학술논문
머신러닝을 활용한 4년제 대학 중도탈락률 예측요인 탐색
이용수 140
- 영문명
- Exploring Predictive Factors of Dropout Rate of 4-year Universities using Machine Learning
- 발행기관
- 한국교육재정경제학회
- 저자명
- 문명현 김길재
- 간행물 정보
- 『교육재정경제연구』제32권 제2호, 57~77쪽, 전체 21쪽
- 주제분류
- 사회과학 > 교육학
- 파일형태
- 발행일자
- 2023.06.30
5,320원
구매일시로부터 72시간 이내에 다운로드 가능합니다.
이 학술논문 정보는 (주)교보문고와 각 발행기관 사이에 저작물 이용 계약이 체결된 것으로, 교보문고를 통해 제공되고 있습니다.
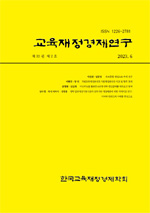
국문 초록
본 연구의 목적은 머신러닝 알고리즘을 활용하여 4년제 대학 중도탈락률을 예측하는 요인을 밝히고자 하였다. 머신러닝 알고리즘은 랜덤포레스트와 XGBoost을 사용하였고, 모델 개발을 위한 자료는 한국대학평가원의 대학통계와 대학알리미 정보공시 자료를 활용하였다. 수집된 자료는 2011년부터 2021년까지의 공시 자료를 사용하였으며, 교육재정, 교육여건, 교육 및 연구성과에 해당하는 총 17개 변인을 설명변수로, 중도탈락률을 종속변수로 분석하였다. 최종적인 랜덤포레스트와 XGBoost 모델은 중도탈락률 예측함에 있어 각각 65%와 66%로 중도탈락률의 변동성을 설명하는 것으로 나타났다. 머신러닝의 두 가지 모델을 통해 변수 중요도 기준 상위 10개의 설명변인을 도출하였으며, 재학생 충원율을 제외하고 공통요인을 확인하였다. 모델은 학생 1인당 자료구입비, 전임교원 1인당 교내연구비, 전임 교원 1인당 교외 연구비, 신입생 충원율, 직원 1인당 학생 수, 취업률을 중도탈락을 예측하는 중요 변수로 도출하였다. 두 알고리즘에서 공통으로 예측한 5개의 중요 설명변수의 부분 의존성 도표를 통해, 설명변수의 변화에 따라 대학 중도탈락률 예측값이 어떻게 변화하는지 분석하였다. 랜덤포레스트와 XGBoost 간 곡선의 차이는 있으나 전반적으로 중요 설명변수의 변화 곡선의 방향과 변화 추이가 유사한 것으로 나타났다. 5개의 중요 설명변수의 향상은 중도탈락률 감소를 에측하는 음의 상관이 있는 것으로 나타났다. 이러한 연구결과를 바탕으로 주요 시사점과 후속 연구를 위한 제언을 제시하였다.
영문 초록
The purpose of this article was to identify factors that predict the dropout rate in 4-year universities using machine learning algorithms. The Random Forest and XGBoost algorithms were employed, and data from the Korean Council for University Education and the University Information Disclosure System were utilized for model development. The collected data spanned from 2011 to 2021 and included a total of 17 independent variables related to educational finance, educational conditions, and educational and research performance. The dropout rate was analyzed as the dependent variable. The final Random Forest and XGBoost models demonstrated the ability to explain 65% and 66% of the variability in the dropout rate, respectively. Using the two machine learning models, the top 10 explanatory variables were identified based on their importance, excluding the student enrollment rate. The models identified the following variables as important predictors of the dropout rate: expenditure per student for academic materials, research budget per full-time faculty member within the university, research budget per full-time faculty member outside the university, freshman enrollment rate, employee-to-student ratio, and employment rate. By examining the partial dependence plots of the five common important explanatory variables, it was analyzed how the predicted dropout rate changes in response to variations in these variables. Although there were differences in the curves between Random Forest and XGBoost, the overall direction and trends of the curves for the important explanatory variables were found to be similar. The improvement of these five important variables was found to have a negative correlation with the dropout rate, indicating a decrease in the predicted dropout rate. Based on these research findings, key implications and suggestions for further studies were provided.
목차
Ⅰ. 서론
Ⅱ. 이론적 배경
Ⅲ. 연구 방법
Ⅳ. 연구 결과
Ⅴ. 결론
참고문헌
해당간행물 수록 논문
참고문헌
관련논문
사회과학 > 교육학분야 BEST
더보기사회과학 > 교육학분야 NEW
- 핀란드, 미국, 한국 학생의 기대교육포부 수준과 학업성취도의 관계: 부모의 SES 집단 비교를 중심으로
- 청년과 사회이동: 교육, 직업 그리고 자산
- 부모의 사회경제적 지위에 따른 자녀의 기대 직업 지위 차이 변화: 2000-2022
최근 이용한 논문
교보eBook 첫 방문을 환영 합니다!
신규가입 혜택 지급이 완료 되었습니다.
바로 사용 가능한 교보e캐시 1,000원 (유효기간 7일)
지금 바로 교보eBook의 다양한 콘텐츠를 이용해 보세요!
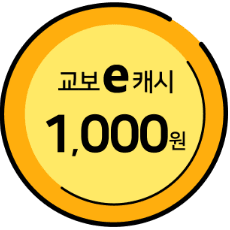