학술논문
관측 강우와 심층신경망의 적용을 통한 도시홍수 분석
이용수 128
- 영문명
- Applying Observed Rainfall and Deep Neural Network for Urban Flood Analysis
- 발행기관
- 한국방재학회
- 저자명
- 김현일(Kim, Hyun Il) 이재영(Lee, Jae Young) 한건연(Han, Kun Yeun) 조재웅(Cho, Jae Woong)
- 간행물 정보
- 『2. 한국방재학회 논문집』 20권1호, 339~350쪽, 전체 12쪽
- 주제분류
- 공학 > 기타공학
- 파일형태
- 발행일자
- 2020.02.28
4,240원
구매일시로부터 72시간 이내에 다운로드 가능합니다.
이 학술논문 정보는 (주)교보문고와 각 발행기관 사이에 저작물 이용 계약이 체결된 것으로, 교보문고를 통해 제공되고 있습니다.
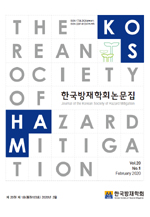
국문 초록
도시유역의 홍수 발생은 주로 저지대의 유수 집중과 배수관거의 만관에 의해 발생하는데, 이는 높은 강우강도와 집중적인양상을 가지는 강우 사상과 함께 나타난다. 도시유역에 대한 유출량 사전 분석을 위해서는 SWMM이 이용될 수 있지만, 대상 유역이 넓고 배수시스템이 복잡한 경우 1차원 도시유출해석에 적지않은 시간이 발생할 수 있다. 또한, 특정 도시 유역에대하여 많지 않은 침수 사례와 침수 유발 관측강우의 부족은 홍수 대비에 대한 기초자료를 제공하는데 어려움이 있다. 홍수를일으킬 위험 강우 발생 시 긴급하게 도시 유출량을 예측하기 위해서, 본 연구에서는 6시간 지속시간을 가지는 전국단위관측 강우사상을 반영하여 심층신경망 모형을 구축하였다. 하나의 강우사상에서 10개의 통계적 특성을 입력 자료로서 고려하였으며, 예측하고자 하는 목표값은 SWMM을 통한 10분 단위 총 누가 월류량으로 하였다. SWMM모의 결과의 적절성을 검증하기위해 2차원 수리해석 모형과 침수흔적도를 연계한 검증을 실시하였다. 대상지역에 대한 SWMM의 모의 시간은 14분이 소요되었으며, 본 연구에서 제시된 심층신경망과 관측 강우 자료 기반 모형은 2~3초 내외에서 수행되었다. 예측은 강남(400) AWS에서2011년 7월 27일에 관측된 강우사상에 대한 총 누가 월류량을 대상으로 하여 모형의 실용성을 검증하였다.
영문 초록
Urban flooding primarily occurs due to flash floods in low-lying regions or insufficient capacity of drainage systems. Urban inundation has become more dangerous to residents because of an increase in impermeable areas, uncertainties in urban sewage planning, and the accumulation of sedimentation in drainage pipes. As an urban watershed with a drainage system and an urbanized river is complicated, a specific time interval is required for the preparation and processing of urban runoff analysis. To allow for rapid simulations of urban runoff estimation during heavy rainfall, a deep neural network model that imitates the conditions of a 6-h duration rainfall was developed in this study. Ten different statistical aspects for each rainfall event were considered as input data, and the total accumulated overflow from a manhole was calculated at intervals of 10 min using storm water management model (SWMM). To verify the accuracy of the results from SWMM, the results obtained from a two-dimensional hydraulic model and an inundation trace map were compared. The computational times for the deep neural network and rainfall data-based models proposed in this study were estimated to be within 1 s, whereas the simulation using SWMM required 14 min. The proposed deep learning model was tested using the total accumulated overflow for the rainfall event observed at Gangnam (400) automatic weather station on July 27, 2011. The simulated results agreed with the observed results in terms of the total accumulated discharge.
