학술논문
대용량 자료의 이항 분류에서의 충분 차원 축소를 위한 전향적 접근 방법
이용수 11
- 영문명
- A Forward Approach for Sufficient Dimension Reduction in Binary Classification for Large-scale Data
- 발행기관
- 한국자료분석학회
- 저자명
- 강종경(Jongkyeong Kang) 박승환(Seunghwan Park) 방성완(Sungwan Bang)
- 간행물 정보
- 『Journal of The Korean Data Analysis Society (JKDAS)』Vol.26 No.1, 79~92쪽, 전체 14쪽
- 주제분류
- 자연과학 > 통계학
- 파일형태
- 발행일자
- 2024.02.29
4,480원
구매일시로부터 72시간 이내에 다운로드 가능합니다.
이 학술논문 정보는 (주)교보문고와 각 발행기관 사이에 저작물 이용 계약이 체결된 것으로, 교보문고를 통해 제공되고 있습니다.
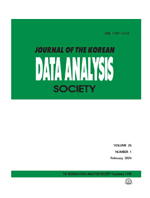
국문 초록
반응변수의 정보를 담고있는 설명변수의 저차원 부분공간인 차원축소공간을 찾는 충분 차원축소에 대한 연구는 주로 역방향 기반의 방법론에 근거하고 있다. 이러한 방법들은 구현이 용이한 장점이 있으나, 일반적으로 선형성 조건이나 상수 분산과 같은 제약 조건등을 필요로 한다. 이러한 한계를 극복하기 위해, 중심부분공간을 전향적으로 추정하는 기법들이 개발되었다. 특히, 재생성 커널 힐베르트 공간을 활용하는 방법들이 주목받았으나, 커널 공간의 특성으로 인해 대용량 자료의 분석에 직접적으로 활용하기에는 제한이 있다. 본 논문에서는 대용량 자료의 이항 분류에서의 충분 차원 축소를 위한 새로운 전향적 접근 방법에 관해 연구하였으며, 분할 정복기법을 활용하여 자료를 부분집합으로 나눈 다음 각 부분집합에서 독립적으로 차원 축소를 수행한 후 이를 종합하여 최종모델을 구축하는 방법을 제안하였다. 부분 자료의 분할 수가 적절히 선택되었을 때, 기존의 방법에 비해 예측 정확성에서 손실이 크지 않으면서도 저장공간 및 계산비용 측면에서 매우 효율적임을 입증하였다. 다양한 모형에서의 모의실험을 통해 다른 역방향기반의 방법들과 비교하여 예측 정확성 측면에서 뛰어남을 보였으며, 나아가 실제 자료의 분석을 통해 제안 방법의 유용성을 확인하였다.
영문 초록
Sufficient dimension reduction, aimed at finding a lower-dimensional subspace in explanatory variables that contains response variable information, typically relies on inverse-based methodologies. These methods are easy to implement but often require linear or constant variance conditions. To address these limitations, techniques for forwardly estimating the central subspace have been developed. In particular, methods utilizing the Reproducing Kernel Hilbert Space have gained attention, but their use in analyzing large datasets is limited due to the characteristics of the kernel space. In this paper, we study a novel forward approach for sufficient dimension reduction in binomial classification of large-scale data. We propose a method that employs a divide-and-conquer technique to split data into subsets, then independently perform dimension reduction on each subset before synthesizing them into a final model. It was shown that when the number of partitions of data was appropriately selected, the loss in prediction accuracy was not significant compared to the existing method, while being efficient in terms of storage space and calculation cost. In addition, simulations in various models showed superior prediction accuracy than other inverse-based techniques. The utility of the proposed method was confirmed through the real data analysis.
목차
1. 서론
2. 이항 분류에서의 충분 차원 축소를 위한 전향적 방법
3. 대용량 자료의 이항분류에서의 충분 차원 축소를 위한 전향적 방법
4. 모의실험
5. 실제 자료 분석
6. 결론 및 제언
References
키워드
해당간행물 수록 논문
- 예측과정을 이용한 연령별 시차 분포 모형
- LSTM Auto Encoder 이용한 접근 이상 항적 탐지 모형
- 벼, 팥, 들깨, 땅콩 작물 재배 농가 조사를 위한 표본설계
- 프레일티 모형을 이용한 부산광역시 주요 암종별 생존율 비교 분석
- Mitigating Attentional Bias: The Impact of Perceived Social Self-Efficacy in Individuals with MMO Games Addiction Tendency
- 대용량 자료의 이항 분류에서의 충분 차원 축소를 위한 전향적 접근 방법
- 함수형 시공간 기법을 활용한 지표면 온도의 변화점 분석
- 텍스트 데이터와 재정데이터를 이용한 사회정책분야 예산 분석
- 딥러닝 기반 시계열 분석 모델의 불확실성 정량화 비교 연구
- Tobit 모형을 활용한 취업 만족도 결정요인 분석
- 이산확률분포의 표본 분위수 계산 방법에 관한 연구
- 한국과 미국의 대표 경제 학술지에 대한 토픽분석
- 네트워크 텍스트분석을 통한 치매대상자 인간중심돌봄 연구 경향 분석
- 임금근로자의 고용보험 사각지대와 미가입 특성 분석
- 모바일 동영상 광고 편익-비용 인식, 광고에 대한 태도, 모바일 동영상 이용량이 모바일 동영상 광고태도에 미치는 영향
- 한국 주택가격의 동태적 변화 연구
- 골관절염 여성노인의 건강관련 삶의 질 영향요인
- 스포츠 전공 학생-교수 간의 교환관계(LMX)가 학습성과에 미치는 영향
- 역할 과부하와 과업 수행의 관계
- 문해력과 어휘 접속 효율 간의 관련성
- 재개발·재건축 조합원의 조직몰입이 조직성과에 미치는 영향
- 머신러닝과 통계 모형을 이용한 미술품 가격 예측
- Determinants of Managerial Pay: The Relative Contribution of Compensation Predictors
- 투자심리와 현금전환주기 간의 관계
- 증권회사의 고객민원과 기업가치에 관한 연구
- 글로벌 손해보험산업의 성장성과 수익성 및 안정성에 관한 연구
- 노인의 우울 관련 요인
- 기업 소유 스포츠 구단의 비대면 ESG 활동 효과
- 자기통제고갈이 스마트폰 중독자의 스마트폰 사용욕구 증가에 미치는 영향
- 1인가구의 행복에 영향을 미치는 심리사회적 요인
- Journal of The Korean Data Analysis Society (JKDAS) Vol.26 No.1 표지, 목차
참고문헌
관련논문
자연과학 > 통계학분야 NEW
- 행사성 사업의 효과 분석 방안
- Journal of The Korean Data Analysis Society (JKDAS) Vol.26 No.2 목차
- OTT 서비스 확산 이후 유료방송 VOD 이용의 결정 요인 분석
최근 이용한 논문
교보eBook 첫 방문을 환영 합니다!
신규가입 혜택 지급이 완료 되었습니다.
바로 사용 가능한 교보e캐시 1,000원 (유효기간 7일)
지금 바로 교보eBook의 다양한 콘텐츠를 이용해 보세요!
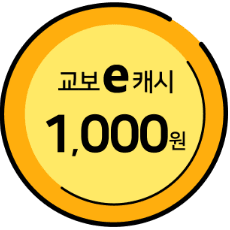