학술논문
Machine learning-based prediction of depression levels: developing a model for male and female senior citizens
이용수 164
- 영문명
- 머신러닝기반 남⋅녀 노인의 우울 수준 예측 및 분석
- 발행기관
- 연세대학교 사회복지연구소
- 저자명
- 홍기혜(KiHye Hong)
- 간행물 정보
- 『한국사회복지조사연구』70권, 145~172쪽, 전체 28쪽
- 주제분류
- 사회과학 > 사회복지학
- 파일형태
- 발행일자
- 2021.09.30
6,160원
구매일시로부터 72시간 이내에 다운로드 가능합니다.
이 학술논문 정보는 (주)교보문고와 각 발행기관 사이에 저작물 이용 계약이 체결된 것으로, 교보문고를 통해 제공되고 있습니다.
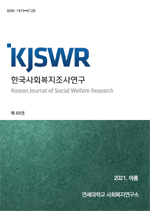
국문 초록
본 연구는 머신러닝 기법을 활용하여 우울 척도의 측정 없이 개별 노인의 우울 수준을 예측할 수 있는 모형 개발을 목적으로 한다. 아울러 예측에 기여하는 요인들의 특성을 성별로 분석하여 노인의 우울 수준을 줄이거나 예방하기 위한 방향을 제시하고자 한다. 실증분석에는 노인실태조사 자료를 활용하였으며 최종 연구대상자는 남성노인 12,544명과 여성노인 18,425명이다. 선행연구에서 노인의 우울에 영향을 미치는 것으로 보고한 변수에 대해 스트레스-대처 이론에 근거하여 56개의 요인을 설명변수로 취하고, 그래디언트 부스팅 머신러닝 알고리즘을 활용하여 예측 모형을 추정한 후, 예측 요인을 성별로 분석하였다. 예측 모형의 성능은 6개의 평가 지표로 남성노인과 여성노인에 대해 각각 평가되었다: 정분류율 76.0%과 73.9%, 민감도 60.7%와 73.3%, 특이도 85.3%과 74.4%, 정밀도 71.5%와 73.3%, F1-점수 65.7%와 73.6%, ROC-AUC-점수 82.7%와 82.1%. 건강 관련 요인과 소소한 사회적 활동은 노인의 우울을 예측하는데 중요한 요인이었으며 주목할만한 성별의 차이가 나타난 요인은 배우자와의 관계와 운동이었다. 본 연구는 노인과 가까운 사람이면 쉽게 알 수 있는 정보를 활용하여 노인의 우울 수준을 예측할 수 있는 모형을 개발했다는 점에 의의가 있다. 이는 사회복지 영역에서 우울의 위험에 노출되어있는 노인을 식별하는 것에 활용될 수 있을 것이다. 또한 본 연구는 56개의 설명변수를 분석하여 노인의 우울 예방에 통합적인 관점을 지원하고 성별에 따라 구별되거나 공통되는 근거를 제공하였다는 점에도 의의가 있다.
영문 초록
This study aims to develop a predictive model for individuals’ depression levels without using depression assessment scales. It will instead use a gradient boosting machine learning algorithm. This study will also analyze the predictive factors of depression by gender and suggests directions of intervention for depression in senior citizens. Data from the ‘Korean National Survey on the Elderly’ were used in this study. The participants were 12,544 elderly males and 18,425 elderly females. This study set 56 factors as explanatory variables based on stress-coping theory for the variables verified in previous studies, estimated a predictive model, and analyzed predictors by gender. The model performance of elderly males and females was evaluated, respectively, using six classification performance metrics: Accuracy was 76.0% and 73.9%. Recall was 60.7% and 73.3%. Specificity was 85.3% and 74.4%. Precision was 71.5% and 73.3. F1-score was 65.7% and 73.6%. ROC-AUC-score was 82.7% and 82.1%. Day-to-day health and small social activities were important in developing a predictive model for individuals’ depression levels. The predictors that have shown notable gender differences were marital relationships and exercise. This study demonstrated that it could be possible to predict geriatric depression with the factors that were recognizable to people close to the elderly. This predictive model can be used to identifying at-risk elderly individuals in the social welfare sector. This study is also meaningful in that 56 factors contributing to the prediction of depression levels were analyzed by gender. This supports an integrated perspective and provides gender-specific and gender-common evidence to prevent or reduce depression among senior citizens.
목차
I. Introduction
II. Theoretical and Empirical Framework
III. Method
IV. Results
V. Discussion
키워드
해당간행물 수록 논문
- A Study on Emotional Labor, Depression, and Anxiety Among Social Workers: Moderating Effect Analysis on the Availability of Flexible Work Arrangements
- 아동보호전담요원의 가정외보호 및 원가정복귀와 관련된 태도 연구
- 다문화 접촉경험에 따른 다문화수용성
- 생존분석을 활용한 발달장애인의 고용유지 영향 요인 분석
- Machine learning-based prediction of depression levels: developing a model for male and female senior citizens
- 농촌 1인 가구의 삶의 만족도 영향요인
- A Study on the Types and Characteristics of Welfare Attitudes toward Social Service Policy
- An Exploration on the Prevalence of Inter-generational Co-incidence of Prosocial Behavior in Korea: Charity Begins at Home?
참고문헌
교보eBook 첫 방문을 환영 합니다!
신규가입 혜택 지급이 완료 되었습니다.
바로 사용 가능한 교보e캐시 1,000원 (유효기간 7일)
지금 바로 교보eBook의 다양한 콘텐츠를 이용해 보세요!
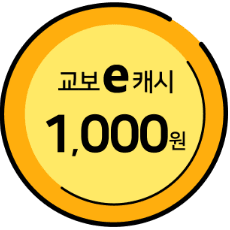