학술논문
glmmLasso 기계학습 기법을 통한 일반고와 특성화고 고등학생의 학교소속감 예측 변수 탐색
이용수 46
- 영문명
- Exploration of Variables to Predict Students’ Sense of Belonging to School via glmmLasso, a Machine Learning Technique: A Comparison of General and Specialized Vocational High Schools
- 발행기관
- 한국교원대학교 교육연구원
- 저자명
- 유진은(Yoo, Jin Eun) 구미령(Koo, Miryeong)
- 간행물 정보
- 『교원교육』제37권 제2호, 467~486쪽, 전체 20쪽
- 주제분류
- 사회과학 > 교육학
- 파일형태
- 발행일자
- 2021.04.30
5,200원
구매일시로부터 72시간 이내에 다운로드 가능합니다.
이 학술논문 정보는 (주)교보문고와 각 발행기관 사이에 저작물 이용 계약이 체결된 것으로, 교보문고를 통해 제공되고 있습니다.

국문 초록
학교소속감이 중도탈락을 막을 수 있는 중요한 변수임에도 불구하고 특히 고등학교 학생에 대한 학교소속감 연구는 그 수가 많지 않다. 아직 충분한 연구가 이루어지지 않은 분야에서 기계학습 기법을 활용하는 탐색적 연구가 학문적 기여를 할 수 있다. 본 연구의 주요 목적은 일반고 및 특성화고 학생들의 학교소속감을 예측하는 중요한 변수를 파악하는 것이다. 이를 위하여 부산교육종단연구 2016 4차년도 데이터의 일반고와 특성화고 학생, 교사, 교장, 학교 변수를 모두 활용하였다. 구체적으로 75개 일반고 1,775명의 824개 변수 자료와 36개 특성화고 739명의 854개 변수 자료를 기계학습 기법으로 분석한 결과, 일반고와 특성화고에서 각각 20개와 21개의 학생, 교사, 학교 관련 변수가 선택되었다. 학교소속감을 개인의 심리적 차원에 초점을 맞추어 분석한 선행연구와 달리, 본 연구는 교사, 교장, 학교 변수까지 모두 모형에 투입함으로써 학교 현장에서의 변화를 꾀하였다. 기계학습 기법 중 벌점회귀모형으로 분류되는 glmmLasso를 활용하여 변수 선택 시 자료의 위계적 구조를 반영한 점 또한 연구 의의라 하겠다. 특히 특성화고 자료는 사례 수보다 변수 수가 더 많은 고차원 자료였으므로 기계학습 기법을 활용하는 것이 필수적이었다. 연구 결과를 토대로 고등학생의 학교소속감을 향상시키기 위하여 필요한 정책적 제언을 제시하고, 후속 연구주제 또한 논하였다.
영문 초록
Although students’ sense of belonging to school is an important indicator of at-risk students including early dropouts, there has not been sufficient research on high school students’ sense of belonging. While confirmatory research requires solid theoretical background, exploratory research particularly via machine learning is unbounded by established theory and thus can make contributions to the existing body of literature. This study aimed at exploring and identifying important variables to predict students’ sense of belonging enrolled in general and specialized vocational high schools. BELS (Busan Educational Longitudinal Study) 2016 fourth wave data (824 variables of 1,775 general high school students and 854 of 739 specialized vocational high school students) were analyzed with glmmLasso, a machine learning technique. In particular, vocational high school data were high-dimensional and thus machine learning was a necessary tool. Specifically, glmmLasso, grouped as penalized regression among machine learning, is known to consider the hierarchical data structure resulting from multistage sampling schemes in variable selection. Among student, teacher, principal, and school variables explored, a total of 20 and 21 variables were selected as important for general and vocational high schools, respectively. Policy suggestions and future research topics were discussed based on the results of the study.
목차
Ⅰ. 서론
Ⅱ. 선행연구
Ⅲ. 연구방법
Ⅳ. 연구 결과
Ⅴ. 논의
키워드
해당간행물 수록 논문
- 영유아교사의 마음챙김이 공감능력에 미치는 영향
- 시각장애 특수학교 고등학생의 여가생활에 대한 경험과 인식
- 중등교사의 ICT 활용 및 인식에 따른 하위집단 분류 및 영향요인 검증
- Concepts of Co-teaching in Secondary English Classrooms: Perspectives of Korean and Native Teachers of English in South Jeolla Province
- 공교육의 관점에서 본 한국 유아교육체제 분석
- 그림기반 진리값 판정과제에 나타난 한국인 EFL 학습자의 영어 재귀사 해석양상
- 교내 온라인 체육 한마당 운영 경험에 관한 협력적 자문화기술지
- 사회적 지지, 교사의 자아존중감 및 교사 소진의 관계
- 교원학습공동체를 통한 가상현실 기반의 음악교육 콘텐츠 개발 및 효용성에 관한 논의
- 상향식 교육과정 평가 모형 개발 및 적용에 관한 협력적 실행 연구
- 초등학생 자신이 지각하는 부모의 자율성 지지, 성장 마인드셋, 그릿에 기초한 군집유형별 학업지연행동에서의 차이
- 범교과 학습 주제에 맞는 경제교육 자료 활성화 방안
- 초등학교 신규교사의 동학년조직 적응 전략에 대한 질적 연구
- 유아의 학교준비도, 학업능력, 사회적 유능감이 초등학교 적응과 학업수행능력에 미치는 영향
- 초학문적 융합에 기초한 도덕적 탐구 수업
- 교사가 지각한 불안정한 성인애착과 대인관계 유능성의 관계에서 대인존재감의 매개효과
- glmmLasso 기계학습 기법을 통한 일반고와 특성화고 고등학생의 학교소속감 예측 변수 탐색
- 수사구조이론을 활용한 고등학생 논증 텍스트 분석 및 지도 방안
- 목생자(牧生者)로서의 교사
- 정책 목표의 추상성과 정책 담론의 모순
- 학생 시선으로 미래교육 바라보기
- 디지털전환 시대 미래 학교교육 시나리오 탐색
- 예비 음악교사의 세계시민성 함양을 위한 음악 수업 사례연구
- 사립유치원교사의 셀프리더십과 행복감이 이직의도에 미치는 영향
- 교직과목 「교육행정」 수업에 관한 질적 사례 연구
- 초등 신규교사 직무역량 강화를 위한 프로그램 설계
- 글 유형에 따른 예비국어교사의 평가 특성 분석
- 학교자치에 관한 교직원 인식 분석 연구
- 판소리 원격수업 콘텐츠 개발의 실제
참고문헌
교보eBook 첫 방문을 환영 합니다!
신규가입 혜택 지급이 완료 되었습니다.
바로 사용 가능한 교보e캐시 1,000원 (유효기간 7일)
지금 바로 교보eBook의 다양한 콘텐츠를 이용해 보세요!
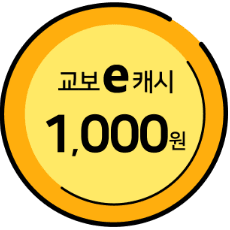