학술논문
딥러닝 기반 시계열 분석 모델의 불확실성 정량화 비교 연구
이용수 58
- 영문명
- A Comparison of Uncertainty Quantification of Deep Learning models for Time Series
- 발행기관
- 한국자료분석학회
- 저자명
- 윤영인(Young-In Yoon) 정혜영(Hye-Young Jeong)
- 간행물 정보
- 『Journal of The Korean Data Analysis Society (JKDAS)』Vol.26 No.1, 163~174쪽, 전체 12쪽
- 주제분류
- 자연과학 > 통계학
- 파일형태
- 발행일자
- 2024.02.29
4,240원
구매일시로부터 72시간 이내에 다운로드 가능합니다.
이 학술논문 정보는 (주)교보문고와 각 발행기관 사이에 저작물 이용 계약이 체결된 것으로, 교보문고를 통해 제공되고 있습니다.
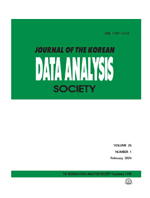
국문 초록
인공지능의 발전으로 머신러닝과 딥러닝 모델이 다양한 산업에서 적용되어 좋은 성능을 보이고 있으며 최근 금융시장에서도 적용되는 사례가 증가하고 있다. 그러나 딥러닝 모델은 예측 결과가 나오게 된 과정과 해석을 파악하기에 어려움이 있다. 이는 결과에 대한 해석이 특히 중요시 되는 금융에 딥러닝 모델을 적용하는데 어려움이 있어 신뢰할 수 있는 모델에 대한 필요성이 대두되고 있다. 신뢰할 수 있는 모델이란 모델에 Dropout과 같은 변화에도 일관된 예측을 보이는 안정적인 모델로 모델의 불확실성을 통해 파악할 수 있다. 본 연구는 딥러닝 모델의 불확실성을 확인하여 신뢰할 수 있는 모델의 기준을 보이고 모델의 불확실성을 통해 이상 탐지하는 모델을 파악하고자 한다. 실험에서 전통적인 통계 모델 ARIMA(Auto Regressive Integrated Moving Average)와 시계열 데이터에 주로 쓰이는 딥러닝 모델인 CNN(Convolutional Neural Network), LSTM(Long Short Term Memory), MLP(Multi-Layer Perceptron), 및 CNN-LSTM 모델을 적용하였고 MC(Monte Carlo) Dropout을 통해 베이지안 관점에서 불확실성을 측정하였다. 실험 결과 다양한 패턴의 시계열 데이터에 대해 통계 모델보다 여러 딥러닝 모델이 성능이 좋음을 확인하였고 성능이 가장 우수하지는 않아도 불확실성이 적어 안정적인 모델이 LSTM 계열임을 확인하였다. 이를 통해 불확실성이 모델의 정확도와 함께 모델 선택 시 고려되어야 할 요소임을 확인하였고 불확실성이 큰 모델이 이상 탐지하므로 CNN 계열의 모델이 적합함을 확인하였다.
영문 초록
With the advancement of artificial intelligence, machine learning, and deep learning, their applications in various industries, particularly finance, have increased. However, interpreting predictions from deep learning models poses challenges, especially in finance where result interpretation is important. This study aims to determine the uncertainty of stable deep learning models, despite changes in the model like dropout, to establish standards for reliable models and identify those detecting anomal data through model uncertainty. In the experiment, the traditional statistical model ARIMA and deep learning models mainly used for time series analysis, CNN, LSTM, MLP, and CNN-LSTM. Uncertainty was measured from a Bayesian perspective using MC Dropout. The experimental results confirmed that deep learning models performed better than statistical models for various patterns of time series data. It was observed that, even if the performance was not the best, LSTM based models exhibited low uncertainty, indicating stability. Consequently, this study highlights the importance of considering uncertainty along with accuracy in model selection. Moreover, it was confirmed that models with higher uncertainty are suitable for anomaly detection, making CNN based models particularly fitting for this purpose.
