학술논문
합성곱 신경망과 머신러닝을 활용한 화물운반 주의 표식 인식
이용수 32
- 영문명
- Recognition of Logistics Safety Labels Using Convolutional Neural Network and Machine Learning
- 발행기관
- 한국물류학회
- 저자명
- 간행물 정보
- 『물류학회지』제32권 제3호, 67~76쪽, 전체 10쪽
- 주제분류
- 경제경영 > 무역학
- 파일형태
- 발행일자
- 2022.06.30
4,000원
구매일시로부터 72시간 이내에 다운로드 가능합니다.
이 학술논문 정보는 (주)교보문고와 각 발행기관 사이에 저작물 이용 계약이 체결된 것으로, 교보문고를 통해 제공되고 있습니다.
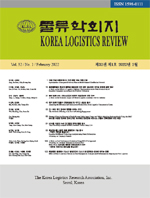
국문 초록
4차산업혁명, e-commerce 발달 및 코로나 시대 도래와 함께 물류량이 증가함에 따라 상·하차 자동화의 개발수요가 증가하고있다. 하역 자동화의 핵심기술인 운반객체분류는 컴퓨터 비전 및 인공지능의 기술과 함께 발전하고 있으며, EU의 인지 및 자율로봇 개발 프로젝트(Robotic Logistics)의 ‘Empticon Ⅱ’가 대표적이다. 해당 모델은 3D카메라로 커피콩 자루 더미를 촬영한 후 컴퓨터 비전 기술을 통해 위치를 파악하며 위치파악 및 인식 정확도를 높이기 위해 취급할 수 있는 객체의 모양과 크기를 사전에 학습했다. 객체탐지성능은 사전 학습 객체의 양과 비례하지만 다양한 크기와 질감을 가진 모든 객체를 사전에 학습하기엔 어렵다는단점이 있다. 본 연구는 운반 주의 표식을 부착한 다양한 크기의 상자를 인식하고 분류하여 하역 자동화기기를 컨트롤 할 수 있는모델 개발의 기초학습모델 및 자료를 개발하고자 한다. 모델 개발을 위해 가장 보편적인 4가지 운반 주의 표식을 선정하였으나, 온라인으로 배포되고 있는 데이터 세트가 없어 연구자가 직접 촬영했다. 촬영 및 수집한 데이터의 양이 1천 장을 넘지 않았기 때문에 합성곱 신경망 기반 분류기를 학습하는 데 적합하지 않았다. 더하여 앙상블 기반 머신러닝 분류기 성능은 높지 않았기 때문에 합성곱 신경망을 사용하여 운반 주의 표식 특징추출기와 머신러닝 분류기를 결합한 모델을 개발했다. 실제 테스트 단계에선학습에 사용되지 않고 노이즈가 있는 사진을 사용했으며, 상자 주변 배경을 제거하여 성능저하 문제를 해결했다. 그 결과Precision 0.9675, Recall 0.9575, F1-score 0.9625를 달성해 우수한 성능을 보였으며 육안으로 판별할 수 있는 차이가 큰 운반 주의표식의 경우 학습 데이터양이 적을 때 합성곱 신경망 특징추출기와 앙상블 머신러닝 분류기 결합 모델의 성능이 우수하다는 것을밝혔다. 본 연구는 운반 주의 표식 데이터 세트 수집, 전처리와 인식모델 개발 및 활용연구의 과정에 대해 연구했으며 하역 자동화연구의 기초 자료로써 학문적 함의를 갖는다.
영문 초록
The development speed of the loading and unloading automation is accelerating due to such initiators as the prevailing 4th industrial revolution technologies, the expansion of e-commerce, and the increase in logistics volumes inevitably by the advent of the corona era. The logistic-object classification evolves along with the computer vision as a core technology for the automatic loading and unloading. As a practical example, ‘Empticon Ⅱ’ of the ‘Roblog’ project identifies the location of the coffee bean sack to be transported. The 3D camera is used for photographing the sack and analyzing it through the computer vision technology. Tremendous amounts of data training efforts were required in advance to make the object detection function better. This method, however, has a disadvantage in that it is difficult to learn and utilize all the objects of various sizes and textures in advance because the object detection performance is proportional to the amount of objects learned in advance. Most of the objects handled are boxes. To prevent damage from using the unloading robot, logistics safety labels are attached to the box. This study aims to make a model that can control automatic loading and unloading robots by recognizing and classifying logistics safety labels attached to boxes of various sizes. To train the machine learning model, the most common logistics safety labels attached to the box or printed need to be obtained as training examples. Unfortunately, there was no such dataset being distributed as an open source, so the authors built a dataset by themselves. A classifier using the ensemble model was created. In order to increase the efficiency of the classifier, the characteristics of the logistics safety labels were extracted through a convolutional network and used as training and test data. As a result, we observed the performance of the ensemble classifier having Precision 0.9675, Recall 0.9575, and F1-score 0.9625. In addition, in order to solve the problem of performance degradation of the classifier depending on the background in which the box is photographed, a pipeline was designed to detect only the box without background and caution signs.
목차
Ⅰ. 서 론
Ⅱ. 문헌연구
Ⅲ. 연구설계
Ⅳ. 연구결과
Ⅴ. 시사점과 한계점
참고문헌
키워드
해당간행물 수록 논문
- A Study on the Utilization of Existing Innovation Information to the Improvement of Korea’s National Innovation System (NIS)
- VECM을 이용한 화물자동차 운임 결정요인의 실증 분석
- 동남권 집배송 물류수요 해소를 위한 산업단지의 활용 다각화 방안
- 서울시 음식물류폐기물의 날씨 변화에 따른 발생요인 분석과 효율적 처리 방향
- 정기선 해운기업 선박투자 행태분석에 관한 실증연구
- 가상 인플루언서 ‘로지(Rozy)’: 과연 모든 소비자들에게 어필할 수 있을 것인가?
- 인천∼제주 해상물류 활성화 방안에 대한 연구
- T-commerce 산업에 대한 의미연결망 분석
- 합성곱 신경망과 머신러닝을 활용한 화물운반 주의 표식 인식
참고문헌
관련논문
최근 이용한 논문
교보eBook 첫 방문을 환영 합니다!
신규가입 혜택 지급이 완료 되었습니다.
바로 사용 가능한 교보e캐시 1,000원 (유효기간 7일)
지금 바로 교보eBook의 다양한 콘텐츠를 이용해 보세요!
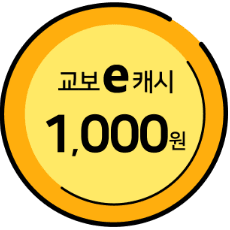