목차
1. 서 론
2. 연구 방법
3. 모형의 적용
4. 결 론
감사의 글
References
해당간행물 수록 논문
- 대규모 자연재해의 근린 다양성에 미치는 영향
- 건축물 보·바닥 법정 내화구조 분류체계 개선 방안 연구
- 도심지 터널식 공동구 시공 중 대형건설재해 피해 저감을 위한 Activity Diagram 개발
- 인공지능 기반의 지능형 재난안전관리체계 구축에 관한 연구
- 인천지역 미세먼지에 의한 건강취약성 평가 연구
- 단층의 파괴 과정이 지진해일의 전파에 끼치는 영향
- 풍수해 재해정보 기본통계 교육·훈련과정 기획 연구
- 광역 단위 자연재해저감종합계획의 공간적 범위에 관한 연구
- 개구부에 설치되는 유리의 화재위험성 분석에 관한 연구
- 물류창고의 소화설비 배관 동결방지 실험
- 혼화재료 및 양생조건에 따른 폴리에틸렌 섬유보강 시멘트 복합재료의 에너지흡수성능 강화
- 수돗물 공급관로 내 수질문제 인지를 위한 계측기 위치결정
- 기후변화에 따른 관개저수지의 용수공급 안정성 평가
- 15명의 사상자가 발생한 공장화재의 타임라인 및 사상자 발생 실태 분석
- 주차 차량 전기화재 원인 분석 및 위험 진단에 관한 연구
- 수해폐기물의 효율적 처리를 위한 폐기물 정보 및 통계관리 방안에 관한 연구
- Neuro-Fuzzy 초기화 함수 및 학습자료 구성에 따른 도시침수 위험기준 예측 모델 개선
- 기후변화 적응대책 수립을 위한 설해 위험도 분석 방안
- 관측 강우와 심층신경망의 적용을 통한 도시홍수 분석
- 비정상성을 가정한 서울지역 미래 확률강우량 산정
- 광역자치단체 안전감찰제도 정착 및 개선을 위한 정책제언
- 방화구획을 관통하는 덕트 풍도주위의 스프링클러설비 설치기준에 관한 연구
- Faster R-CNN 학습데이터 구축과 모델을 이용한 안전모 탐지 연구
- 재해영향평가 협의내용의 이행도 제고를 위한 사후관리제도 개선방안 연구
- 비구조적 접근을 통한 원자력발전소 주변지역 사고 피해 저감 방안 연구
- 가속도와 임피던스를 이용한 볼트 접합부 결함탐지기법
- 업무시설의 주요가연물 연소특성에 관한 실험적 연구
- 피난기구 사용인식 및 안전체험교육에 관한 연구
- 딥러닝 기반의 합성곱 신경망을 이용한 화염 및 연기 감지 알고리즘에 관한 연구
- PUFA 메타평가 모형 기반의 재난관리평가 개선방안 연구
- 지하주차장 제연성능 확보방안 연구
- S-RAT 모형을 이용한 홍수유출모의 적용성 검토
- 지반침하예방 최적 모니터링 기술 개발을 위한 기술수준 분석
- GIS 기반의 통계정보와 재해정보를 활용한 재해관리 방안 연구
- 화재시뮬레이션에 의한 보 인접 스프링클러헤드의 동작시간 분석에 관한 연구
- 미세먼지와 아동의 신체 및 정신건강 관계
- 대기 습도가 광 산란 미세먼지 측정에 미치는 영향
- SWMM 이중배수모형(1D-1D) 기반 실시간 도시침수 예측
- 도로터널 내 비상전원 이중화에 의한 안전성 확보 연구
- 단파장 가시광선을 이용한 감광식 연기농도 측정기 개발
- 지역의 재난 취약성 평가방법의 제안과 호우 재난에 적용
- 지역별 재해 유발 강설량의 임계값 추정과 지역구분
- 무도상 판형교의 장대레일 부설을 위한 거더-침목고정장치의 횡저항력 산정
- 기계학습을 이용한 동상방지층의 온도예측에 관한 연구
참고문헌
교보eBook 첫 방문을 환영 합니다!
신규가입 혜택 지급이 완료 되었습니다.
바로 사용 가능한 교보e캐시 1,000원 (유효기간 7일)
지금 바로 교보eBook의 다양한 콘텐츠를 이용해 보세요!
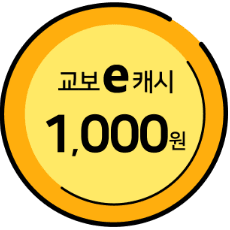