학술논문
혼합 반응 변수를 위한 의사결정나무에서 분류 변수 선택
이용수 16
- 영문명
- Split Variable Selection in Decision Tree for Mixed Responses
- 발행기관
- 한국자료분석학회
- 저자명
- 천재혁(Jae Hyuck Chun) 문호석(Ho Seok Moon) 이석형(Seok Hyung Lee) 조형준(HyungJun Cho)
- 간행물 정보
- 『Journal of The Korean Data Analysis Society (JKDAS)』Vol.15 No.3, 1339~1345쪽, 전체 7쪽
- 주제분류
- 자연과학 > 통계학
- 파일형태
- 발행일자
- 2013.06.30
4,000원
구매일시로부터 72시간 이내에 다운로드 가능합니다.
이 학술논문 정보는 (주)교보문고와 각 발행기관 사이에 저작물 이용 계약이 체결된 것으로, 교보문고를 통해 제공되고 있습니다.
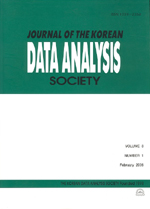
국문 초록
의사결정나무는 데이터마이닝에 쓰이는 대표적인 알고리즘 중 하나로 분류와 해석, 예측의 장점을 가지고 있다. 일변량 반응변수의 의사결정나무와 동일한 유형의 다변량 반응변수의 의사결정나무는 많은 연구가 되고 있다. 하지만 반응변수가 연속형과 범주형이 혼합된 자료 분석을 위한 의사결정나무는 연구가 제대로 이루어지고 있지 않다. 두 개 이상의 반응 변수를 일변량으로 따로 분석하는 것에 비해 동시에 분석할 경우에 더 간략한 모형으로 두 유형의 반응 변수를 함께 설명하고 해석할 수 있다는 장점이 있다. 기존의 알고리즘은 CART 알고리즘을 확장했기 때문에 분류변수 선택 편의와 과도한 계산비용의 문제가 존재한다. 본 논문에서는 혼합 반응변수자료 분석을 위한 의사결정나무에서 분류 변수 선택에 대해 연구하였다. 연구 결과로 전체 탐색알고리즘은 변수의 중요도보다 분할 가능한 수가 많은 변수를 선호하는 경향이 있음을 알 수 있었다. 반면에 잔차 분석 알고리즘은 변수의 분포와 상관없이 변수의 중요도에 따라 분할 변수로 선택하였다. 또한, 전체 탐색 방법에 비해 잔차 분석 방법이 월등히 빠른 계산 속도를 가짐을 확인하였다.
영문 초록
Decision tree is one of the popular data mining tools and has the merits in classification, interpretation, and prediction. A number of algorithms have been developed for univariate data, while few studies exist for the mixed response data. When there are two or more response variables of different types, the variables can be explained and interpreted at the same time by the simpler model from simultaneous analysis rather than separate analyses. The previous tree algorithms for mixed data are based on CART, so they require much computing time and tend to generate selection bias. In the paper, we studied split variable selection for mixed response data. We found that an exhaustive search algorithm had a preference to split variables with more possible splits rather than important variables. In contrast, a residual analysis algorithm accounted for variable importance for split variable selection rather than variable distribution. In addition, a residual analysis algorithm was computationally less expensive than an exhaustive search algorithm.
