영향
- 유사 사이트 분석을 통한 청년층 대상 온라인 공공고용서비스 개편 방안
- 어린이집 선정에 대한 영아 어머니의 경험
- 재난발생시 재난약자 지원에 관한 연구 -복지 대피소를 중심으로-
- 재한 몽골 유학생의 중도 탈락 경험에 관한 질적 연구
- 온라인 P2P 대출의 연체율과 부실률 결정요인에 관한 연구
- 배려와 돌봄의 학급공동체 형성 프로그램이 초등학생의 또래관계 및 학교생활태도에 미치는 영향
- 청각자극에 의한 부정적 이미지 자극의 마스킹효과 연구
- 성공적인 초등영어 독자의 웹기반 영어 읽기에 나타난 뉴리터러시 기능 및 온라인 읽기 전략 탐구
- 아이돌보미의 영유아 감염예방에 미치는 영향 -지식과 실천 중심으로-
- Deviene의 학습기술 구성요소 기반 복합적 교수-학습 방법이 학습성과에 미치는 효과
- 간호대학생의 또래애착, 우울이 인터넷 중독에 미치는 효과
- 학교폭력 가해 청소년의 자아정체감 탐색을 위한 집단프로그램 적용 이야기치료에 기초하여
- 한국교통대학교 Solar Car 구동 시스템 분석 및 설계
- 컴퓨팅 사고와 문학 : 『에밀리에게 장미를』
- 건설적 발언의 선행요인들과 경로모형의 탐색
- 공학계열 대학생의 좋은 수업에 대한 인식 유형 분석
- 간호대학생의 우울, 월경전후 대처 및 증상 간의 관계
- FPI의 문학치료와 동작중심 표현예술치료의 융합적 활용 - 성인 여성 참여자들을 중심으로
- 사회복지서비스의 책임성에 관한 연구 경향 분석
- 유아의 공감능력 검사 도구(AST)의 국내 사용변천
- 북한이탈여성의 스트레스와 사회적 지지가 생활만족도에 미치는 영향
- 고령자의 구직활동 경험에 관한 현상학적 연구
- 지역 연계 인턴십을 통한 현장 중심의 진로교육 방향 연구 -청소년 직업 체험 LTI(Learning Through Internship) 프로젝트 운영을 중심으로-
- 온라인 뉴스 댓글을 통해 본 신고리 5·6호기 공론화위원회의 주요 이슈
- 한국어 교사의 심리적 소진에 대한 연구
- 보살핌과 조직지향 시민행동의 효과경로에서 조직주인의식과 자부심의 매개효과 : 중국을 중심으로
- 예비교사가 기대하는 실습지도 -대학, 실습기관, 실습지도교사의 역할을 중심으로
- 개인, 가족, 환경요인이 청소년의 자아존중감에 영향을 미치는 요인
- SNS중독성현상(조절실패, 몰입 및 내성, 부정정서의 회피, 가상세계지향)에 관한 연구
- 고등학생의 식습관이 건강상태에 미치는 영향에 관한 연구
- 자동차 그래픽 시뮬레이터에서 동시자극에 대한 페퍼민트 향의 효과
- 아르메니아 정교회 사원건축의 형성
- 만성 폐쇄성 폐질환 환자의 국내 연구 동향 분석: 연구 주제 및 관련 측정도구를 중심으로
- 핵심역량 기반 비교과프로그램 효과성연구를 바탕으로 환류체계 고도화
- 변혁적 리더십이 조직구성원의 조직몰입에 미치는 영향 - 상사와의 프렌드 십과 혁신행동의 매개효과 -
- 저소득 청소년이 인식한 지역사회환경이 진로정체감에 미치는 영향: 학교생활적응의 매개효과
- BuddyMirror: 이미지 메이킹 서비스를 지원하는 스마트 미러
- 한국 시문학에 나타난 탈물질주의 의식: 촛불 혁명을 다룬 시들을 중심으로
- 동화를 활용한 공감능력 증진 프로그램 효과
- 건조환경의 쾌적성과 미기후가 도시공간의 외부활동 지속에 미치는 영향 분석
- 중국 연변지역 내 해외취업가정 청소년의 우울・불안과 학교적응의 관계에서 자아정체감의 조절효과
- 사회적 지지가 암 진단을 받은 환자의 외상후 스트레스에 미치는 영향
- 학생 공부인생의 내러티브가 학교교육 및 교육과정 개발자에게 주는 시사점
- ICT 기반 SW 교육용 로봇 시스템을 활용한 교육 프로그램이 학업 흥미도에 미치는 영향
- 컴퓨터과학 전공 대학생의 진로태도 성숙도에 영향을 미치는 요인
- 학습포트폴리오를 활용한 학습컨설팅이 대학생의 시간관리능력 및 시간관에 미치는 효과
- 경련성 감소를 위한 치료적 중재 연구
참고문헌
관련논문
사회과학 > 사회과학일반분야 BEST
더보기사회과학 > 사회과학일반분야 NEW
- Journal of Asia Social Science Vol.11 No.2 목차
- Pentadic Criticism of T.S. Eliot’s Plays
- An Analysis of the Role of Religion in China's Foreign Exchanges from the Perspective of the Community of Human Destiny
최근 이용한 논문
교보eBook 첫 방문을 환영 합니다!
신규가입 혜택 지급이 완료 되었습니다.
바로 사용 가능한 교보e캐시 1,000원 (유효기간 7일)
지금 바로 교보eBook의 다양한 콘텐츠를 이용해 보세요!
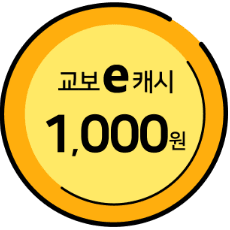