학술논문
머신러닝을 활용한 한계대학 예측 요인 탐색 연구
이용수 224
- 영문명
- A study on exploring factors for predicting business crisis universities using machine learning : Focusing on Random Forest Model
- 발행기관
- 한국교육재정경제학회
- 저자명
- 김경민 이종현
- 간행물 정보
- 『교육재정경제연구』제32권 제1호, 1~30쪽, 전체 30쪽
- 주제분류
- 사회과학 > 교육학
- 파일형태
- 발행일자
- 2023.03.30
6,400원
구매일시로부터 72시간 이내에 다운로드 가능합니다.
이 학술논문 정보는 (주)교보문고와 각 발행기관 사이에 저작물 이용 계약이 체결된 것으로, 교보문고를 통해 제공되고 있습니다.
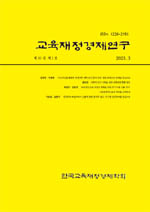
국문 초록
본 연구는 머신러닝(Machine Learning)을 활용하여 한계대학 예측 요인을 발견함으로써 정부와 사회적인 대응책을 마련하기 위한 기준을 찾아보고자 한다. 2012년부터 2020년 사이에 정부가 추진한 대학구조조정 평가에서 부실대학, 재정지원제한대학, 역량강화 대학 등에서 하위의 평가 결과를 받은 대학을 한계대학으로 정의하고, 대학의 구성요소인 학생, 교원, 재무자료 등의 데이터를 활용하여 한계대학을 예측할 수 있는 요인을 도출하는 것을 목표로 하였다. 분석결과 랜덤 포레스트를 사용하는 경우 로지스틱 회귀분석모형에 비해 보다 정확하게 한계대학을 파악할 수 있었으며, shap 분석과 통계적(t-검정)으로 한계대학을 예측하는데 중요한 역할을 하는 요인을 찾아낸 결과는 다음과 같다. 우선 한계대학 예측을 위해 동일한 데이터에 대해 로지스틱 회귀분석모형과 랜덤 포레스트를 각각 적용하여 예측력을 평가할 수 있는 ROC-AUC 값을 확인해본 결과, 로지스틱 회귀분석모형은 대학 0.819, 전문대학 0.854로 평가되었고, 랜덤 포레스트는 대학 0.927, 전문대학 0.943으로 평가되었다. 대학의 경우 한계대학 예측에 정(+)의 영향을 미치는 요인은 2개로 중도탈락율, 지역_경남임을 알 수 있었다. 정(+)의 영향을 미치는 요인이 증가하거나 해당되면 한계대학으로 예측될 가능성이 높아진다는 의미이다. 반면 한계대학 예측에 부(-)의 영향을 미치는 요인은 8개로 학생1인당교육비, 교육비환원율, 재학생충원율, 유지충원율, 신입생충원율, 취업률, 운영경비부담율, 전임교원1인당연구비임을 알 수 있었다. 전문대학의 경우 한계대학 예측에 정(+)의 영향을 미치는 요인은 5개로 이월금비율, 교원확보율, 지역_강원, 중도탈락율, 부채비율임을 알 수 있었다. 반면 한계대학 예측에 부(-)의 영향을 미치는 요인은 9개로 학생1인당교육비, 재학생충원율, 대학규모, 교육비환원율, 유지충원율, 운영경비부담율, 신입생충원율, 장학금지급율, 전임교원1인당연구비임을 알 수 있었다. 본 연구의 공헌점은 최근 널리 활용되는 머신러닝 기법을 활용하여 한계대학을 예측할 수 있는 새로운 방법론을 제시하고자 하였다. 대학알리미에서 공시되고 있어 접근이 쉬운 데이터를 바탕으로 한계대학을 예측할 수 있는 모형을 설계하고, 그 중 한계대학 예측과 깊은 연관이 있는 항목을 찾아냄으로써 한계대학 예측가능성을 제시하였다.
영문 초록
This study seeks to find the criteria for preparing government and social countermeasures by using machine learning to explore predictive factors for universities in business crisis. Between 2012 and 2020, universities with low evaluation results in the government's university restructuring evaluation are defined as universities in business crisis. The goal was to discover factors that could predict a university in business crisis by using data such as students, faculty, and financial data, which are university components. As a result of the analysis, when using the Random Forest, it was possible to accurately identify universities in business crisis compared to the Logistic Regression model. The results of predicting universities in business crisis through shap analysis and statistical (t-test) are as follows. First of all, the ROC-AUC value that can evaluate the predictive power was checked by applying the logistic regression model and random forest to the same data for the prediction of universities in business crisis, and the logistic regression model was evaluated as 0.819 for universities and 0.854 for colleges. , Random Forest was evaluated as 0.927for universities and 0.943 for colleges. In the case of universities, the factors that have a positive(+) influence on the prediction of universities in business crisis are the dropout rate, region_Gyeongnam. On the other hand, the factors that have a negative(-) effect on the prediction of universities in business crisis are education expenses per student, education expense reimbursement rate, enrollment rate, maintenance recruitment rate, new student recruitment rate, employment rate, operating expense burden rate, and research expenses per full-time faculty member. In the case of colleges, the factors that have a positive (+) influence on the prediction of universities in business crisis are the carryover rate, teacher retention rate, region_Gangwon, dropout rate and debt ratio. On the other hand, the factors that have a negative(-) effect on the prediction of universities in business crisis are education expenses per student, student recruitment rate, university size, education expense reimbursement rate, maintenance recruitment rate, operating expense burden rate, new student recruitment rate, scholarship payment rate, and research expenses per full-time faculty member. The contribution of this study is to propose a new methodology to predict universities in business crisis using machine learning techniques, which are widely used recently.
목차
Ⅰ. 서론
Ⅱ. 이론적 배경
Ⅲ. 연구방법
Ⅳ. 연구결과
Ⅴ. 결론
참고문헌
키워드
해당간행물 수록 논문
참고문헌
교보eBook 첫 방문을 환영 합니다!
신규가입 혜택 지급이 완료 되었습니다.
바로 사용 가능한 교보e캐시 1,000원 (유효기간 7일)
지금 바로 교보eBook의 다양한 콘텐츠를 이용해 보세요!
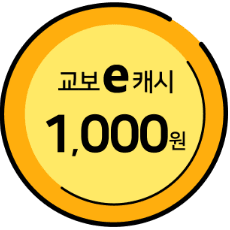