학술논문
기계학습을 활용한 점토 특성에 따른 압축지수 예측 모델 비교
이용수 96
- 영문명
- Comparison of Performance of Machine Learning Models for Predicting Compression Index Based on Clay Properties
- 발행기관
- 한국방재학회
- 저자명
- 이성열(Lee, Sung Yeol) 김진영(Kim, Jinyoung) 강재모(Kang, Jaemo) 백원진(Baek, Won Jin) 윤현준(Yoon, Hyeon Jun)
- 간행물 정보
- 『2. 한국방재학회 논문집』22권 4호, 127~134쪽, 전체 8쪽
- 주제분류
- 공학 > 기타공학
- 파일형태
- 발행일자
- 2022.08.31
4,000원
구매일시로부터 72시간 이내에 다운로드 가능합니다.
이 학술논문 정보는 (주)교보문고와 각 발행기관 사이에 저작물 이용 계약이 체결된 것으로, 교보문고를 통해 제공되고 있습니다.

국문 초록
해안가의 연약지반을 중심으로 대형 구조물 시공이 증가하면서 지반침하에 의한 구조물의 손상 방지에 주의를 기울여야한다. 과거부터 지반침하에 관한 연구가 꾸준히 수행되고 있으며, 침하량은 점토의 압축지수를 통해 산정할 수 있으므로압축지수를 정확히 산정하여 지반의 침하량을 예측하는 것은 매우 중요한 일이다. 따라서 본 연구에서는 부산, 광양, 목포지역을대상으로 점토의 자연함수비, 액성한계, 소성지수, 초기간극비, 압축지수의 데이터를 수집하여 데이터셋을 구축하였다. 구축된데이터셋을 통해 인자들 사이의 상관분석을 실시하였으며, 기계학습 알고리즘인 랜덤포레스트, 다중선형회귀, Ridge, Lasso, SVM, XGBoost, LightGBM, DNN에 적용하여 압축지수 예측 모델을 제시하였다. 또한, 각 모델의 결과를 RMSE와 R2 평가지표로선정하여 비교하였다. 그 결과, 자연함수비, 액성한계, 소성지수, 초기간극비와 압축지수는 높은 상관성을 나타냈으며, 기계학습모델을 비교한 결과 LightGBM 모델이 가장 우수한 성능을 나타냈다.
영문 초록
As the construction of large structures increases primarily on the soft ground along coasts, prevention of damage to the structures due to subsidence should be prioritized. Ground subsidence has been investigated actively. Because the amount of settlement can be calculated based on the compression index of clay, the latter must be calculated accurately. In this study, data pertaining to the natural water content, liquid limit, plasticity index, initial void ratio, and compression index of clay are acquired from Busan, Gwangyang, and Mokpo to construct a dataset for predicting the compression index. Correlation analysis between factors is performed using the dataset, and compression index prediction models are developed using machine learning algorithms, random forest, multiple linear regression, ridge, Lasso, SVM, XGBoost, LightGBM, and DNN. Subsequently, the results of each model are compared in terms of the RMSE and R2. The results show that the natural water content, liquid limit, plasticity index, initial void ratio, and compression index are correlated significantly. Among the machine learning models, LightGBM demonstrates the best performance.
목차
해당간행물 수록 논문
- 지방자치단체의 기능연속성계획 도입에 대한 비판적 고찰
- 일라이트와 카올리나이트의 침강 및 자중압밀 특성
- 인터넷컴퓨터게임시설제공업의 책상 간격에 따른 피난시간 분석
- 초기 화재 사고 시의 계단을 통한 화재 확산에 대한 실험적 연구
- 아스팔트 포장 공법별 장기 소음 저감 성능 평가
- 교육시설 발화요인 원인분석 및 개선방안에 관한 연구: 대학교 중심으로
- 주택용 소방시설의 설치효과 개선방안
- AI 기반 모형을 이용한 홍수위 예측 및 홍수피해 예⋅경보 기법 개발
- 팬틸트 기반의 IR 카메라를 이용한 산불 위치 추정 방법
- 유해화학물질 누출사고 발생 시 경제활동과 비 경제활동 시간의 취약성 분석
- 과거 20년간 국내 산불피해지에서 산사태 발생 경향 연구
- 옥상 헬리포트의 안전 확보를 위한 설치기준 제정에 관한 연구
- 골든타임 확보를 위한 119안전센터 설치기준 개선방안 마련 연구
- 토양수분자료를 활용한 밭가뭄 취약성 평가지표 개발
- GPR 탐사 기법을 활용한 포항지역 액상화 발생사례 조사연구
- 한국방재학회 논문집 22권 4호 목차
- 기계학습을 활용한 점토 특성에 따른 압축지수 예측 모델 비교
- 캠핑카 화재의 실태 및 예방대책 분석
참고문헌
교보eBook 첫 방문을 환영 합니다!
신규가입 혜택 지급이 완료 되었습니다.
바로 사용 가능한 교보e캐시 1,000원 (유효기간 7일)
지금 바로 교보eBook의 다양한 콘텐츠를 이용해 보세요!
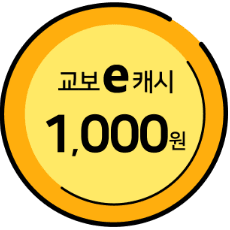