학술논문
ODIM: a method to identify inliers via inlier-memorization effect of deep generative models
이용수 6
- 영문명
- 발행기관
- 한국자료분석학회
- 저자명
- Dongha Kim Jaesung Hwang Yongdai Kim
- 간행물 정보
- 『한국자료분석학회 학술대회자료집』2022년 하계학술대회 발표집, 127~129쪽, 전체 3쪽
- 주제분류
- 자연과학 > 통계학
- 파일형태
- 발행일자
- 2022.07.07
4,000원
구매일시로부터 72시간 이내에 다운로드 가능합니다.
이 학술논문 정보는 (주)교보문고와 각 발행기관 사이에 저작물 이용 계약이 체결된 것으로, 교보문고를 통해 제공되고 있습니다.
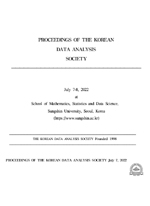
국문 초록
영문 초록
Identifying whether a given sample is an outlier or not is a significant issue in various real-world domains. Many trials have developed outlier detection methods, but they mainly presumed no outliers in the training data set. This study considers a more general situation where training data contains some outliers, and any information about inliers and outliers is not given. We propose a powerful and efficient learning framework to identify inliers in a training data set using deep neural networks. We start with a new observation, called the inlier-memorization effect, that when we train a deep generative model with data contaminated with outliers, the model first memorizes inliers before outliers. Exploiting this finding, we develop a new method called Outlier Detection via the Inlier-Memorization effect(ODIM). The ODIM only requires a few updates; thus, it is fast and efficient. We also provide a data-adaptive strategy to find the optimal number of updates, which makes the ODIM applied to real domains at ease. We empirically demonstrate that our method can refine inliers successfully in both tabular and image data sets.
목차
1. Introduction
References
해당간행물 수록 논문
- 한국 노인의 NISS 손상지수에 영향을 미치는 요인
- 사회안전지수로 살펴본 사회안전 유형화와 다층모형의 적용
- 양념채소(대파) 재배농가의 재배규모에 따른 경영성과 순환구조 분석
- 재정데이터 내의 이상치 탐지를 위한 비지도 방법의 성능 비교
- 코로나 19로 인한 일상생활변화가 한국 청소년의 범불안장애에 미치는 영향
- 지역사회의 안전수준을 측정하기 위한 사회안전지수의 개발
- 학원 교습시간 규제와 학교 성적분포
- 식민지기 교회와 인적자본
- 2022년 하계학술대회 발표집 목차
- 외국인 직접투자(FDI)와 경제자유도가 경제성장에 미치는 영향
- 패널자료에서의 은닉마르코프모형을 이용한 사례 분석 연구
- 부모의 정년이 자녀의 혼인에 미치는 영향
- 간호대학생의 심리적 독립, 자아존중감에 따른 대인관계 유능성 연구
- 만성통증 노인환자의 주관적 건강상태에 따른 건강행위간 네트워크 분석
- 토양 내 오염물질 농도 예측을 위한 베이지안 벌점 스플라인
- 간호대학생의 자기인식, 타인인식과 의사소통능력 간의 관계
- 한국 성인에서 체질량지수와 허리둘레를 기준으로 분류한 비만 유형별 건강행위 특성 분석
- 음원 스트리밍 플랫폼 선택속성의 중요도 분석에 관한 연구
- 현금흐름 뉴스와 할인율 뉴스 상황에 따른 모멘텀 수익성에 관한 연구
- 조건부 Quatember 확률화응답모형
- Decomposition of the Dividend Model: from the Perspective of ESG and Firm Characteristics
- 리만 좌표하강 알고리즘을 이용한 조각 측지선 적합
- Dynamic Portfolio Using Association Rules
- 한국 배출권거래시장 수요측면의 가격결정요인분석
- Distribution Contrast for Disentangled Fair Representation
- Application of the Conway-Maxwell-Poisson Hidden Markov models for analyzing traffic accident
- PLS 의사결정나무 분석
- 유방암 진단 여성의 삶의 질 영향요인
- Neural Temporal-Filter Network
- 중년 여성의 갱년기 자각증상, 스트레스, 건강증진행위 및 삶의 질
- 간호사 소진 경감 중재 프로그램의 효과: 체계적 문헌고찰 및 메타분석
- 간호대학생의 비판적 사고성향, 자기주도적 학습능력과 학업적 자기효능감
- SNS의 프라이버시 염려에 관한 연구
- ODIM: a method to identify inliers via inlier-memorization effect of deep generative models
- Bayesian Approach for Eliminating User Influence and Item Popularity Biases in User-Item Bipartite Networks
- SVD 기반 데이터 증강과 기계학습을 통한 웨이퍼 결함 패턴 분류
- 같은 정보를 <그림> VS <표>로 제시하면 소비자의 인지능력이 달라지는가?
- 분리된 공정 표현 학습을 위한 분포 대조
- 제주 지역 사례를 통한 재난지원금 지급의 경제적 영향 분석
- Ensemble Variable Selection Using Genetic Algorithm
- 유튜브 악플 탐지를 위한 기계학습
- 딥러닝을 이용한 서술형 강의 평가 분류 연구
- 중소병원 간호사의 감성지능과 동료 간 돌봄-배려행위가 조직몰입에 미치는 영향
- 실물옵션(Real Option) 평가방법을 활용한 올슨모형(Ohlson Model) 실증분석
- 제한폭 확대 영향 연구
- Multinationality, Portfolio Coordination, and Downside Risk
- 간호대학생의 감성지능수준, 스트레스, 스트레스 대처능력 간 관계
- CNN 및 Grad-CAM 기반의 궤양병 감귤 이미지 분류모델 구축 및 점검
- EXoN: EXplainable encoder Network
- 응급실에 내원한 3대 중증응급환자 양상변화
- 위기 하의 호텔업의 기업실패와 기업부실위험 예측에 관한 연구
- 코로나19로 인한 일상 및 행동 변화 분석
- 외식업 O2O 배달 앱 품질이 소비자의 이용의도에 미치는 영향
- 임상간호사의 일과 삶의 균형, 간호업무성과가 행복지수에 미치는 영향
- Self-supervised classification for functional data
- Forecasting Tail Risk of Time Series with Weighted Scoring Rules
- Predicting the Effect of the Fear and Greed Index for Digital Assets and Stocks on the Trends of Apartment Sales by Artificial Neural Network
- 강설이 지역별 교통사고에 미치는 영향
- CNN 기반 위성 이미지를 활용한 격자단위 인구추정
- Different Perspectives on Learning Statistics
- 간호대학생의 학년별 학업소진과 스트레스 대처능력 및 자기효능감 비교
참고문헌
관련논문
자연과학 > 통계학분야 NEW
- 행사성 사업의 효과 분석 방안
- Journal of The Korean Data Analysis Society (JKDAS) Vol.26 No.2 목차
- OTT 서비스 확산 이후 유료방송 VOD 이용의 결정 요인 분석
최근 이용한 논문
교보eBook 첫 방문을 환영 합니다!
신규가입 혜택 지급이 완료 되었습니다.
바로 사용 가능한 교보e캐시 1,000원 (유효기간 7일)
지금 바로 교보eBook의 다양한 콘텐츠를 이용해 보세요!
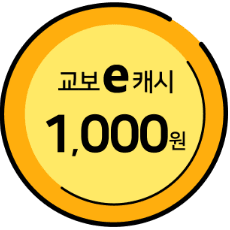