학술논문
재범의 위험성 예측 알고리즘과 설명가능성 및 공정성의 문제
이용수 497
- 영문명
- Recidivism prediction algorithm and issues of explainability and fairness
- 발행기관
- 대검찰청
- 저자명
- 양종모(Yang, Jongmo)
- 간행물 정보
- 『형사법의 신동향』제70호, 207~240쪽, 전체 34쪽
- 주제분류
- 법학 > 법학
- 파일형태
- 발행일자
- 2021.03.31
무료
구매일시로부터 72시간 이내에 다운로드 가능합니다.
이 학술논문 정보는 (주)교보문고와 각 발행기관 사이에 저작물 이용 계약이 체결된 것으로, 교보문고를 통해 제공되고 있습니다.

국문 초록
인공지능 특히 머신러닝 알고리즘은 의료 분야나 금융 분야뿐만 아니라 법 분야에서 재범의 위험성 예측과 범죄 예측 등에 활용되고 있다. 이러한 인공지능에서 가장 문제되는 것은 블랙박스 속성으로, 인공지능의 작동 기제를 인간이 제대로 이해하지 못한다는 것이다. 재범의 위험성 예측 등에 사용되는 딥러닝 또는 머신러닝 아키텍처의 내부 작동은 점점 더 복잡하고 불투명해지고 있다. 다른 분야도 마찬가지이지만, 법 분야에서 이러한 인공지능의 의사 결정 과정의 투명성을 보장하고 나아가, 그 의사결정과정을 비전문가들에게 설명할 수 있는 체계가 필수적이다. 법 분야에서의 의사결정은 그 결과뿐만 아니라, 그 절차적 정당성이 매우 중요하기 때문이다. 법 분야 의사결정 알고리즘의 대표 격으로 거론되는 재범 위험성 예측 알고리즘 COMPAS는 피고인의 석방 여부 결정이나 형량 결정 등에 사용되고 있다. COMPAS의 도입・시행 이후, 이 알고리즘을 둘러싼 논란과 여러 비판에서 보듯 미국에서 널리 사용되고 있는 COMPAS는 인종차별적인 결과를 내는 등 문제점이 많은 것으로 드러났다. 인공지능의 블랙박스 속성 때문에 COMPAS의 작동 기제를 알지 못한다. 편향적 결과로 인해 피해를 본 사람도 이와 같은 블랙박스 속성 때문에 그 결과를 탄핵할 수 없다. 이러한 블랙박스로 인한 인공지능 알고리즘의 문제점을 해결하려는 해법이 필요하다. 그 해법의 가장 큰 줄기가 바로 ‘설명 가능한 인공지능’이다. 설명가능 인공지능은 인공지능이 내린 의사결정의 이유를 사람이 이해할 수 있는 방식으로 제시하는 인공지능을 일컫는다. 인공지능의 발전에 상당한 기여를 했다는 평가를 받고 있는 미방위고등연구계획국은 몇 년 전부터 이러한 설명 가능한 인공지능 연구에 대해 막대한 지원을 하고 있다.
물론 인공지능 연구자 중에는 설명 가능한 인공지능의 가치에 대해 의문을 던지고 있는 이도 있다. 심지어 인간의 의사결정과정도 쉽게 설명될 수 있는 것이 아니라며, 인공지능의 의사결정 과정의 설명 가능성과 실용성 모두를 의문시한다. 이런 회의론에도 불구하고, 법 분야에서 인간의 의사결정을 대체하는 수준의 인공지능 알고리즘이 개발되고, 자리 잡기 위해서는 설명 가능한 인공지능 과제는 반드시 해결되어야 한다. 따라서 본 연구에서는 이러한 설명 가능한 인공지능의 개념과 그것이 법 분야 인공지능 알고리즘 구현과 활용에 얼마나 중요한지를 따져보고, 그것의 실현가능성을 점쳐 본다.
이런 설명 가능한 인공지능으로 인하여 편향성이나 차별 등 공정성 가치를 저해하는 등 여러 가지 문제점이 해결될 것으로 기대한다.
