학술논문
금융 분야의 범주 불균형 문제 해결을 위한 성과 최적화 기반의 부스팅 학습
이용수 90
- 영문명
- Performance optimization-based boosting algorithm for resolving class imbalance problems in finance
- 발행기관
- 한국자료분석학회
- 저자명
- 김명종(Myoung-Jong Kim) 안재현(Jae-Hyun Ahn) 김윤후(Yun-Hu Kim)
- 간행물 정보
- 『Journal of The Korean Data Analysis Society (JKDAS)』Vol.25 No.2, 749~762쪽, 전체 14쪽
- 주제분류
- 자연과학 > 통계학
- 파일형태
- 발행일자
- 2023.04.30
4,480원
구매일시로부터 72시간 이내에 다운로드 가능합니다.
이 학술논문 정보는 (주)교보문고와 각 발행기관 사이에 저작물 이용 계약이 체결된 것으로, 교보문고를 통해 제공되고 있습니다.
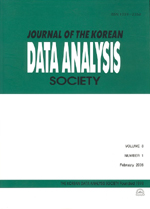
국문 초록
본 연구에서는 금융 분야의 범주 불균형 문제에 적용된 부스팅 계열 알고리즘의 성과 개선을 위하여 성과지표에 대한 직접적인 최적화 기법을 도입한 GMBoost(Geometric Mean-based Boosting) 기법을 제안한다. 본 연구에서는 기업 부실, 카드 연체 및 카드 사기와 같은 대표적인 범주 불균형 금융 문제를 대상으로 GMBoost의 성과를 비교했다. 성과 비교를 위해 벤치마킹 모형으로 부스팅 계열 알고리즘인 AdaBoost, GBM, XGBoost를 활용하였으며, 이들과 GMBoost간의 성과 차이를 비교하였다. 30회의 교차 검증을 통하여 분석한 결과, 첫째, 다수 범주의 특이도에 초점을 맞추어 학습을 진행하여 범주 불균형 문제에서 유의한 성과를 내지 못하는 기존 부스팅 모형과는 달리 GMBoost는 다수 범주의 특이도와 소수 범주의 민감도를 동시에 고려하는 균형적인 학습을 진행함으로써 범주 불균형 문제 해결에 효과적임을 확인하였다. 둘째, 기존 부스팅 모형과 비교하여 GMBoost는 GM 및 AUC 측면에서 우수한 예측 성과를 보여주고 있으며, GM 및 AUC에 대한 t-검정 결과에서도 유의적인 성과 차이를 보여주었다.
영문 초록
In this paper, we propose a GMBoost (Geometric Mean-based Boosting) with a direct performance optimization technique to resolve class imbalance problem in financial field, such as bankruptcy, card insolvency, and card fraud. The conventional boosting models including AdaBoost, GBM, and XGBoost are used as benchmarking models for performance comparison. The main findings of 30 rounds of cross validations are as follows. First, the conventional boosting models largely depend on the specificity of majority, but ignore the sensitivity of minority. On the contrary, GMBoost proceeds with balanced learning that simultaneously considers the specificity and the sensitivity at the same time. Second, GMBoost outperforms the conventional boosting algorithms in terms of GM and AUC, and the results of the t-test for GM and AUC also showed that the performance of GMBoost is significantly different from those of the benchmarking models.
목차
1. 서론
2. 선행연구고찰
3. 학습 알고리즘
4. 연구방법론
5. 연구 결과
6. 결론
References
키워드
해당간행물 수록 논문
- 온라인 학습 기반 LDA 모형을 이용한 이미지 주석 군집화에 대한 연구
- BK21 사업단의 연구 성과물에 대한 서울과 지방대학 비교 분석
- 민영화와 효율성
- COVID-19 환자를 간호하는 중환자실 간호사의 소진 영향요인
- 직무도전성이 학습민첩성을 매개로 주관적 경력성공에 미치는 영향
- 효율적인 물류 운송 배차를 위한 최적 프로세스 연구
- 공간자료에 대한 통계적 위험 경계선 결정 방법에 대한 연구
- 공공도서관의 수요특성에 대한 연구
- CNN 기반 주간 위성 이미지를 활용한 격자 단위 인구추정
- 앙상블 비모수적 변수 선택 방법
- 경영컨설턴트의 셀프리더십이 혁신행동에 미치는 영향
- 면적속도 기반 DTW를 이용한 이탈 항적 탐지
- 시험불안, 사회불안이 간호대학생의 섭식 태도에 미치는 영향
- 민영치매보험의 현물급부에 대한 이용 및 이용 의향에 대한 영향요인 분석
- PIN 추정을 위한 최적모형에 관한 연구
- 증권산업의 NCR제도 개정과 회계정보의 가치관련성
- 연속적 간격법의 타당성
- 비정규직의 일자리 이동성 분석
- 정보전이지수를 이용한 원자재가격과 산업별 주식수익률의 연계성에 대한 Covid-19과 러우전쟁의 영향 분석
- 태풍에 따른 기상요소와 사과의 낙과 피해율 분석
- 코스닥 기업의 ESG 활동이 기업 위험에 미치는 영향
- 시뮬레이션 방법에 의한 보조재생함수의 근사
- 미세먼지의 야외 여가활동 수요에 대한 효과
- 기술분석보고서 발간이 주가급락위험에 미치는 영향에 대한 연구
- 금융 분야의 범주 불균형 문제 해결을 위한 성과 최적화 기반의 부스팅 학습
- 은닉 마르코프 모델을 이용한 적응형 학습경로 생성에 관한 연구
- 한국 가계부채의 결정요인에 대한 실증분석
- 결핵 치료 중 사망에 대한 다수준 분석
- 영구적·일시적 현금흐름 뉴스와 할인율 뉴스에 따른 모멘텀 수익률 분석
- 일반학교와 혁신학교 교사의 마을학습 교육과정에 대한 인식 차이
- Journal of The Korean Data Analysis Society (JKDAS) Vol.25 No.2 목차
참고문헌
교보eBook 첫 방문을 환영 합니다!
신규가입 혜택 지급이 완료 되었습니다.
바로 사용 가능한 교보e캐시 1,000원 (유효기간 7일)
지금 바로 교보eBook의 다양한 콘텐츠를 이용해 보세요!
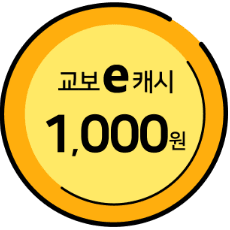