목차
1. 서론
2. 분할 변수 선택 알고리즘
3. 모의 실험
4. 결론
References
해당간행물 수록 논문
- 보건소 금연클리닉 등록자의 니코틴 의존도 및 흡연관련특성
- 대학생의 비판적 사고력, 메타인지, 문제해결력간의 관계 연구
- Attributably Pure Increment Ratio for Exploration of Meaningful Association Rules
- 연관성 규칙에서의 조정된 상대적 순수 신뢰도의 제안
- A Study of the Impact of Servicescape in the Convention Facilities: Focused on Convention Hotel
- 기억 의도와 작업 기억 부하가 목격자의 얼굴 재인에 미치는 영향
- 관광도시 제주의 도시 브랜드개성이 도시 브랜드태도와 특산품태도에 미치는 영향
- 주택재개발사업의 추가분담금 상승요인 분석에 의한 저감방안
- 마을지도자 교육사업과 농업생산성
- 고용성과가 노후 생활에 끼치는 영향에 관한 연구
- 금융위기 이후 실업자의 고용변동에 관한 연구
- 영과잉 포아송-로그정규회귀모형의 시뮬레이션 최대우도추정
- 여성 초혼율을 사용한 합계출산율 예측에 관한 연구
- Lee-Carter 모형에서 사망률 추정과 보험수리적 현가 분석
- An Objective Bayesian Analysis of the Powered Ratio of Multinomial Proportions
- Analysis of Riskless Short Term Interest Rate GARCH Models with Level Effect
- 수산업 협동조합 신용사업의 효율성과 그 결정요인에 관한 연구
- Learning by Doing and Learning from Others in Contraception
- Proposal for a Concise Method to Explore Reasonable Cutoffs from Clinical Databases by Exploiting Serial Testing
- 패스에 기초한 스포츠 경기력 분석
- 서비스실패 후 불평 토로자와 불평 비토로자의 개성적 차이
- 순서화된 범주형 실험자료에 대한 누적법과 비-누적법
- 여대생의 완벽주의, 신체상, 대인관계가 월경전후기 증상에 미치는 영향
- 중강도 트레이닝이 비활동적인 성인 남성의 인슐린, 성장호르몬, 렙틴 및 주관적 식욕에 미치는 영향
- Modified Tukey-Willams’ Confidence Intervals for Variance of A in One-Factor Components of Variance Model
- 스마트폰 영상을 이용한 관심전환이 학령전기 아동의 정맥주사시 통증과 주사공포에 미치는 효과
- 비열등성 임상시험의 비열등성 한계 설정에 대한 체계적 문헌고찰과 메타분석
- 한국의 국민건강증진모형 구축에 관한 연구
- 내부자의 거래행태에 영향을 미치는 요인
- 이익조정과 터널링
- 카네기분류를 활용한 한국 자연계 연구중심대학 분류 연구
- 양한방 종합병원 환자의 만족도와 충성도에 영향을 미치는 요인
- 학교 특기적성 프로그램을 통한 사회성발달이 예절에 미치는 영향
- 초등학생의 정서인식 및 정서표현의 부족이 공격성 및 또래관계에 미치는 영향
- The Sharia Stock, MGS, and Sukuk GII of Islam Finance
- The Momentum Investment Strategy in the Korean Stock Market
- 협력적 필터링에서 순서 일치도와 응답특성의 관련성
- 혼합 반응 변수를 위한 의사결정나무에서 분류 변수 선택
- 2012년 기업체 장애인고용 실태조사 표본추출 사례 연구
- 글로벌 상품선물 거래정보가 국내 상장지수펀드(ETF) 수익률에 미치는 영향
- 지역 전시박람회 서비스 품질이 만족도 및 재방문 의도에 미치는 영향에 관한 연구
- 지역사회 건강조사자료에 대한 다수준 모형의 활용성에 대한 연구
- 중년 남성의 중년 위기감에 영향을 미치는 요인
- 2008년 금융위기 전후 국내 은행산업의 수익성과 효율성의 관계
- 보건소 자원봉사자 교육 프로그램의 개발 및 인지된 효과 분석
참고문헌
교보eBook 첫 방문을 환영 합니다!
신규가입 혜택 지급이 완료 되었습니다.
바로 사용 가능한 교보e캐시 1,000원 (유효기간 7일)
지금 바로 교보eBook의 다양한 콘텐츠를 이용해 보세요!
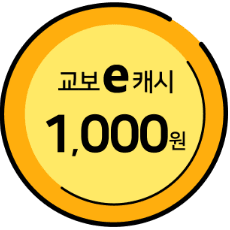