학술논문
XAI Grad-CAM 기반 궤양병 감귤 이미지 분류 CNN 모델의 점검
이용수 59
- 영문명
- An Inspection of CNN Model for Citrus Canker Image Classification Based on XAI: Grad-CAM
- 발행기관
- 한국자료분석학회
- 저자명
- 이동찬(Dongchan Lee) 변상영(Sangyoung Byeon) 김기환(Keewhan Kim)
- 간행물 정보
- 『Journal of The Korean Data Analysis Society (JKDAS)』Vol.24 No.6, 2133~2142쪽, 전체 10쪽
- 주제분류
- 자연과학 > 통계학
- 파일형태
- 발행일자
- 2022.12.30
4,000원
구매일시로부터 72시간 이내에 다운로드 가능합니다.
이 학술논문 정보는 (주)교보문고와 각 발행기관 사이에 저작물 이용 계약이 체결된 것으로, 교보문고를 통해 제공되고 있습니다.
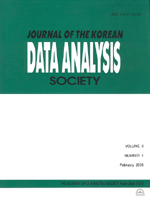
국문 초록
하드웨어의 성능 및 정보처리 기술이 급격히 발전하면서 비정형 데이터의 처리 및 가치 창출에 관한 관심이 증가하고 있다. 이를 위한 다양한 인공지능 아키텍처들이 개발되고 있으며, 모델의 의사결정 분기점이 기하급수적으로 늘어나면서 큰 성능의 개선이 이루어지고 있다. 그러나복잡한 모델 구조는 연구자의 결과 해석 용이성을 저해하는 주요한 원인이 되며, 모델 성능의발전 속도와는 달리 설명 능력에 대해서는 진척이 더딘 실정이다. 설명 가능한 인공지능, 이하XAI(eXplainable Artificial Intelligence)는 위와 같은 문제를 해결하기 위해 등장하였으며, 모델의블랙박스를 이해 가능한 수준으로 분해하여 해석 가능성 및 신뢰도 제고에 도움을 준다. 본 연구에서는 CNN(Convolutional Neural Network) 모델을 사용하여 궤양병 감귤 이미지 분류 문제에접근하였으며, 최종적으로 설계한 모델은 약 97% 수준의 정확도를 보였다. 이후 모델의 신뢰성제고 및 개선 방향 판단을 위해 XAI 기법 중 하나인 Grad-CAM(Gradient-weighted Class Activation Mapping)을 적용하였으며, 이를 통해 구축한 모델이 최종적인 판단을 내리는데 중요한 역할을한 이미지의 특정 영역을 파악하는 과정을 진행하였다. 점검 결과 이미지 외곽의 형태가 객체와구분이 되지 않아 영향을 크게 받는 경우 및 특정 객체의 고유한 형태가 오분류 원인으로 감지되었다.
영문 초록
By the rapid development of hardware performance and information processing technology, interest in processing unstructured data and creating value is increasing. Various types of AI architectures are being developed and as the decision-making junction of the model increased exponentially, the performance is being improved. However, complex model structure is a major cause of hindering researchers' ease of interpret results and unlike the speed of development of model performance, the progress is slow on explanatory ability.
Explainable artificial intelligence (XAI) has emerged to solve this problem and decomposes the model's black box to an understandable level to help improve interpretability and reliability. In this research, we approach the ucler disease citrus image classification problem by using CNN model, and the final model showed approximately 97% accuracy. After that, to improve the reliability of the model and to determine the specific area of the image that played a major role in making the final judgment, Gradient-weighted Class Activation Mapping (Grad-CAM), one of the XAI techniques was applied. As a result of the inspection, it was detected that the shape outside the image wasn't distinguished from the object which was greatly affected. So, the unique shape of a specific object was the main cause of misclassification.
목차
해당간행물 수록 논문
- GLMM을 이용한 지역간 건강도 비교 연구
- ICT 산업 해외투자의 수출입에 대한 영향 분석
- Estimating the Parameters of an Exponentiated Logistic Distribution under Progressive Hybrid Censoring Scheme
- Journal of The Korean Data Analysis Society (JKDAS) Vol.24 No.6 목차
- 인터넷 게임중독 경향군의 게임에 대한 양가감정이 게임갈망에 미치는 영향
- 회복마취 간호사의 그릿, 간호실무숙련도가 간호역량에 미치는 영향
- Fundamentals and machine learning in forecast of won-dollar exchange rate
- 유방암 진단 여성의 삶의 질 영향요인
- 구조방정식모형(SEM)을 활용한 중국 이혼율 영향 요인 분석 연구
- 주가변동성에 대한 가격제한폭의 확대 효과 : 포트폴리오 수익률과 EGARCH 모형을 이용하여
- 국립공원의 특성이 지불의사액에 미치는 영향 분석 : 메타분석을 활용하여
- 연결 RBC제도의 도입과 가용자본의 가치관련성 변화
- Who Adopts Industry 4.0 Technology?
- 표본추출틀에 따른 선거여론조사의 정확성 평가에 대한 연구
- 보리, 밀, 감자, 고구마, 콩, 옥수수, 이탈리안라이글라스 작물재배 농가 조사를 위한 표본설계
- 거래량과 가격정보에 따른 모멘텀과 주식수익률의 관계에 관한 연구
- 웰다잉(well-dying) 교육프로그램이 대학생의 죽음과 웰다잉에 대한 인식에 미치는 효과
- 공동주택의 주거환경 요인과 지각 가치가 주거 만족도에 미치는 영향분석
- 간호사의 일과 삶의 균형과 간호업무성과가 행복지수에 미치는 영향
- 코로나-19 전후의 자산포트폴리오 변동에 관한 고찰
- 매일 흡연 청소년의 특성
- 심리부검의 증거 효용성에 관한 논의 : 토픽모델링을 통한 심리부검의 판례분석
- Effects of External Corporate Governance Mechanism on Financial Distress Risk: Evidence from Chinese Listed Firms
- 모바일, SNS, MCN 미디어 시대에 데이터 어낼리틱스 기반 미디어 시너지 제고 방안: ‘미디어 소비단위’별 연결망 분석을 통한 접근
- 중소·벤처기업 CEO의 온-오프라인 사회적 네트워크가 기업의 동적역량과 회복탄력성에 미치는 영향에 관한 연구
- 대응비교를 통한 가우시안 프로세스 회귀모형의 공분산 모수 선택법
- XAI Grad-CAM 기반 궤양병 감귤 이미지 분류 CNN 모델의 점검
- 미디어 사용 행태 토픽 분석을 통한 추천모델링
- 글꼴의 네모틀 모양 및 삐침이 한글 낱자와 단어에 대한 시각 탐색에 미치는 영향
- 코로나 이전 및 이후 ‘어린이 유튜브’에 관한 기사 내용 트렌드 : 단어 빈도와 토픽 내용 탐색
- A Comparative Study of Nursing Necessity Tools used in Korea and Japan
참고문헌
교보eBook 첫 방문을 환영 합니다!
신규가입 혜택 지급이 완료 되었습니다.
바로 사용 가능한 교보e캐시 1,000원 (유효기간 7일)
지금 바로 교보eBook의 다양한 콘텐츠를 이용해 보세요!
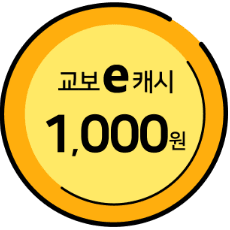