학술논문
공급 사슬 티어 예측을 통한 LSTM 네트워크의 성능 평가
이용수 13
- 영문명
- Performance Evaluation of LSTM Network through Supply Chain Tier Prediction
- 발행기관
- 인문사회과학기술융합학회
- 저자명
- 박경종(KyoungJong Park)
- 간행물 정보
- 『예술인문사회융합멀티미디어논문지』9권 8호, 791~800쪽, 전체 10쪽
- 주제분류
- 사회과학 > 사회과학일반
- 파일형태
- 발행일자
- 2019.08.31
4,000원
구매일시로부터 72시간 이내에 다운로드 가능합니다.
이 학술논문 정보는 (주)교보문고와 각 발행기관 사이에 저작물 이용 계약이 체결된 것으로, 교보문고를 통해 제공되고 있습니다.
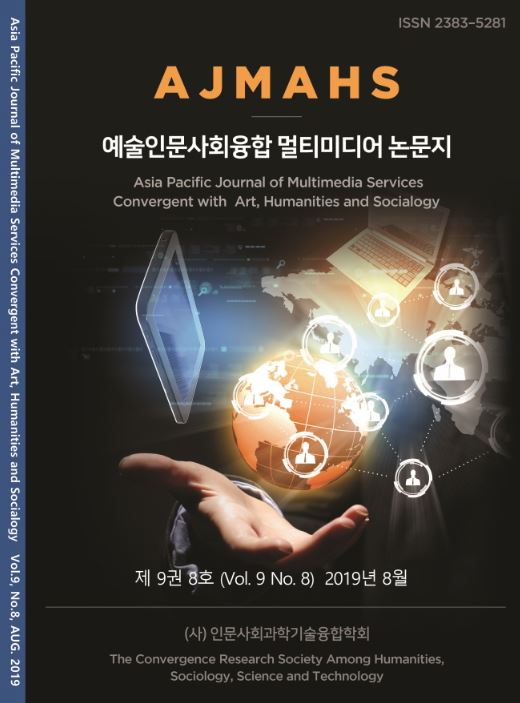
국문 초록
딥 러닝의 성능을 개선하기 위해 사용하는 대표적인 두 종류는 차별적인 네트워크 알고리즘을 사용하는 방법과 실행 프로세스에 변화를 주는 방법이다. 본 연구는 이 중에서 사용하는 네트워크의 알고리즘에 따라 딥 러닝의 성능에 차이가 발생하는지를 확인하고자 한다. 네트워크 알고리즘을 사용하는 방법은 딥 러닝 네트워크 알고리즘의 학습을 위해 어떤 알고리즘을 선택할 것인가에 관한 것이다. 본 연구는 공급 사슬의 티어 예측에 4 종류의 딥 러닝 네트워크 알고리즘을 적용하고 성능 평가를 통해 가장 적절한 알고리즘을 선정하고자 한다. 본 논문에서 성능 평가를 위해 사용되는 딥 러닝 네트워크 알고리즘은 lstmLayer, bilstmLayer, Deeper lstmLayer, Deeper bilstmLayer이며 동일한 실험 조건을 가정한다. 본 연구의 실험 결과는 사용하는 네트워크 알고리즘에 따라 수행도에 차이가 있음을 보여준다. 구체적인 실험 결과는 lstmLayer 계열보다는 bilstmLayer 계열이 더 성능이 우수하며, lstmLayer와 Deeper lstmLayer는 성능 차이가 없고, bilstmLayer 보다는 Deeper bilstmLayer가 더 성능이 우수하다. 결론적으로, 공급 사슬의 시계열 데이터 정보를 딥 러닝에 사용하는 경우는 Deeper bilstmLayer가 가장 효율적임을 알 수 있다.
영문 초록
The two typical types used to improve the performance of deep running are the method of using discriminatory network algorithms and the method of changing the execution process. The purpose of this study is to find out whether there is a difference in the performance of deep learning according to the algorithm of the network used. The method of using the network algorithm is about which algorithm to choose for learning of the deep learning network algorithm. In this study, four types of deep running network algorithms are applied to the tier prediction of the supply chain and the most appropriate algorithm is selected through performance evaluation. Deep learning network algorithms used for performance evaluation in this paper are lstmLayer, bilstmLayer, Deeper lstmLayer, Deeper bilstmLayer and assume the same experimental conditions. The experimental results of this study show that performance varies depending on the network algorithm used. Specific test results show that the bilstmLayer family has better performance than the lstmLayer family, and there is no difference in performance between lstmLayer and Deeper lstmLayer, and Deeper bilstmLayer has better performance than the bilstmLayer. In conclusion, if the time series data information of the supply chain is used for deep running, Deeper bilstmLayer is the most efficient.
