학술논문
전자의무기록 데이터와 머신러닝 기법을 활용한 중환자실 사망 예측모델 개발
이용수 39
- 영문명
- Development of mortality prediction model using electronic health record (EHR) data and machine learning algorithm in intensive care unit (ICU)
- 발행기관
- 한국자료분석학회
- 저자명
- 임선호 이선미
- 간행물 정보
- 『Journal of The Korean Data Analysis Society (JKDAS)』Vol.25 No.5, 1977~1992쪽, 전체 16쪽
- 주제분류
- 자연과학 > 통계학
- 파일형태
- 발행일자
- 2023.10.31
4,720원
구매일시로부터 72시간 이내에 다운로드 가능합니다.
이 학술논문 정보는 (주)교보문고와 각 발행기관 사이에 저작물 이용 계약이 체결된 것으로, 교보문고를 통해 제공되고 있습니다.
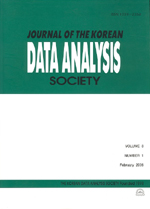
국문 초록
본 연구에서는 전자의무기록(Electronic Health Record, EHR) 데이터와 머신러닝(machine learning) 알고리즘 중 XGBoost(eXtreme Gradient Boosting)를 이용하여 사망예측 모델을 개발하고 평가하였다. 연구자료는 2017년 1월 1일부터 2020년 2월 29일에 1개 3차 의료기관의 내과, 외과중환자실에 입실한 환자 중 5211명(사망군: 685명, 생존군: 4526명)의 전자의무기록이다. 모델 개발 시 사용된 변수는 접근성이 높은 신체사정 기록, 신체사정 기록과 혈액검사, 앞서 언급된 변수와 그 외 다양한 변수로 구분하고 변수 중요도(feature importance)에 따라 각각 14개의 변수를 선정하여 모델을 개발하였다. 연구 결과, 호흡양상, 맥박, RASS(Richmond Agitation Sedation Scale), 수술 후 입실 여부가 다른 변수에 비해 상대적으로 높은 중요도를 보였다. 개발된 모델은 APACHE II, Random Forest 모델, 로지스틱 회귀 모델보다 높은 예측력을 보였다. AUC(Area Under Curve) 측면에서 .93 ~ .98 정도, 정확도 측면에서 0.91 ~ 0.95 정도의 우수한 성능이 관측되었다. 다양한 변수를 활용한 모델이 가장 예측력이 높았고 접근성이 좋은 신체사정 기록만을 활용한 모델도 충분한 예측력을 보였다. 본 연구를 통해 간호사가 환자를 직접 관찰하여 기록하는 정보가 사망예측 시 갖는 중요성과 접근성이 높고 간소화된 변수를 머신러닝 기법과 활용해 효율적인 사망예측을 할 수 있음을 확인할 수 있었다. 향후 의료진은 사망예측에 의한 기록 업무 부담을 줄이고 환자에 관한 정확한 정보를 제공받을 수 있을 것으로 기대된다.
영문 초록
In this study, a mortality prediction model was developed and evaluated using Electronic Health Record (EHR) data and the XGBoost (eXtreme Gradient Boosting) machine learning algorithm. The research dataset comprises EHR records of a total of 5,211 patients who were admitted to the medicine and surgery intensive care units of a tertiary medical institution from January 1, 2017, to February 29, 2020. Models were developed based on the accessibility of variable data, their high correlation with mortality, and their diversity. The research results indicated that variables such as respiratory pattern, pulse rate, RASS (Richmond Agitation-Sedation Scale), and post-surgery admission status showed relatively high importance compared to other variables. Models demonstrated higher predictive power compared to APACHE II, Random Forest and logistic regression models (AUC .93 ~ .98, accuracy .91 ~ .95). The model utilizing various variables showed the highest predictive power, but even a model based solely on accessible physical examination records demonstrated sufficient predictive capability. It was confirmed that patient observations recorded by nurses holds high importance and accessibility in mortality prediction. Also using simplified variables with machine learning techniques can efficiently predict mortality. It is expected that healthcare professionals can reduce the burden of record-keeping for mortality prediction and receive more accurate information about patients.
목차
1. 서론
2. 연구방법
3. 연구결과
4. 논의
5. 결론 및 제언
References
키워드
해당간행물 수록 논문
- 전통적 IPA와 Vavra의 수정된 IPA 비교분석을 통한 설악문화제 활성화 방안
- 112 신고 건수에 영향을 미치는 주요 변수 분석
- 간호대학생 대상 시뮬레이션 실습교육의 효과에 대한 체계적 문헌고찰 및 메타분석
- 토픽 모델을 활용한 지역 글로벌 컨퍼런스에 대한 참여자 만족도 분석
- 전자의무기록 데이터와 머신러닝 기법을 활용한 중환자실 사망 예측모델 개발
- 아시아 국가들의 주관적 건강상태에 미치는 영향요인 분석
- 범주 불균형 금융 문제의 해결을 위한 부스팅 학습
- COVID-19 발생 전후 ESG 투자성과가 유동성에 미치는 영향
- 부산 경남지역의 지역내총생산 동조성에 관한 연구
- 경제정책 불확실성이 장단기 국채 수익률에 미치는 영향 분석
- XAI SHAP 기반 토지 피복 구성에 따른 지표면 온도의 영향 분석
- 동형암호를 활용한 앙상블학습방법의 추론
- 희박한 최소 절대 편차 지지벡터기계
- 기계학습을 이용한 스마트 공장 자료의 불량 분류 모형 개발
- A Study about Inequality in the Scholarly Publishing of Korean Research Institutions using the Gini Coefficient
- Vector Generalized Additive Models for Extreme Rainfall Data Analysis: A case study in South Korea
- 토픽모델링을 이용한 자율주행 연구 동향 분석
- 낙상 경험, 낙상 두려움, 낙상 두려움으로 인한 활동제약이 노쇠에 미치는 영향
- Bottom-Up Projection of Regional Carbon Emissions from Passenger Road Vehicles Based on Discrete-Time Markov Chain
- 임상간호사의 임종간호수행에 미치는 영향요인
- 다문화 학령기 아동의 자아존중감 모형
- 보험회사의 신종자본증권 발행과 기업가치 관련성
- 기술적 비효율성의 오지정이 확률변경모형의 최대가능도 추정에 미치는 영향
- 함수형 시계열 자료를 위한 추정 및 예측모형과 응용
- 토픽모델링과 시계열 분석을 활용한 국내외 독과점 산업 연구 동향 분석
- 금융 시계열 예측 신경망에서의 과적합 완화를 위한 임의 증강 기법
- Journal of The Korean Data Analysis Society (JKDAS) Vol.25 No.5 목차
- 텍스트 마이닝을 활용한 감정 비율 단어 그래프
- 예상치 못한 외국인 순매도로 인한 비대칭적 변동성
참고문헌
교보eBook 첫 방문을 환영 합니다!
신규가입 혜택 지급이 완료 되었습니다.
바로 사용 가능한 교보e캐시 1,000원 (유효기간 7일)
지금 바로 교보eBook의 다양한 콘텐츠를 이용해 보세요!
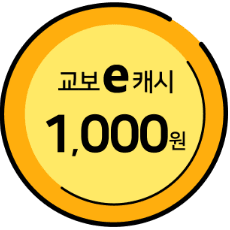