학술논문
예측모형의 오차분산 추정에 관한 모의실험 연구
이용수 57
- 영문명
- A Simulation Study on Prediction Error Variance Estimation
- 발행기관
- 한국자료분석학회
- 저자명
- 이성건(Seong-Keon Lee)
- 간행물 정보
- 『Journal of The Korean Data Analysis Society (JKDAS)』Vol.22 No.6, 2383~2390쪽, 전체 8쪽
- 주제분류
- 자연과학 > 통계학
- 파일형태
- 발행일자
- 2020.12.30
4,000원
구매일시로부터 72시간 이내에 다운로드 가능합니다.
이 학술논문 정보는 (주)교보문고와 각 발행기관 사이에 저작물 이용 계약이 체결된 것으로, 교보문고를 통해 제공되고 있습니다.
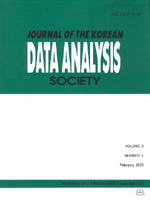
국문 초록
최근 빅데이터에 대한 관심이 증가하여 통계적 분석방법론 뿐만 아니라 기계학습 방법론도 널리 사용되어지고 있다. 통계적 분석방법론은 수리적 배경을 바탕으로 다양한 상황의 오차에 대한 추론과정을 제시하고 있으나, 상대적으로 기계학습 분야에는 상대적으로 강조되고 있지 않다. 기계학습 방법론에는 인공신경망모형(neural network), 의사결정나무모형(decision tree), SVM(support vector machine), 배깅(bagging)을 비롯한 랜덤포레스트(random forest) 등이 널리 활용되고 있다. 이러한 알고리즘에 기반한 방법론들은 기저분포를 비롯한 오차항에 관한 추론에는 관심을 두지 않았던 것이 사실이다. 다행히 최근 이러한 방법론들에 대한 예측오차와 그에 대한 분산추정에 관한 연구가 활발하게 이루어지고 있다. 본 연구는 오차분산 추정을 위하여 OOB(out of bag) 방법을 이용한 평균제곱예측오차(MSPE, mean squared prediction error)를 살펴보고 편의(bias)를 확인 하였다. 이러한 편의를 보정하기 위하여 붓스트랩을 이용한 추정방법을 제시하고 이를 설명변수들의 다양한 공분산 구조와 함수에 대한 모의실험을 통해 효율성을 비교하였다. 기계학습방법론으로는 랜덤포레스트를 이용하였으며 분석 결과 제안한 편의 보정 방법은 복잡한 함수보다는 다항함수를 비롯한 비교적 단순한 함수에서 더 효율적임을 확인하였다.
영문 초록
Recently, as interest in big data analysis has increased, not only statistical analysis methodology but also machine learning has been widely used. Statistical analysis methodology suggests processes based on a mathematical background, but it can be said that relatively less emphasis is placed on the field of machine learning. Machine learning methodologies include a neural network, a decision tree, a SVM, and a random forest including bagging. It is true that methodologies based on these algorithms did not pay attention to inferences about the prediction error terms including the distribution assumptions. Recently, studies on prediction error and variance estimation for these methodologies have been actively conducted. This study examined the mean squared prediction error (MSPE) using the out of bag (OOB) method and bias. To adjust the bias, an estimation method using bootstrap was proposed and the efficiency was compared through simulation. Random forest was used as the machine learning methodology, and as a result of analysis, the proposed bias correction method was more efficient in simple polynomial models than in complex models.
목차
1. 서론
2. 랜덤포레스트
3. 분산 오차 추정량
4. 모의실험
5. 결론
References
키워드
해당간행물 수록 논문
- 남북 관계 뉴스 제목 텍스트 마이닝 분석
- 지역호기심에 영향을 미치는 요인에 관한 연구
- 중국 교통수단의 발전과 지역 경제성장
- 대표이사의 주식소유비중과 주가급락위험
- 손해보험회사의 재보험전략과 기업가치
- 심층신경망을 활용한 비모수적 소프트웨어 신뢰성 모형과 NHPP 소프트웨어 신뢰성 성장 모형 비교
- 예측모형의 오차분산 추정에 관한 모의실험 연구
- 20면체계획과 12면체계획의 통계적 성질에 관한 연구
- Dynamic Spillover across Uncertainty, Financial and Commodity Markets
- 주식수익률 변동성의 비대칭적 반응에 관한 국제적 비교연구
- 1인 창조기업의 기술사업역량이 생존에 미치는 영향에 관한 연구
- Fault Detection of Railway Point Machines Using Electrical Signals
- 중년 및 고령운전자의 지속적 횡적추적과 시각도에 따른 자극선택 수행 차이
- Typologies of Serial Arson: Explorative Study based on Latent Class Analysis
- 국내 OTT 서비스 산업 현황 및 수요 분석
- 머신러닝기법을 이용한 심사위원 자동추천 알고리즘 개발
- 은행의 자금조달 충격과 대출자산 재구성간의 관련성
- WTP 함수에 기반한 Spike 모형에 대한 연구
- 재무적 특성이 기업성장률에 미치는 영향
- 인공신경망을 이용한 실적 공시 후 주가 예측
- 교양강좌로서 데이터 리터러시 가능성 탐색
- 메타분석적 구조방정식모형을 이용한 중년 여성의 우울과 관련 요인 간의 구조적 관계
- 대학생의 베이커리카페 선택속성이 고객만족 및 SNS구전의도에 미치는 영향
- LDA와 Word2vec 방법론을 이용한 의정부시 SNS 데이터의 토픽 모델링 및 시각화
- 간호대학생의 성평등의식, 진로정체감, 의사소통능력이 대학생활적응에 미치는 영향
- A Study on the Medical and Healthcare Consciousness : Centred around 2019 Gyeongsangnam-Do Social Survey
- The Effect of Macroeconomic Fundamentals and Demographic Change on Housing Price
- 간호사 보수교육 실시기관의 교육운영 현황 및 프로그램 질 평가
- 간호대학생의 간호전문직관, 임상실습 스트레스가 진로정체감에 미치는 영향
- CNN을 활용한 CTR 예측 시각화 분석
- COVID-19 팬데믹과 온라인 개학
- 한국에서 알츠하이머 질환 사망의 사회경제적 불평등
- 중·고령자의 일자리 이동에 관한 연구
- 감성변수들을 포함한 지역별 반려견의 입양성공모형
- 오이의 엽록소 형광 분석에 의한 광합성능 지표화 연구
- Prophet 모형을 이용한 마늘 가격의 장기 예측 및 트렌드 분석
참고문헌
관련논문
자연과학 > 통계학분야 NEW
- 행사성 사업의 효과 분석 방안
- Journal of The Korean Data Analysis Society (JKDAS) Vol.26 No.2 목차
- OTT 서비스 확산 이후 유료방송 VOD 이용의 결정 요인 분석
최근 이용한 논문
교보eBook 첫 방문을 환영 합니다!
신규가입 혜택 지급이 완료 되었습니다.
바로 사용 가능한 교보e캐시 1,000원 (유효기간 7일)
지금 바로 교보eBook의 다양한 콘텐츠를 이용해 보세요!
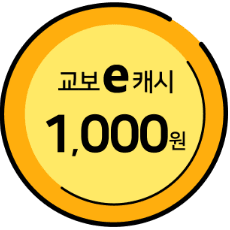