학술논문
여론조사에서 무응답 대체를 통한 선거 결과 예측
이용수 37
- 영문명
- Predicting Election Results through Non-Response Substitution in Polls
- 발행기관
- 한국자료분석학회
- 저자명
- 권민수(MinSoo Kwon) 성진용(JinYong Sung) 최승배(Seung Bae Choi) 강창완(Changwan Kang)
- 간행물 정보
- 『Journal of The Korean Data Analysis Society (JKDAS)』Vol.26 No.4, 1005~1013쪽, 전체 9쪽
- 주제분류
- 자연과학 > 통계학
- 파일형태
- 발행일자
- 2024.08.30
4,000원
구매일시로부터 72시간 이내에 다운로드 가능합니다.
이 학술논문 정보는 (주)교보문고와 각 발행기관 사이에 저작물 이용 계약이 체결된 것으로, 교보문고를 통해 제공되고 있습니다.
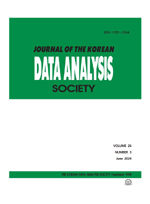
국문 초록
선거 여론조사를 통해 나타나는 결과에서 후보들의 지지율은 국민의 주요 관심사일 뿐만 아니라 해당 정당의 선거 전략 수립을 위해 중요한 요소로 작용한다. 그러나 선거 여론조사의 부정확하거나 왜곡된 결과가 나오면 그 영향력 때문에 큰 문제를 일으킬 수 있어 여론조사에 관한 논란이 자주 발생한다. 여론조사 예측 결과들이 실제 선거 결과와 다른 사례들이 다수 나타남에 따라 여론조사 관련 기관인 정당이나 조사기관 등에서 선거 예측의 정확성 제고를 위해 노력하고 있다. 본 연구에서는 제20대 대통령 선거, 2021년 부산시장 보궐선거, 제8회 전국동시지방선거(부산광역시 남구, 김해시장) 여론조사 데이터를 사용하여 머신러닝(Machine Learning) 알고리즘을 통해 무응답을 대체하여 선거 예측의 정확성을 높이고자 한다. 이를 위해서 첫째, 선거여론조사 문항 중 후보 선택 문항에서 무응답(모르겠다/없다)을 제외한 데이터를 훈련용 데이터(train data)로, 무응답(모르겠다/없다)을 선택한 데이터는 검증용 데이터(test data)로 데이터분할을 하였다. 둘째, 머신러닝 기법인 Random Forest, XGBoost, LightGBM 방법을 적용하였으며 훈련용 데이터를 통해 후보 예측 시 문항 중요도를 도출하여 상위 3개의 문항울 선택하였다. 셋째, 훈련용 데이터를 기반으로 학습된 모델을 통해 검증용 데이터의 무응답을 한 명의 후보를 선택한 응답으로 예측, 대체하여 해당 데이터와 훈련용 데이터를 합쳐 후보별 득표율을 산출하였다. 무응답을 대체하여 후보별 득표율과 실제 선거 결과를 비교한 결과 Random Forest 방법이 무응답 대체를 통한 선거 예측의 정확성 제고를 위한 모델로 적합한 것으로 확인하였다.
영문 초록
In the results of the election polls, the candidates' approval ratings are not only a major concern of the people, but also an important factor in establishing the election strategy of the party concerned. However, if there is an inaccurate or distorted result of the election poll, it can cause a big problem because of its influence, and the controversy about the poll often arises. There are many cases in which the actual election results are different from the results of the polls, and political parties and research institutes, which are related to the polls, are making efforts to improve the accuracy of the election forecasts. The purpose of this study is to improve the accuracy of election prediction by replacing non-response through machine learning algorithm using the survey data of the 20th Presidential Election, the 2021 Busan Mayor By-election, and the 8th National Simultaneous Local Election (Busan Metropolitan City, Nam-gu, Gimhae Mayor). For this purpose, first, the data excluding the non-response (I do not know/don't know) was separated into train data and the data selected as non-response (I do not know/don't know) was separated into test data. Second, Random Forest, XGBoost, and LightGBM methods were applied, and only the top three questions were used by deriving the importance of questions when predicting candidates through train data. Third, through the model learned based on the train data, the non-response of the test data was replaced with the response of one candidate, and the corresponding data and the train data were combined to calculate the percentage of votes for each candidate. As a result of comparing the actual election results with the percentage of votes for each candidate by replacing the non-response, it was confirmed that the Random Forest method is suitable for the model for improving the accuracy of election prediction through non-response substitution.
목차
1. 서론
2. 무응답 대체를 위한 머신러닝 모델
3. 데이터 분석
4. 결론
References
해당간행물 수록 논문
- 수출이 기업의 연구개발투자에 미치는 영향
- 코로나19 팬데믹이 의료서비스 이용에 미친 영향 분석
- A Study on the Effect of Research and Development Expenditures on Firm Value and Corporate Performance
- 분포동학을 통한 중국 위안화 환율의 안정성 및 결정요인 분석
- 베타의 변동성과 주식의 기대수익률
- 조선업 생산직 근로자의 셀프리더십이 일탈행동에 미치는 영향: 자기효능감과 직무만족의 매개효과
- 노인의 성별에 따른 골관절염 유병 관련 영향요인
- 텍스트 마이닝 기반 고등학교 일본어 교육에 적합한 일본 대중음악 선별 연구
- 토픽모델링에 기반한 R&D 투자 포트폴리오 분석 및 예측
- 여론조사에서 무응답 대체를 통한 선거 결과 예측
- ARIMAX-Threshold 모형을 이용한 20대 이하 마약류 사범 검거 수 시계열 분석
- Word2Vec과 엔트로피를 활용한 연구동향 분석
- 측정오차 공변량을 고려한 포아송 소지역 모델의 준모수적 계층적 베이즈 추정
- 경제정책 불확실성이 한국 금융 불안에 미치는 영향 분석
- 공무원 채용시험 과목의 변화가 공공봉사동기에 미치는 영향: 이중차분법을 중심으로
- 상장지수펀드의 성과에 영향을 미치는 상장지수펀드의 횡단면 특성
- 머신러닝을 통한 관절염 환자의 삶의 질 영향 분석
- 라포형성 수사기법을 통한 수사 면담과 메타기억이 증언의 정보량과 정확도에 미치는 영향: 대인존재감의 조절효과를 중심으로
- 가정 내 아동학대 행위자 특성에 따른 유형분류: 잠재계층분석(Latent Class Analysis)을 중심으로
- Journal of The Korean Data Analysis Society (JKDAS) Vol.26 No.4 목차
참고문헌
관련논문
자연과학 > 통계학분야 BEST
더보기자연과학 > 통계학분야 NEW
- Journal of The Korean Data Analysis Society (JKDAS) Vol.27 No.1 Contents
- A Research on ESG Commitment, Governance, and Firm Value
- 영상 콘텐츠 추천시스템을 위한 AWSGLD 알고리즘
최근 이용한 논문
교보eBook 첫 방문을 환영 합니다!
신규가입 혜택 지급이 완료 되었습니다.
바로 사용 가능한 교보e캐시 1,000원 (유효기간 7일)
지금 바로 교보eBook의 다양한 콘텐츠를 이용해 보세요!
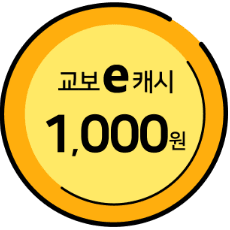