학술논문
XGBoost 앙상블에 의한 서울시 초미세먼지 예측
이용수 265
- 영문명
- The Prediction of PM2.5 in Seoul through XGBoost ensemble
- 발행기관
- 한국자료분석학회
- 저자명
- 김혁(Hyeuk Kim)
- 간행물 정보
- 『Journal of The Korean Data Analysis Society (JKDAS)』Vol.22 No.4, 1661~1671쪽, 전체 11쪽
- 주제분류
- 자연과학 > 통계학
- 파일형태
- 발행일자
- 2020.08.30
4,120원
구매일시로부터 72시간 이내에 다운로드 가능합니다.
이 학술논문 정보는 (주)교보문고와 각 발행기관 사이에 저작물 이용 계약이 체결된 것으로, 교보문고를 통해 제공되고 있습니다.
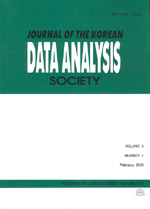
국문 초록
미세먼지는 사람의 건강에 많은 영향을 미치는 물질로서 환경과 건강에 대한 관심이 높아지면서 이와 관련하여 다양한 연구가 이루어지고 있다. 대기환경 분야에서는 전통적으로 수치모형을 이용하여 미세먼지와 관련된 연구를 수행하였으나 기계학습 분야가 발달함에 따라 최근 들어 기계학습을 이용한 연구도 수행하고 있다. 본 연구는 초미세먼지 농도의 예측에 관한 연구로서 기존의 연구들이 대부분 단기(1시간~2일 후) 예측에 그치는데 반해 중기(1주일 후) 예측을 시도하고 있다. 서울지역의 일평균 초미세먼지 농도를 보통(36βm미만)과 나쁨(36βm이상)의 이 단계로 나누어 이를 예측하는 분류모형을 만들었다. 분류모형으로는 XGBoost를 사용하였으며 모형의 성능 극대화를 위하여 각기 다른 평가지표를 기준으로 초모수 최적화(hyperparameter optimization)를 시도한 개별 모형들을 만든 후 앙상블 모형을 구축하였다. 2016년부터 2018년까지 3년의 자료를 이용하여 모형을 훈련시킨 후 2019년 1월부터 6월까지의 서울시 일 평균 미세먼지농도를 예측한 결과 단일 모형보다 본 연구에서 제시한 앙상블 모형의 성능이 좋음을 확인하였다. 또한 미세먼지의 등급이 나쁜 경우보다 보통인 경우가 횔씬 많은 것을 고려하여 범주의 불균형 상태를 보완하는 방법을 적용하였으나 환경물질 자료만 설명변수로 사용할 때는 효과적이나 환경물질과 기상 정보에 관한 변수들을 모두 사용할 때는 오히려 모형의 성능에 악영향을 가져오는 것을 확인하였다.
영문 초록
Fine dust is a substance that greatly affects human health, and as interest in the environment and health increases, various studies have been conducted in this regard. In the field of atmospheric environment, studies related to fine dust have been traditionally performed using numerical models, but the research based on the machine learning has been conducted recently as the field of machine learning has developed. This study is about the prediction of ultrafine dust concentration, and it attempts to predict the middle (after 1 week) prediction while most of the existing studies are only short-term (after 1 hour to 2 days) prediction. A classification model was develeped to predict the average daily ultrafine dust concentration in Seoul by dividing it into two stages: normal (less than 36) and bad (more than 36). XGBoost was used as the classification model and an ensemble model was constructed after creating individual models through the hyperparameter optimization based on different evaluation indicators in order to maximize the performance of the model. The average daily fine dust concentration in Seoul from January to June 2019 was predicted after training the model using data from 2016 to 2018, and the performance of the ensemble model presented in this study was higher than that of a single model. In addition, the method of imbalance problem was applied since the number of cases where the level of fine dust is bad is much less than the number of cases where the level of fine dust is normal. It works effectively when only environmental material data is used as explanatory variables, but it adversely affects the performance of the model when both variables related to environmental materials and weather information are used.
목차
1. 서론
2. 선행연구
3. 연구 방법
4. 모형 적용 결과
5. 결론
References
해당간행물 수록 논문
- 연관성 생성을 위한 수정된 대칭적 불균형 비의 제안
- 컨설턴트의 서번트 리더십이 수진기업의 컨설팅 성과에 미치는 영향
- Stacking Based Ensemble with Instance Selection in Random KNN
- 제2형 당뇨병 환자의 당뇨합병증에 대한 비약물적 중재효과
- The Effect of Population Aging on Stock Prices in Korea
- CEO Pay-performance Sensitivity in KOSPI and KOSDAQ Firms
- XGBoost 앙상블에 의한 서울시 초미세먼지 예측
- 부산시 주거환경의 평가와 정비에 관한 연구
- 지역 일자리사업 예산결정요인과 상대적 효율성 분석
- Gaussian Mixture Model을 이용한 선박 메인 엔진 빅데이터의 이상치 탐지
- 연결재무제표 감사시간 공시와 감사품질에 대한 분석
- 경영자 능력이 감사인의 감사의견 형성에 미치는 영향
- 대응분석을 통한 생명보험과 손해보험 보험상품에 따른 가입채널과 이유
- 공공임대주택의 원활한 사업추진을 위한 공공성 분석
- 모호함의 관용, 불안, 시뮬레이션 간호역량, 학습만족도 간의 관계
- 얼굴 표정에 나타난 정서의 인식과 메타인지적 모니터링
- 중소기업의 주요 경영 지표로 살펴본 제조업 업종 간 수렴성 분석
- 성향점수매칭을 이용한 교양교육연구
- 콜센터 상담사들의 직무스트레스가 조직몰입에 미치는 영향
- 브릭스 외환시장의 효율성 변화
- 여자 프로 배구 경기 중 듀스 후 세트포인트에서의 공격 형태 분석
- 직무열의와 번영의 관계에 대한 직무성과의 매개효과와 일의 의미의 조절된 매개효과 연구
- 비례오즈 로짓모형에 의한 비대면수업 만족도에 미치는 영향 연구
- 국내 은행의 자본적정성과 자금조달비용간의 연관성 연구
- IPA 분석을 통한 중국 관광객의 감천문화마을 관광 활성화 방안에 관한 연구
- 탐색 자극의 시각적 특징이 공간적 배열의 맥락 단서 학습에 미치는 영향
- 비정상성 일반화극단치분포를 이용한 한반도 오존농도 분석
- 기상상태가 초미세먼지농도에 미치는 영향에 대한 분석
- 상속이 기혼남성의 노동공급에 미친 영향
- 2단계 집락 조건부 승법 무관양적속성 모형
참고문헌
관련논문
자연과학 > 통계학분야 NEW
- 행사성 사업의 효과 분석 방안
- Journal of The Korean Data Analysis Society (JKDAS) Vol.26 No.2 목차
- OTT 서비스 확산 이후 유료방송 VOD 이용의 결정 요인 분석
최근 이용한 논문
교보eBook 첫 방문을 환영 합니다!
신규가입 혜택 지급이 완료 되었습니다.
바로 사용 가능한 교보e캐시 1,000원 (유효기간 7일)
지금 바로 교보eBook의 다양한 콘텐츠를 이용해 보세요!
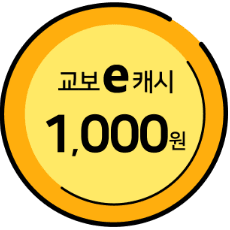