How Well can Machine Learning Models Classify Individuals with and without Non Specific Chronic Neck Pain based on Cervical Movements during Protraction and Retraction?
이용수 7
- 영문명
- 발행기관
- KEMA학회
- 저자명
- Ui jae Hwang Jun hee Kim
- 간행물 정보
- 『Journal of Musculoskeletal Science and Technology』제7권 제2호, 62~70쪽, 전체 9쪽
- 주제분류
- 의약학 > 재활의학
- 파일형태
- 발행일자
- 2023.12.31
구매일시로부터 72시간 이내에 다운로드 가능합니다.
이 학술논문 정보는 (주)교보문고와 각 발행기관 사이에 저작물 이용 계약이 체결된 것으로, 교보문고를 통해 제공되고 있습니다.

국문 초록
영문 초록
목차
해당간행물 수록 논문
- Effects of Rhythmik Program on Isokinetic Muscle Function and Static Ability
- Comparison of the Hip Flexor Strength in Subjects with and without Femoral Anterior Glide Type according to Manual Fixation of Femoral Head during Active Straight Leg Raise
- Changes in Muscle Activity of Hip Joint Muscles during Clam Exercise after Abdominal Draw in Exercise in Men with Weak Abdominal Muscles
- Analysis of the Correlation Coefficient between Experimental Device and F RAY for Skin Elasticity
- Effect of Hip Mobilization with Movement in Weight Bearing Position on Mobility of Hip Internal Rotation
- Validity and Reliability of Digital Navicular Drop Equipment in Young People with and without Pes Planus
- Machine Learning vs. Logistic Regression for Classifying Pressure Pain Hypersensitivity Based on Postural Analysis Data in Food Service Workers with Nonspecific Neck/Shoulder Myofascial Pain
- How Well can Machine Learning Models Classify Individuals with and without Non Specific Chronic Neck Pain based on Cervical Movements during Protraction and Retraction?
- A Multi Layer Perceptron Neural Network for Predicting the Diagnosis of Osteoporosis in Women Using Physical Activity Factors
- Prevalence and Anatomical Distribution of Non Contact Musculoskeletal Pain among Elite Adolescent Cricket Fast Bowlers in Sri Lanka
참고문헌
관련논문
의약학 > 재활의학분야 BEST
- 중증정신질환자가 이용하는 지역사회 정신건강서비스에 대한 체계적 고찰
- 조현병 환자에게 적용한 그룹인지재활프로그램이 인지기능과 작업적 참여에 미치는 효과
- 정신건강분야에서의 ICF 관련 연구 동향
의약학 > 재활의학분야 NEW
- A Systematic Review on the Management of Cortical Visual Impairment
- 2D Single-legged Dynamic Knee Valgus assessments Methods: Evaluating Risk Factor for Internal Derangement of the Knee; Literature Review
- The Effects of Training with Immersive Virtual Reality Devices on Balance, Walking and Confidence in Chronic Stroke Patients
최근 이용한 논문
신규가입 혜택 지급이 완료 되었습니다.
바로 사용 가능한 교보e캐시 1,000원 (유효기간 7일)
지금 바로 교보eBook의 다양한 콘텐츠를 이용해 보세요!
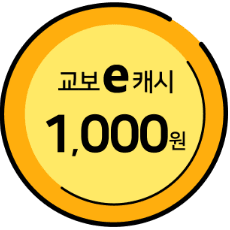