영문 초록
Artificial intelligence, in particular, machine learning algorithms are used not only in the medical field or finance field, but also in the law field to predict the risk of recidivism, predict crime, and improve legal services. The most problematic aspect of such artificial intelligence is the black box problem, which is that humans do not properly understand the mechanism of operation of artificial intelligence. The inner workings of deep learning or machine learning architectures are becoming increasingly complex and opaque. As is the case in other fields, in the field of law, a system is essential to ensure transparency in the decision-making process of artificial intelligence, and to explain the decision-making process to non-experts. Decision-making in the field of law is because not only the outcome, but also its procedural justification is very important. The recidivism risk prediction algorithm COMPAS, which is mentioned as a representative case of decision-making algorithms in the legal field, is used for determining whether to release a defendant or determining a sentence. Since the introduction and implementation of COMPAS, as seen in the controversy and criticisms surrounding this algorithm, COMPAS, which is widely used in the United States, has been found to have many problems, such as racist results. Due to the black box nature of artificial intelligence, we do not know the mechanism of COMPAS. Even those who have suffered damage due to the biased result cannot challenge the result because of the black box property. There is a need for a solution to solve the problem of artificial intelligence algorithms caused by such black boxes. The biggest stem of the solution is ‘explainable artificial intelligence . Explainable artificial intelligence refers to artificial intelligence that presents the reasons for decisions made by artificial intelligence in a way that humans can understand. The U.S. Defense Advanced Research Projects Agency (Darpa), which is considered to have made a significant contribution to the advancement of artificial intelligence, began to provide enormous support for such explanatory artificial intelligence research several years ago.
Of course, some of the AI researchers are questioning the value of explainable AI. Even the human decision-making process cannot be explained easily, and they question both the explanatory feasibility and practicality of the decision-making process of artificial intelligence. Despite this skepticism, in order to develop and establish an artificial intelligence algorithm at a level that replaces human decision-making in the field of law, an explainable AI task must be solved. Therefore, in this study, the concept of explainable artificial intelligence and how important it is to the implementation and application of artificial intelligence algorithms in the legal field, and its feasibility are examined.
It is expected that a large number of problems that hinder fairness values such as bias and discrimination will be solved by such explainable artificial intelligence. Apart from that, I would like to discuss with you whether there is a way to solve the problem of impairing fairness value caused by artificial intelligence algorithms.
목차
Ⅰ. 서 론
Ⅱ. 재범의 위험성 예측 알고리즘과 문제점
Ⅲ. 설명 가능한 인공지능 알고리즘 이슈
Ⅳ. 결 론
※ 참고문헌
키워드
해당간행물 수록 논문
- 안보형사법상 증거재판주의와 자유심증주의의 이론과 실제
- 채용비리는 업무방해인가
- 아트펀드와 배임죄
- 고위공직자범죄수사처 검사의 영장청구권에 관한 검토
- 영장주의 예외인 형사소송법 제216조 제3항의 적용 범위와 사후영장
- 특가법 어린이보호구역치사상에 관한 판결분석과 교통조사 실무대응
- ʻ도촬행위ʼ 처벌규정의 도입 필요성 및 그 내용에 관한 고찰
- 영상녹화의 적극적 활용 및 증거능력 인정 필요성에 대한 검토
- 성폭력처벌법 제3조 제1항의 ʻ주거ʼ에 대한 제한적 해석론
- 재건축 관련 범죄의 형법이론적· 형사정책적 대응방안
- 재범의 위험성 예측 알고리즘과 설명가능성 및 공정성의 문제
- 형사소송법 제308조가 규정하고 있는 자유심증주의의 의미와 그 한계에 관하여
- 범죄피해재산의 몰수·추징 및 피해자 환부 제도에 대한 비교법적 고찰
참고문헌
관련논문
최근 이용한 논문
교보eBook 첫 방문을 환영 합니다!
신규가입 혜택 지급이 완료 되었습니다.
바로 사용 가능한 교보e캐시 1,000원 (유효기간 7일)
지금 바로 교보eBook의 다양한 콘텐츠를 이용해 보세요!
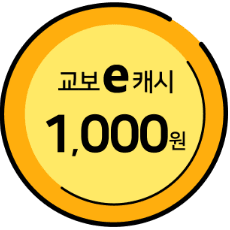