목차
1. 서론
2. 기존 연구 고찰
3. 공급 사슬 모델
4. 실험 및 결과
5. 결론
키워드
해당간행물 수록 논문
- 우리나라 중소기업의 무역기술장벽(TBT) 회피, 제거 및 완화전략에 대한 연구방법론 제안
- 행복 및 안녕감 의미 단어에 대한 빅 데이터 활용 기초연구
- The Effect of the Teaching Ethics of the Pre-service Child Care Teachers on their Happiness
- 광주민주화운동 관련 도덕적 손상 연구
- 중국의 소비자 적대감이 국가이미지, 한국화장품의 구매의도 및 구매만족에 미치는 영향
- 일본의 재해 대피 표지판에 대한 한국인 관광객의 이해 증진을 위한 딥러닝 기반 의미 전달 기법
- 제주 지역 체류 예멘 난민 신청자를 둘러싼 갈등요인에 관한 연구
- 가정폭력발생원인과 인식정도에 관한 연구
- 일 지역 취약계층 노인의 신체화 심리적 안녕감이 심리적 복지감에 미치는 영향
- 무기체계에 적용 가능한 적응형 시험평가에 관한 연구
- 부모양육태도와 청소년 게임과몰입 연구 : 도피이론을 중심으로
- 간호대학생의 임상수행능력에 영향을 미치는 요인
- IoT 환경의 스마트 에이전트를 위한 이더리움 블록체인 기반의 협업 워크스페이스
- 치매(인지증)가족의 노후준비가 행복감에 미치는 영향 - 자기효능감의 매개효과 -
- 자아존중감과 학교생활만족도가 대학생 스트레스에 영향을 미치는 요인
- 재가요양보호사의 직무스트레스와 이직의도의 관계에서 직업의식 매개효과 검증
- 노인 회상치료에 관한 체계적 문헌 고찰: 증거기반 실천과정 적용
- 사회적 책임 공시 기업의 결정요인 분석
- 중년기의 자아존중감 및 가족기능이 건강증진행위에 미치는 영향
- 영화 ‘국제시장’대사 속 한국 문화교육 및 수업설계 연구
- 도이 머이(Doi Moi) 이후 베트남 교육에 관한 고찰
- 안전동요를 활용한 유아 안전교육 프로그램의 효과성 검증 연구
- 공감 커뮤니케이션 모형과 관여수준
- 정서지능이 자기결정성과 학습몰입에 미치는 영향
- 중국신세대의 직업가치관이 이직의도에 미치는 영향과 직무만족의 조절효과에 관한 연구
- 한국과 영미권의 데이팅 관습에 대한 교차문화적 자각력에 관한 연구: 영화 프라임 러브를 중심으로
- 대규모 행사 시 교통대책 수립 방법 및 사례 연구
- 예비유아교사의 주관적 안녕감에 영향을 미치는 관련변인들간의 구조분석 교직적성 교사효능감 교육신념을 중심으로
- 뇌교육 기반 창의성 계발 프로그램이 창의적 능력 및 창의적 인성에 미치는 영향
- 신학생의 행복가치관과 목회자 정체성 관계에 미치는 성경공부와 기도생활의 조절효과
- 중·고령자의 노년기 일 희망 여부 예측요인에 관한 연구
- 한·중 기업가정신교육이 창업만족도에 미치는 영향에 관한 연구 - 기회형창업의 매개효과를 중심으로 -
- 디자인 씽킹을 적용한 3차원 교육프로그램 개발 모형 탐색
- 여성건강간호학 임상실습 후 간호대학생의 전문직자아개념, 임상실습만족도, 임상실습스트레스, 임상수행능력에 관한 연구
- 대학생의 지각된 스트레스와 자살행동의 관계
- AccountEOS : EOS 블록체인 응용 어플리케이션을 위한 어카운트 관리 도구
- 도심지역 퇴행성 무릎 관절염 환자들의 민간치료요법에 미치는 영향 요인
- 읍면/동별 정신 건강이 건강행태를 매개로 하여 삶의 질에 미치는 영향 -2016년 국민건강영양조사 데이터 활용
- 도약? 또는 추락? : IPA를 활용한 골프장 사업 성공을 위한 접근법
- 공기청정기의 소음 특성 측정을 통한 환경 소음 영향 민감도 분석
- 메이커 사례 및 인식조사를 통한 메이커 활성화 및 성공 요인 분석
- 간호대학생들의 신체활동 영향요인: 일개대학
- 업무우수자의 에니어그램 성격유형별 직무만족에 관한 연구
- 부적응 유아 관련 국내연구동향 분석
- 영아교사의 의사소통능력이 이직의도에 미치는 영향: 교사효능감과 직무스트레스의 매개효과를 중심으로
- 공급 사슬 티어 예측을 통한 LSTM 네트워크의 성능 평가
- 간호대학생의 자기효능감 유머감각 인지적 정서조절과 대학생활적응과의 관련성
- 담론적 대상으로서 컴퓨터아트
- 청소년기 여학생의 월경전증후군 영향요인
