학술논문
핵심어 추출 및 데이터 증강기법을 이용한 텍스트 분류 모델 성능 개선
이용수 122
- 영문명
- Improving the performance of text classification models using keyword extraction and data augmentation techniques
- 발행기관
- 한국자료분석학회
- 저자명
- 이강철(Kangchul Lee) 안정용(Jeongyong Ahn)
- 간행물 정보
- 『Journal of The Korean Data Analysis Society (JKDAS)』Vol.24 No.5, 1719~1731쪽, 전체 13쪽
- 주제분류
- 자연과학 > 통계학
- 파일형태
- 발행일자
- 2022.10.31
4,360원
구매일시로부터 72시간 이내에 다운로드 가능합니다.
이 학술논문 정보는 (주)교보문고와 각 발행기관 사이에 저작물 이용 계약이 체결된 것으로, 교보문고를 통해 제공되고 있습니다.
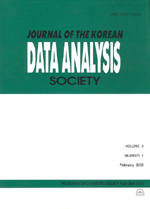
국문 초록
토픽 모델링은 문서에 잠재되어 있는 토픽을 발견하고 분류하는 기법으로 각 문서의 핵심 토픽과 토픽들이 가지고 있는 특성을 파악하는데 유용하다. 그러나 동일한 단어가 여러 토픽에서 높은 가중치를 가지는 경우, 토픽 간 변별력이 있는 핵심어 추출이 어렵다는 문제점을 가지고 있다. 또한, 이 기법은 핵심어와 의미적 유사성이 있으나 핵심어로 채택되지 못한 단어들이 존재하는 경우 정보의 누락이 발생하며, 데이터의 크기와 질에 따라 분류 성능이 달라진다는 단점을 가지고 있다. 이러한 문제점을 개선하기 위하여 본 연구에서는 핵심어를 추출할 때 연관성 척도(relevance)와 워드 임베딩(word embedding) 기법을 적용하는 방법을 제안한다. 또한, 데이터 분류성능을 개선하기 위해 EDA(easy data augmentation) 기법을 이용하여 데이터를 증강한 후 KoBERT 모델을 적용한다. 데이터 분석 결과, 토픽 간 변별력 있는 핵심어를 추출하여 해당 토픽의 구체적인 내용을 파악할 수 있었다. 또한, 데이터 증강기법을 적용한 경우 94% 정확한 분류 결과를 얻어 데이터 증강기법을 적용하지 않은 경우에 비해 9% 정도 개선된 결과를 얻을 수 있었다.
영문 초록
Topic modeling aims to identify and categorize topics latent in documents, and is useful for exploring core topics of each document and the characteristics of the topics. However, a problem with interpreting topics this technique is that common terms often appear near the top of multiple topics, making it hard to extract keywords identifying the topics. Another weakness is that this technique can lead to loss of information when synonyms are excluded from keywords, and high performance often depends on the size and quality of data. To improve these problems, we propose a method that utilizes relevance and word embedding techniques for extracting keywords. In addition, we use the EDA(easy data augmentation) techniques to increase the size of the data, and then apply the KoBERT model for boosting performance on text classification tasks. As a result of data analysis, it was possible to grasp the specific characteristics of the topics based on the discriminating keywords. The results also showed that using the augmented data sets, the text classifier model has higher accuracy than the original data sets with a score of 0.94 and 0.85, respectively.
목차
1. 서론
2. 관련연구
3. 연구 방법 및 분석 결과
4. 결론 및 향후 연구과제
References
키워드
해당간행물 수록 논문
- 소득수준과 이념성향 관계에서 성장 및 분배의 가치선호가 미치는 영향
- 고령화연구패널조사 자료를 이용한 노인 인지기능의 영향요인
- 구글 트렌드를 이용한 2022년 대통령 선거의 지역별 관심도와 네트워크 분석
- Trading costs, fat-tailed liquidity risk, and expected returns
- 토픽 모델링과 로지스틱 회귀분석을 활용한 한국 사회의 혐오 담론 탐구 및 정책 결정 과정과의 상관성 분석
- Visual Attentional Pattern of the Eyewitness in a Mock Crime with Weapon Presence
- 농림어업숙련종사자의 만성질환 사망원인 특성
- 민영건강보험의 현물급부에 대한 소비자 이용의 결정요인 분석
- 주택 매매가격과 전세가격 간의 비대칭적 연관관계
- 학생만족도에 대한 교육품질 속성의 비대칭·비선형 영향 연구
- 전ㆍ후기 노인의 손상특성이 NISS에 미치는 영향요인
- Journal of The Korean Data Analysis Society (JKDAS) Vol.24 No.5 목차
- 성별·연령대별 특성에 따른 위험자산 선택요인 분석
- 위기 하의 호텔업의 기업실패와 기업부실위험 예측에 관한 연구
- 재난지원금 지급에 대한 일별 소비 반응 분석
- 이항 회귀모형의 연결함수 오지정 문제에서 확률화된 분위수 잔차 사용에 대한 연구
- 우리나라 초등학생의 그릿(Grit)수준이 인지, 정서, 사회 발달에 미치는 영향
- 온라인 임상실습 교육이 간호대학생의 학습에 미치는 효과
- 간호대학생의 진로준비행동 영향요인 분석
- 함수형 공간 자료를 위한 추정 및 예측모형과 응용
- 경부고속도로의 경제적 효과
- 가치공동창출 고객행동이 고객경험을 매개로 고객충성도에 미치는 영향
- 핵심어 추출 및 데이터 증강기법을 이용한 텍스트 분류 모델 성능 개선
- The effect of governance quality on tax avoidance: Evidence from Mongolia
- Applications of the Conway-Maxwell-Poisson Hidden Markov models for analyzing traffic accident
- 생육시기 기상을 활용한 배추 가격 예측
- 토양 내 오염물질 농도 예측을 위한 베이지안 벌점 스플라인
- 사내 커뮤니케이션 캠페인의 선행요인과 조직신뢰 및 변화몰입 간 관계
- 상사무례가 직무열의 및 반생산적 과업행동에 미치는 영향
- 미취학 자녀를 둔 간호사의 양육 관련 심리적 요인과 이직 의도의 관계
- 수용자 자살에 대한 다각적 분석
- Fine Dust is Coming Again: The Effect of Air Pollution on Health Using Seasonal Weather Patterns
참고문헌
관련논문
자연과학 > 통계학분야 BEST
더보기자연과학 > 통계학분야 NEW
- Journal of The Korean Data Analysis Society (JKDAS) Vol.27 No.1 Contents
- A Research on ESG Commitment, Governance, and Firm Value
- 영상 콘텐츠 추천시스템을 위한 AWSGLD 알고리즘
최근 이용한 논문
교보eBook 첫 방문을 환영 합니다!
신규가입 혜택 지급이 완료 되었습니다.
바로 사용 가능한 교보e캐시 1,000원 (유효기간 7일)
지금 바로 교보eBook의 다양한 콘텐츠를 이용해 보세요!
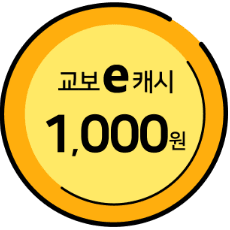