학술논문
자율운항선박 지원을 위한 실시간 관측 기반의 해양환경 인공지능 예측 기술 검증
이용수 0
- 영문명
- Verification of Artificial Intelligence Prediction Technology of Marine Environment Based on Real-Time Observation for Maritime Autonomous Surface Ship
- 발행기관
- 한국항해항만학회
- 저자명
- 엄대용(Dae-Yong Eom) 서의성(Eui-Sung Seo) 전형섭(Hyung-Seop Jeon) 이방희(Bang-Hee Lee)
- 간행물 정보
- 『한국항해항만학회지』제48권 제6호, 554~565쪽, 전체 12쪽
- 주제분류
- 공학 > 해양공학
- 파일형태
- 발행일자
- 2024.12.31
4,240원
구매일시로부터 72시간 이내에 다운로드 가능합니다.
이 학술논문 정보는 (주)교보문고와 각 발행기관 사이에 저작물 이용 계약이 체결된 것으로, 교보문고를 통해 제공되고 있습니다.
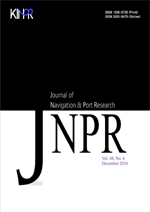
국문 초록
자율운항선박 등 스마트 선박에서 항로상의 해양환경 상태를 관측·예측하는 과정은 필수 요소이며, 선박의 관측자료만을 이용하여 의사결정이 가능하도록 해양환경 정보를 생산하는 기술이 필요하다. 제약된 선박 장비 상황 속에서 실시간 수집 가능한 해양환경정보는 파랑, 해상풍이 일반적이다. 본 연구에서는 4종의 인공지능 알고리즘(ANN, RNN, Conv-LSTM, GAN)과 2개의 학습 구성 방안(초단기-비연속, 장기-연속)을 접목하여 항로상의 시계열 관측 정보만으로 다음 시간대의 해양환경 예측 정보를 생산할 수 있는 기술을 개발하였다. 개발된 알고리즘은 기상청에서 운용 중인 ‘기상1호’의 실 선박 관측자료 1년 치를 활용하여 계절별로 비교·분석하였다. 초단기-비연속 학습 구성에서는 지도 학습모델 중 RNN이 가장 좋은 성능을 보였고 비지도 학습 모델은 낮은 성능을 보인 반면, 장기-연속 학습 구성에서는 모든 모델이 모든 계절에서 높은 정확도를 보였다. 학습 구성 방안에 따른 알고리즘별 예측 정확도 검증에서는 초단기-비연속에 비해 장기-연속 학습 구성이 파랑, 해상풍 모두에서 계절별 예측 정확도가 우수하였다. 본 연구를 통해 자율운항선박 내 데이터 기반 해양환경 예측 정보를 제공하기 위한 기술의 유의미성과 가능성을 확인하였으며 향후 범용수로데이터모델(S-100)과의 연계, 선박 운동성 및 항로 추정 등과 연계되어 보다 실질적인 의사결정 정보 생산에 기여할 것으로 기대된다.
영문 초록
For smart ships, such as autonomous vessels, technology that can predict and observe the state of the marine environment along the route in real-time is essential. This requires observational data from ships. The capability to generate marine environmental information for effective decision-making is crucial. Typically, the information that can be collected in real-time under limited equipment conditions on ships includes wave and offshore wind data. In this study, we developed an algorithm that produces marine environment prediction information for subsequent time periods based solely on time series observation data from the route. This prediction information integrates four types of artificial intelligence algorithms (ANN, RNN, Conv-LSTM, and GAN) and two learning structure methods (ultra-short-term - discontinuous and long-term - continuous). The algorithm was compared and analyzed by season using one year of actual ship observation data from 'Meteorological No. 1,' operated by the Korea Meteorological Administration. Among the supervised learning models, RNN demonstrated the best performance in the ultra-short-term and discontinuous learning structure. While unsupervised learning models exhibited lower performance, all models achieved high accuracy in the long-term continuous learning structure across all seasons. In terms of prediction accuracy by algorithm based on learning structure, the long-term continuous learning structure outperformed the short-term continuous learning structure in seasonal prediction accuracy for both waves and sea surface winds. This study confirms the significance and potential of technology for providing data-based marine environment prediction information within autonomous ships. In the future, this technology is expected to facilitate the generation of more practical decision-making information, rather than simply linking it to next-generation waterway products, ship mobility, and route estimation.