- A Study on Orientations & Properties of 2015 Revised English Education Curriculum in Korea
- 심상 시치료 프로그램이 노인의 정신건강에 미치는 효과
- 직무스트레스와 사회적 지지가 복지기관 근로자의 자아존중감에 미치는 영향
- 지역아동센터 이용 아동의 서비스만족도와 심리적 복지감 사이의 관계
- 인공지능의 이론으로서 연결주의에 대한 재평가: 체계성 문제에 대한 연결주의의 인과적 설명의 가능성
- 철학의 관점으로 본 시대별 동물윤리와 태도변화의 고찰
- 한국 중·고령자의 직업교육요구 실태에 관한 연구: 은퇴 이후 일하기를 희망하는 중·고령자를 대상으로
- 청소년의 정보통신 윤리의식 수준과 선플활동 경험과의 관계
- 컴퓨터기반 리듬 타이밍 훈련이 혈관성 경도인지장애 환자의 기억기능에 미치는 효과
- 그림책을 활용한 극놀이 프로그램 개발 및 효과
- 자하하디드(Zaha Hadid) 작품에 나타나는 은유적 표현에 관한 연구
- 중년여성의 평생교육 참여 동기와 자기효능감이 삶의 질에 미치는 영향
- 순수한 NbTiO2 박막과 산소 분위기속의 재 증착된 NbTiO2 박막의 성장 변화 패턴에 관한 연구
- 희망과 공감화법이 삶의 만족과 행복에 미치는 영향
- 뇌교육 기반 심성수련이 스트레스 반응 및 대인관계 문제에 미치는 영향
- 대학 이동수업 설계와 활성화에 관한 탐색적 연구
- 다문화가족의 한국생활적응에 대한 교회평생교육 경험 연구
- 소규모 사업장의 기혼여성근로자를 위한 원예활동프로그램 효과
- 영업직 직장인의 스트레스가 이직의도에 미치는 영향 : 긍정심리자본의 매개효과
- 민진 리의 『파친코』에 나타난 재일한인의 장소담론
- 간호대학생의 보건의료 관계 법규 교육에 대한 효과
- 청소년의 문제음주에 영향을 미치는 요인: 2017년 청소년건강행태 온라인조사를 바탕으로
- 마을교육공동체의 현황과 쟁점
- 과대기능(Hyperfunctional) 및 과소기능적(Hypofunctional) 발성을 중심으로 한 가창 지도 개선방법
- 임상실습을 경험한 간호대학생의 자아탄력성, 비판적 사고성향 및 공감피로의 관계
- 도시재생사업의 형태기반코드 적용 가능성 고찰
- 간호학생의 전문직 자아개념과 전공만족도: 학업적 자기효능감의 매개효과
- 노년층의 구강 건강 상태와 심리적 요인의 관련성
- The effect of advertising appeal type on Chinese college students‘ attitude toward advertising message - Exploring the moderation effect of brand type -
- 스포츠산업 지속성을 위한 지역 스포츠단체 윤리경영 제도 정착에 대한 조사 연구
- MOOC 활성화를 위한 블록체인 기술 적용 연구
- 텍스트 마이닝과 언어네트워크 분석을 활용한 국외 ‘교사교육자’ 연구 동향 분석
- 창업의도 요인에 관한 연구 -기업가정신에 따른 개인적 특성의 매개효과와 사회적 지지의 조절효과를 중심으로-
- The Effect of Experiential Values of Tourism on Behavior Intention: Focused on the Mediating Role of Destination Attachment and Destination Trust - An Example of Chinese Travelers to Korea -
- 청소년 활동가의 전문성 구성 요소와 형성 과정에 관한 사례연구
- 액션러닝기반 학업효능감이 성인학습자의 문제해결력, 대인관계, 문제해결태도에 미치는 효과
- 생태체계 요인이 장애인복지관 근로자의 소진에 미치는 영향
- 나눔문화 및 재능기부에 대한 의미 빅 데이터 활용 연구
- 유치원 교육실습생 지도교사의 피드백 내용 분석
- 대학생의 스마트폰 집착 이유 연구
- 태국의 대표 산업과 무역 정책에 관한 고찰
참고문헌
교보eBook 첫 방문을 환영 합니다!
신규가입 혜택 지급이 완료 되었습니다.
바로 사용 가능한 교보e캐시 1,000원 (유효기간 7일)
지금 바로 교보eBook의 다양한 콘텐츠를 이용해 보세요!
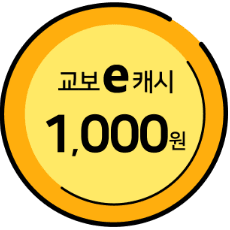