학술논문
수학학습 정의적 영역을 이용한 대학 이공계 전공 예측 기계학습 분석
이용수 2
- 영문명
- Machine Learning Analysis for Predicting College STEM Majors with the Affective Domain in Learning Mathematics
- 발행기관
- 대한수학교육학회
- 저자명
- 가연석(Yeonseok Ka) 황지현(Jihyun Hwang)
- 간행물 정보
- 『수학교육학연구』제34권 1호, 153~170쪽, 전체 18쪽
- 주제분류
- 사회과학 > 교육학
- 파일형태
- 발행일자
- 2024.02.28
4,960원
구매일시로부터 72시간 이내에 다운로드 가능합니다.
이 학술논문 정보는 (주)교보문고와 각 발행기관 사이에 저작물 이용 계약이 체결된 것으로, 교보문고를 통해 제공되고 있습니다.
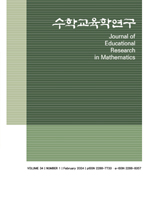
국문 초록
본 연구는 한국교육종단연구 2005의 자료를 활용하여 중학교, 고등학교 재학 중 학생이 가진 정의적 영역을 바탕으로 대학 진학시 이공계 전공 선택을 예측하는 모형을 개발하고 분석하였다. 중학교 2학년과 고등학교 1학년 자료에서 도구적 동기, 수학에 대한내재적 동기, 수학에 대한 자기효능감, 지능에 대한 신념을 예측을 의한 독립변수로 활용하였다. 로지스틱 회귀모형, 다층퍼셉트론(Multi Layer Perceptron) 모형, 랜덤포레스트(Random Forest) 모형을 적용하였고 다양한 지표를 종합적으로 고려하여 최적의예측 모형을 찾고자 하였다. 중학교 2학년 자료을 활용한 경우, 이 세 모형 중에서 다층퍼셉트론모형이 다른 모형들에 비해 더 우수한예측력을 보였다. 고등학교 1학년의 자료를 활용한 경우에는, 모든 모형이 좋은 예측력을 보여주지 못하였다. 중학교 2학년 자료를바탕으로 한 모형이 실제 활용 가능성있다고 판단하였다. 이러한 결과는 수학에 대한 정의적 영역이 이공계 전공 선택에 있어서중요한 요소이지만, 고등학교 1학년 시기의 전공 예측을 위해서는 더 다양한 변수와 복잡한 모형이 필요함을 시사한다. 또한, 기계학습 모형의 한계를 인지하면서 전공 선택과 수학의 정의적 요소 간 관련성에 대한 이론적 논의가 더 필요하며 더불어 다양한 예측모형이 개발되어 실제 현장에서 사용되기를 기대한다.
영문 초록
This study developed and analyzed models to predict the selection of STEM majors in college based on the affective domain characteristics of students during their middle and high school years, using data from the Korean Education Longitudinal Study 2005. Instrumental motivation, intrinsic motivation towards mathematics, self-efficacy in mathematics, and beliefs about intelligence were used as independent variables for prediction based on the data from the second grade of middle school and the first grade of high school. Logistic models, Multi Layer Perceptron (MLP) models, and Random Forest models were applied, and the optimal prediction model was sought by comprehensively considering various indicators. In the case of using the data from the second grade of middle school, the MLP model showed superior predictive power compared to other models. In the case of using the data from the first grade of high school, all models did not show good predictive power. The model based on the data from the second grade of middle school was deemed to have practical applicability. These results suggest that while the affective domain regarding mathematics is an important factor in the choice of a STEM major, more diverse variables and complex models are needed for predicting majors during high school years. Additionally, while recognizing the limitations of machine learning models, more theoretical discussions are needed on the relationship between major selection and the affective elements of mathematics, and it is hoped that various prediction models will be developed and used in actual practice.
목차
Ⅰ. 서론
Ⅱ. 선행연구 분석
Ⅲ. 연구 방법
Ⅳ. 연구 결과
Ⅴ. 결론 및 논의
CONFLICTS OF INTEREST
REFERENCES
해당간행물 수록 논문
- 잠재프로파일 분석을 통한 초등학생들의 산술-대수 구조 감각 탐색
- 수학교육학연구 제34권 1호 목차
- 직관주의 수학 및 고전 수학 관점에 따른 교과서의 귀류법 증명에서 무리수 √2의 존재성 문제
- 미국과 한국 초등 예비교사의 노티싱 역량과 유형 비교
- 비구조화된 의사결정형 문제해결에서 초등수학영재 집단의 창의적 시너지 사례 연구
- 과제의 반복적인 수정과 실행을 통한 수학교사의 집단 창의성 개념에 대한 지식과 교수 활동의 변화 분석
- 수학적 모델링 과정에서 수학적 기호의 의미작용 분석
- 활동 이론에 입각한 디지털 테크놀로지 활용 수학 수업의 역동성 분석
- 수학학습 정의적 영역을 이용한 대학 이공계 전공 예측 기계학습 분석
참고문헌
관련논문
최근 이용한 논문
교보eBook 첫 방문을 환영 합니다!
신규가입 혜택 지급이 완료 되었습니다.
바로 사용 가능한 교보e캐시 1,000원 (유효기간 7일)
지금 바로 교보eBook의 다양한 콘텐츠를 이용해 보세요!
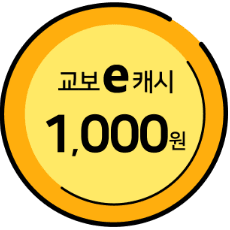