목차
1. 서 론
2. 실험 방법 및 자료
3. 실험 결과
4. 결론 및 고찰
후 기
References
키워드
해당간행물 수록 논문
- 국내 크루즈 관광객의 선택속성에 대한 중요도-만족도 분석 : 목포-제주 관광객을 중심으로
- 한국항해항만학회지 제48권 제6호 목차
- 선박 자동조타시스템의 사용성 향상과 상용화를 위한 해상 실증 연구
- 해상 무선 통신에서 결정 피드백을 이용한 시퀀스 등화 방법에 대한 연구
- 제한수역에서 대각도 변침으로 계획항로를 추종하기 위한 선회율에 관한 연구
- 항만도시 수변공간의 소프트 인프라 계획에 관한 연구 - 부산남항 수변공간을 대상으로
- 자동화 컨테이너터미널 안벽 크레인의 AGV 작업 차선 할당에 관한 연구
- 부산항 화물자동차 휴게소 운영 효율성 분석
- 그린포트 조성에 따른 항만 배후지역의 리버빌리티 향상에 관한 연구
- 승선 중 선원의 인터넷 커뮤니케이션이 선원 직업 유지 의도에 미치는 영향
- 선박 복원성 판단을 위한 선회구간 횡요각 예측 모델
- 스마트 항로표지에서의 AIS-ASM 기반 환경 정보 제공을 위한 표준화 방안
- 완성차 판매물류종사자의 중대재해처벌법에 대한 인식수준이 안전의식과 안전행동에 미치는 영향에 관한 연구
- 100MW급 해상풍력발전단지의 최적 배치에 관한 연구
- 글로벌 공급망 압력이 Sea&Air 물동량에 미치는 영향에 관한 연구
- 자율운항선박 지원을 위한 실시간 관측 기반의 해양환경 인공지능 예측 기술 검증
- 리스회계기준 개정이 해운사의 타인자본비용에 미치는 영향
- 국내 컨테이너항만의 관문항 기능변화에 관한 연구
- 운송 서비스 유형별 해운업 파산예측에 관한 연구
- 한국 해역 관측 데이터를 이용한 앙상블 학습 기반 파고 예측
- 국내 항만에서의 해양사고 예방 및 해양환경 보호하기 위한 PSC 점검지원 서비스에 관한 연구
- 표준해사통신용어를 이용한 자율운항선박 원격운용자의 적합성 평가방법
- 충돌 위험 지수 모듈(CRIM) 개발과 이를 이용한 선박 안전운항지원 시스템 개발에 관한 연구
- 드론을 활용한 광파표지의 실외 광도 측정 시스템 연구
참고문헌
관련논문
공학 > 해양공학분야 BEST
- 시스템다이내믹스를 활용한 평택·당진항 수입 승용차 물동량 예측에 관한 연구
- 후쿠시마 원전 오염수 방류에 따른 지자체 대응 전략
- 크루즈관광산업 활성화를 위한 관광경쟁력 제고방안 연구
최근 이용한 논문
교보eBook 첫 방문을 환영 합니다!
신규가입 혜택 지급이 완료 되었습니다.
바로 사용 가능한 교보e캐시 1,000원 (유효기간 7일)
지금 바로 교보eBook의 다양한 콘텐츠를 이용해 보세요!
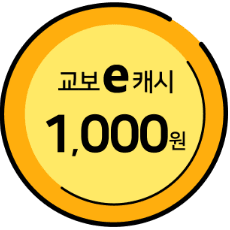