- 영문명
- Visualization Analysis on CNN Based CTR Prediction
- 발행기관
- 한국자료분석학회
- 저자명
- 김태석(Tae-Suk Kim)
- 간행물 정보
- 『Journal of The Korean Data Analysis Society (JKDAS)』Vol.22 No.6, 2603~2614쪽, 전체 12쪽
- 주제분류
- 자연과학 > 통계학
- 파일형태
- 발행일자
- 2020.12.30
4,240원
구매일시로부터 72시간 이내에 다운로드 가능합니다.
이 학술논문 정보는 (주)교보문고와 각 발행기관 사이에 저작물 이용 계약이 체결된 것으로, 교보문고를 통해 제공되고 있습니다.
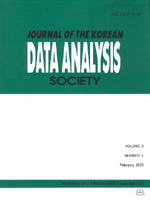
국문 초록
클릭률(click through rate, CTR) 예측은 사용자에게 노출된 광고가 클릭될 확률을 추정하는 것으로 온라인 광고 수익 극대화를 위한 광고 노출 전략 결정에 중요한 역할을 담당한다. CTR 예측의 핵심은 데이터 필드(특징) 간 상호작용을 효과적으로 모델링하는 것인데 최근 시·공간적 연계성을 갖는 데이터에 있어 탁월한 성능을 발휘하고 있는 심층신경망(deep neural network)을 모델링에 활용한 연구가 활발히 진행되고 있다. 본 연구는 CTR 예측을 위해 이미지·음성 인식 분야에서 뛰어난 성능을 보여주고 있는 신경망 구조인 Convolutional Neural Network(CNN)의 활용가능성에 관한 기초적인 연구 결과를 제시한다. 먼저 이미지·음성 데이터와 구별되는 CTR 데이터의 특성과 이로 인한 CNN 적용 시 발생할 수 있는 성능상의 한계점을 도출한다. CNN의 하이퍼 파라미터인 임베딩과 풀링에 관한 비교 설정 실험 결과를 시각적으로 해석하여 CTR 데이터에 대한 하이퍼 파라미터의 영향력을 분석한다. 실험 결과에 따르면, CTR 데이터에 있어 임베딩과 풀링은 유기적으로 성능에 영향을 미치므로 데이터 특성에 맞게 둘 간의 최적 조합을 선택하는 것이 CNN 성능 향상에 매우 중요한 요소가 됨을 확인하였다.
영문 초록
The click through rate(CTR) prediction estimates the probability that an advertisement exposed to a user is clicked, and plays an important role in determining an advertisement exposure strategy for maximizing online advertisement revenue. The key to predicting CTR is to effectively model the interactions between data fields(features). Recently, researches using deep neural network(DNN), which exhibits excellent performance in data with temporal and spatial correlation, are actively conducted for modeling. This study presents basic research results on the possibility of using a convolutional neural network(CNN), a neural network structure that shows the best performance in the image and speech recognition field, for CTR prediction. First, we derive the characteristics of CTR data that are distinguished from image and voice data and the performance limitations that can occur when regular CNN is applied. Then, we visually analyze the experiment results of the comparison setup for CNN hyperparameters(embedding and pooling) to understand the imapct of hyperparameters on CTR data. According to the experimental results, since embedding and pooling organically affect the performance of CTR data, it was confirmed that selecting the optimal combination between the two based on the data characteristics is a very important factor in improving CNN performance.
목차
1. 서론
2. CTR 데이터 특성과 CNN 적용의 한계
3. 시각화 기법을 활용한 성능 분석
4. 결론
Reference
해당간행물 수록 논문
- 지역호기심에 영향을 미치는 요인에 관한 연구
- 재무적 특성이 기업성장률에 미치는 영향
- 중국 교통수단의 발전과 지역 경제성장
- 교양강좌로서 데이터 리터러시 가능성 탐색
- 메타분석적 구조방정식모형을 이용한 중년 여성의 우울과 관련 요인 간의 구조적 관계
- 손해보험회사의 재보험전략과 기업가치
- 대학생의 베이커리카페 선택속성이 고객만족 및 SNS구전의도에 미치는 영향
- 심층신경망을 활용한 비모수적 소프트웨어 신뢰성 모형과 NHPP 소프트웨어 신뢰성 성장 모형 비교
- 예측모형의 오차분산 추정에 관한 모의실험 연구
- 20면체계획과 12면체계획의 통계적 성질에 관한 연구
- 간호대학생의 성평등의식, 진로정체감, 의사소통능력이 대학생활적응에 미치는 영향
- Dynamic Spillover across Uncertainty, Financial and Commodity Markets
- A Study on the Medical and Healthcare Consciousness : Centred around 2019 Gyeongsangnam-Do Social Survey
- 1인 창조기업의 기술사업역량이 생존에 미치는 영향에 관한 연구
- The Effect of Macroeconomic Fundamentals and Demographic Change on Housing Price
- Fault Detection of Railway Point Machines Using Electrical Signals
- 중년 및 고령운전자의 지속적 횡적추적과 시각도에 따른 자극선택 수행 차이
- Typologies of Serial Arson: Explorative Study based on Latent Class Analysis
- 머신러닝기법을 이용한 심사위원 자동추천 알고리즘 개발
- 간호사 보수교육 실시기관의 교육운영 현황 및 프로그램 질 평가
- 은행의 자금조달 충격과 대출자산 재구성간의 관련성
- 간호대학생의 간호전문직관, 임상실습 스트레스가 진로정체감에 미치는 영향
- CNN을 활용한 CTR 예측 시각화 분석
- WTP 함수에 기반한 Spike 모형에 대한 연구
- 한국에서 알츠하이머 질환 사망의 사회경제적 불평등
- 중·고령자의 일자리 이동에 관한 연구
- 감성변수들을 포함한 지역별 반려견의 입양성공모형
- 오이의 엽록소 형광 분석에 의한 광합성능 지표화 연구
- Prophet 모형을 이용한 마늘 가격의 장기 예측 및 트렌드 분석
- 인공신경망을 이용한 실적 공시 후 주가 예측
- 남북 관계 뉴스 제목 텍스트 마이닝 분석
- 대표이사의 주식소유비중과 주가급락위험
- 주식수익률 변동성의 비대칭적 반응에 관한 국제적 비교연구
- 국내 OTT 서비스 산업 현황 및 수요 분석
- LDA와 Word2vec 방법론을 이용한 의정부시 SNS 데이터의 토픽 모델링 및 시각화
- COVID-19 팬데믹과 온라인 개학