학술논문
Video Vision Transformer를 이용한 벼의 수확량 예측과 Self-attention 시각화
이용수 12
- 영문명
- Rice yield prediction and self-attention visualization using Video Vision Transformer
- 발행기관
- 한국자료분석학회
- 저자명
- 김다현(Dahyun Kim) 나명환(Myung Hwan Na)
- 간행물 정보
- 『Journal of The Korean Data Analysis Society (JKDAS)』Vol.25 No.4, 1249~1259쪽, 전체 11쪽
- 주제분류
- 자연과학 > 통계학
- 파일형태
- 발행일자
- 2023.08.31
4,120원
구매일시로부터 72시간 이내에 다운로드 가능합니다.
이 학술논문 정보는 (주)교보문고와 각 발행기관 사이에 저작물 이용 계약이 체결된 것으로, 교보문고를 통해 제공되고 있습니다.
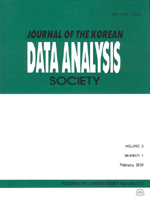
국문 초록
정부와 농민단체는 매년 쌀을 얼마나 생산할 수 있을지 예측하는 문제에 많은 관심을 기울이고 있다. 하지만 해마다 변하는 기상이변과 다양한 병충해와 같은 변동요인들로 인하여 벼의 수확량을 정확하게 예측하는 것을 어렵게 만든다. 본 연구에서는 무인항공기에 탑재된 다중 스펙트럼 센서를 통해 벼의 생육기간 동안 이미지를 여러 번 수집하고, 딥러닝 알고리즘을 이용하여벼 수확량을 예측하였다. 다중 스펙트럼 이미지는 일정 간격을 두고 여러 번 촬영된 일종의 영상 데이터로 볼 수 있으며, 딥러닝 알고리즘 중에서 트랜스포머 구조를 영상 컴퓨터 비전에 적용한 Video Vision Transformer(ViViT) 모델을 사용하여 벼 수확량을 예측하였다. ViViT 모델은입력 영상을 일정한 크기로 분할한 패치(patch)들을 생성하는데, 이 패치의 크기를 다르게 설정하여 모델을 학습한 결과 작은 패치 크기를 사용할수록 예측력이 좋아지는 것으로 나타났다. 또한이미지 처리 분야에서 사용되어온 CNN(Convolutional Neural Network) 구조에서 영상을 입력으로받는 3D CNN 모델과 예측 성능을 비교해본 결과 작은 패치 크기를 사용한 ViViT 모델의 성능이 더 우수한 것으로 나타났다. ViViT 모델의 학습된 가중치 행렬을 heat map으로 시각화한 결과 8월 중후반에 촬영된 이미지가 수확량 예측에 중요하게 나타나 벼를 수확하기 약 두 달 전에수확량 예측이 가능할 것으로 보인다.
영문 초록
The government and farmers' organizations are paying much attention to the problem of predicting how much rice can be produced each year. However, it is difficult to accurately predict the yield of rice due to variable factors such as extreme climate change and various pests and diseases that change every year. In this study, images were collected several times during the growing season of rice through a multi-spectral sensor mounted on an unmanned aerial vehicle, and rice yield was predicted using a deep learning algorithm. Multispectral images can be viewed as a kind of image data taken several times at regular intervals, and rice yield was predicted using the Video Vision Transformer (ViViT) model, which applies the Transformer structure to image computer vision among deep learning algorithms. The ViViT model generates patches by dividing the input image into a certain size, and as a result of learning the model by setting the size of these patches differently, it was found that the smaller the patch size, the better the predictive power. In addition, as a result of comparing prediction performance with a 3D CNN model that receives an image as an input in a CNN (Convolutional Neural Network) structure used in the image processing field, it was found that the ViViT model using a small patch size performed better. As a result of visualizing the weight matrix of the ViViT model as a heat map, images taken in mid- to late August appear to be important in yield prediction, making it possible to predict yield about two months before rice harvest.
목차
1. 서론
2. 분석방법
3. 분석결과
4. 결론
References
키워드
해당간행물 수록 논문
- Journal of The Korean Data Analysis Society (JKDAS) Vol.25 No.4 목차
- 전남 지역 농기계 탑승자 인적피해 교통사고의 유형별 특성 및 저감 대책
- 디퍼러닝 연계 교양교육튜터링 효과성 분석
- K 대학교 핵심역량에 관한 종단 연구
- 간호사의 일-생활 균형이 직무열의 및 직무성과에 미치는 영향과 조직지원인식의 조절된 매개효과
- 간호대학생의 임상 실습 권리 인식 측정 도구 개발
- 랜덤 포레스트 모델을 이용한 성인의 우울증 예측
- 요양병원 간호사의 간호근무환경, 직무스트레스, 이직의도
- 기업가적 태도가 창업에 미치는 영향
- 고령층 소득의 비선형적 지속성 분석
- 은퇴자의 보유자산 조기 고갈위험과 적정 자산배분에 관한 연구
- LDA 모형의 모형평가 및 잠재집단 해석 방법론에 대한 고찰
- 모바일 데이터 요금제 가입 선택의 결정요인 분석
- 평균온도와 자살 사망률의 연관성
- 층화 조건부 이산양적 확률화응답모형
- Performance of Risk Parity Strategy in Developed Markets
- Gender Differences of Smoking Stigma on Gender and Smoking Self-identification in Korean Smokers
- CEO Pay-performance Sensitivities in the ESG Managed Firms
- Video Vision Transformer를 이용한 벼의 수확량 예측과 Self-attention 시각화
- 인구통계학적 지표를 활용한 지방의 소멸위험 예측모형 개발
- 심리학적 한국어 분석 프로그램을 사용한 언어분석 비교
- 코로나19 시대 간호대학생의 비대면 건강관리 봉사활동 효과
- 청년창업기업의 생존율 변화 분석
- 전력 수요 시계열 분석과 장기예측
- 기상 및 토양환경 정보를 이용한 노지 채소의 토양수분 변화 및 예측 모델 개발
- 주택연금 가입자의 노후 대책 충분성에 대한 연구
- 한국 금융시장의 군집행태의 특성과 상호작용에 관한 연구
- 상호출자제한기업집단 소속 여부와 분기 이익조정
참고문헌
관련논문
자연과학 > 통계학분야 NEW
- 행사성 사업의 효과 분석 방안
- Journal of The Korean Data Analysis Society (JKDAS) Vol.26 No.2 목차
- OTT 서비스 확산 이후 유료방송 VOD 이용의 결정 요인 분석
최근 이용한 논문
교보eBook 첫 방문을 환영 합니다!
신규가입 혜택 지급이 완료 되었습니다.
바로 사용 가능한 교보e캐시 1,000원 (유효기간 7일)
지금 바로 교보eBook의 다양한 콘텐츠를 이용해 보세요!
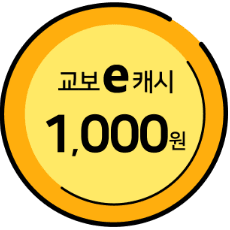