목차
1. 서론
2. 데이터 분석
3. 모델의 불확실성
4. 통계 모델 ARIMA와의 비교 실험
5. 자료 분석
6. 결론 및 향후연구
Reference
해당간행물 수록 논문
- 예측과정을 이용한 연령별 시차 분포 모형
- LSTM Auto Encoder 이용한 접근 이상 항적 탐지 모형
- 벼, 팥, 들깨, 땅콩 작물 재배 농가 조사를 위한 표본설계
- 프레일티 모형을 이용한 부산광역시 주요 암종별 생존율 비교 분석
- Mitigating Attentional Bias: The Impact of Perceived Social Self-Efficacy in Individuals with MMO Games Addiction Tendency
- 대용량 자료의 이항 분류에서의 충분 차원 축소를 위한 전향적 접근 방법
- 함수형 시공간 기법을 활용한 지표면 온도의 변화점 분석
- 텍스트 데이터와 재정데이터를 이용한 사회정책분야 예산 분석
- 딥러닝 기반 시계열 분석 모델의 불확실성 정량화 비교 연구
- Tobit 모형을 활용한 취업 만족도 결정요인 분석
- 이산확률분포의 표본 분위수 계산 방법에 관한 연구
- 한국과 미국의 대표 경제 학술지에 대한 토픽분석
- 네트워크 텍스트분석을 통한 치매대상자 인간중심돌봄 연구 경향 분석
- 임금근로자의 고용보험 사각지대와 미가입 특성 분석
- 모바일 동영상 광고 편익-비용 인식, 광고에 대한 태도, 모바일 동영상 이용량이 모바일 동영상 광고태도에 미치는 영향
- 한국 주택가격의 동태적 변화 연구
- 골관절염 여성노인의 건강관련 삶의 질 영향요인
- 스포츠 전공 학생-교수 간의 교환관계(LMX)가 학습성과에 미치는 영향
- 역할 과부하와 과업 수행의 관계
- 문해력과 어휘 접속 효율 간의 관련성
- 재개발·재건축 조합원의 조직몰입이 조직성과에 미치는 영향
- 머신러닝과 통계 모형을 이용한 미술품 가격 예측
- Determinants of Managerial Pay: The Relative Contribution of Compensation Predictors
- 투자심리와 현금전환주기 간의 관계
- 증권회사의 고객민원과 기업가치에 관한 연구
- 글로벌 손해보험산업의 성장성과 수익성 및 안정성에 관한 연구
- 노인의 우울 관련 요인
- 기업 소유 스포츠 구단의 비대면 ESG 활동 효과
- 자기통제고갈이 스마트폰 중독자의 스마트폰 사용욕구 증가에 미치는 영향
- 1인가구의 행복에 영향을 미치는 심리사회적 요인
- Journal of The Korean Data Analysis Society (JKDAS) Vol.26 No.1 표지, 목차
참고문헌
관련논문
자연과학 > 통계학분야 BEST
더보기자연과학 > 통계학분야 NEW
- Joint Modeling of Multi-Scale Stock Price using Hierarchical Hidden Markov Models
- Journal of The Korean Data Analysis Society (JKDAS) Vol.26 No.5 Contents
- 머신러닝 분류 모형을 이용한 Netflix 콘텐츠 시청 시간 예측
최근 이용한 논문
교보eBook 첫 방문을 환영 합니다!
신규가입 혜택 지급이 완료 되었습니다.
바로 사용 가능한 교보e캐시 1,000원 (유효기간 7일)
지금 바로 교보eBook의 다양한 콘텐츠를 이용해 보세요!
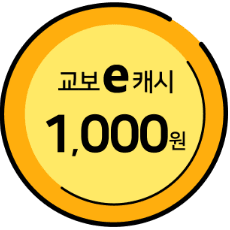