학술논문
데이터 클러스터링을 위한 혼합 시뮬레이티드 어닐링
이용수 51
- 영문명
- Hybrid Simulated Annealing for Data Clustering
- 발행기관
- 한국산업경영시스템학회
- 저자명
- 김성수(Sung-Soo Kim) 백준영(Jun-Young Baek) 강범수(Beom-Soo Kang)
- 간행물 정보
- 『산업경영시스템학회지』제40권 제2호, 92~98쪽, 전체 7쪽
- 주제분류
- 경제경영 > 경영학
- 파일형태
- 발행일자
- 2017.06.30
4,000원
구매일시로부터 72시간 이내에 다운로드 가능합니다.
이 학술논문 정보는 (주)교보문고와 각 발행기관 사이에 저작물 이용 계약이 체결된 것으로, 교보문고를 통해 제공되고 있습니다.
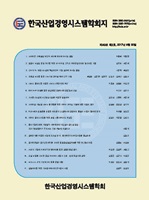
국문 초록
영문 초록
Data clustering determines a group of patterns using similarity measure in a dataset and is one of the most important and difficult technique in data mining. Clustering can be formally considered as a particular kind of NP-hard grouping problem. K-means algorithm which is popular and efficient, is sensitive for initialization and has the possibility to be stuck in local optimum because of hill climbing clustering method. This method is also not computationally feasible in practice, especially for large datasets and large number of clusters. Therefore, we need a robust and efficient clustering algorithm to find the global optimum (not local optimum) especially when much data is collected from many IoT (Internet of Things) devices in these days. The objective of this paper is to propose new Hybrid Simulated Annealing (HSA) which is combined simulated annealing with K-means for non-hierarchical clustering of big data. Simulated annealing (SA) is useful for diversified search in large search space and K-means is useful for converged search in predetermined search space. Our proposed method can balance the intensification and diversification to find the global optimal solution in big data clustering. The performance of HSA is validated using Iris, Wine, Glass, and Vowel UCI machine learning repository datasets comparing to previous studies by experiment and analysis. Our proposed KSAK (K-means+SA+K-means) and SAK (SA+K-means) are better than KSA(K-means+SA), SA, and K-means in our simulations. Our method has significantly improved accuracy and efficiency to find the global optimal data clustering solution for complex, real time, and costly data mining process.
목차
1. 연구의 배경 및 목적
2. 데이터 클러스터링 문제
3. 혼합 시뮬레이티드 어닐링 데이터 클러스터링
4. 실험결과 및 분석
5. 결 론
Acknowledgements
References
해당간행물 수록 논문
- 손실 비용을 고려한 공정 파라미터 허용차 산출 :
- 스마트폰 구매결정 요인이 재구매 의도에 미치는 영향
- 수하물시스템의 사전할당지점을 이용한 병합제어
- 전사적 PL 대응시스템의 핵심요인이 기업 성과에 미치는 영향
- 공구유연성과 공구관련제약을 고려한 통합공정일정계획을 위한 유전알고리즘
- 사회적 기업의 자료포락분석(DEA)을 통한 경영효율성 평가
- 항공사 비정상 운항 복구를 위한 리-타이밍 전략과 개미군집최적화 알고리즘 적용
- DEA AHP 모형을 통한 철강유통산업에서의 공급업체 선정
- 비즈니스 규칙 기반의 3자 물류 운영 지원
- 정밀제조를 위한 기하공차에서의 윤곽공차 사용
- PLS-MGA 방법론을 활용한 제도론적 관점에서의 공공제도 품질과 사용자 행태의 분석
- 중소기업의 외부 기술협력 네트워크의 다양성과 생산성 향상 :
- 경로분석을 이용한 사업의 효과성 분석 :
- 데이터 클러스터링을 위한 혼합 시뮬레이티드 어닐링
- 효율성 비교를 통한 나노기술 분야별 R&D 전략 수립
- 서비스 중요도를 사용한 서비스나무분석의 개선
- 스마트홈 지능형 서비스 플랫폼을 위한 데이터 마이닝 기법에 대한 적합도 평가
참고문헌
교보eBook 첫 방문을 환영 합니다!
신규가입 혜택 지급이 완료 되었습니다.
바로 사용 가능한 교보e캐시 1,000원 (유효기간 7일)
지금 바로 교보eBook의 다양한 콘텐츠를 이용해 보세요!
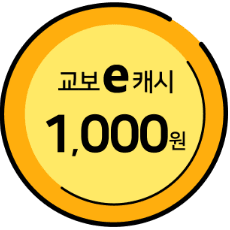