학술논문
종단자료의 속성을 고려한 머신러닝 기법 비교 연구: MERF와 glmmLasso를 중심으로
이용수 0
- 영문명
- A Comparison Study of Machine Learning Methods Considering the Structure of Longitudinal Data: Focusing on MERF and glmmLasso
- 발행기관
- 한국교육평가학회
- 저자명
- 정혜원(Hyewon Chung) 장은아(Eunah Jang)
- 간행물 정보
- 『교육평가연구』제38권 제1호, 209~241쪽, 전체 33쪽
- 주제분류
- 사회과학 > 교육학
- 파일형태
- 발행일자
- 2025.03.31
6,760원
구매일시로부터 72시간 이내에 다운로드 가능합니다.
이 학술논문 정보는 (주)교보문고와 각 발행기관 사이에 저작물 이용 계약이 체결된 것으로, 교보문고를 통해 제공되고 있습니다.
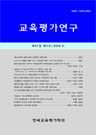
국문 초록
본 연구는 종단자료의 속성을 반영할 수 있는 머신러닝 기법인 MERF(mixed-effects random forest)와 glmmLasso(generalized linear mixed models with Lasso regularization)의 예측성능 및 변인 선택의 정확성을 비교하기 위해 수행되었다. 이를 위해 예측변인 수(25, 50, 100), 반복측정횟수(3, 4, 6), 사례 수(500, 1,000, 2,000, 4,000) 조건을 반영한 모의자료를 생성하고, MERF와 glmmLasso를 적용하여 평균제곱근오차와 변인 선택의 정확률 및 오류율, 상대적 편의 및 편의를 비교하였다. 모의실험 결과, 첫째, 본 연구에서 고려한 모든 모의실험 조건에서 glmmLasso의 예측성능이 MERF보다 우수한 것으로 나타났다. 둘째, 예측변인 수가 많을수록, 사례 수가 적을수록 MERF의 평균제곱근오차가 증가하여 예측성능이 저하되는 경향을 보였다. 반면, glmmLasso의 평균제곱근오차는 모의실험 조건에 따라 뚜렷한 경향 없이 비교적 안정적으로 추정되었다. 셋째, MERF와 glmmLasso를 적용했을 때, 사례 수가 적은 조건에서는 반복측정횟수가 증가할수록 평균제곱근오차가 높아졌다. 그러나 glmmLasso 적용 시 사례 수가 500인 일부 조건에서 상대적 편의가 나타났지만, 일정 사례 수 이상에서는 반복측정횟수 조건에 따른 평균제곱근오차에 뚜렷한 경향이 나타나지 않았다. 넷째, MERF 적용 시, 예측변인 수와 반복측정횟수가 작을수록, 사례 수가 많을수록 변인 선택의 정확률이 증가하는 것으로 나타났다. 이상의 결과를 토대로, 종단자료에 두 모형을 적용 시 고려해야 할 사항과 후속 연구를 위한 제언을 제시하였다.
영문 초록
The purpose of this study is to compare the predictive performance and variable selection accuracy of two machine learning methods—MERF and glmmLasso—that can account for the structure of longitudinal data. To achieve this, a simulation study was conducted with conditions varying in the number of predictors (25, 50, 100), number of repeated measurements (3, 4, 6), and sample sizes (500, 1,000, 2,000, 4,000). The performance of MERF and glmmLasso was evaluated using root mean squared error (RMSE), variable selection accuracy rate, error rate, relative bias, and bias. The results are as follows. First, glmmLasso consistently outperformed MERF in predictive performance across all conditions investigated under the current study. Second, MERF showed increasing RMSE with more predictors and smaller sample sizes, whereas glmmLasso maintained stable RMSE. Third, for both methods, RMSE increased with the number of repeated measurements when the sample size was relatively small. Additionally, in glmmLasso, relative bias was observed under some conditions when the sample size was 500. However, with a sufficiently large sample size, RMSE differences across the different numbers of repeated measurements were minimal. Fourth, in MERF, variable selection accuracy rate improved with fewer predictors, fewer numbers of repeated measurements, and a larger sample size. Based on these findings, practical guidelines for applying MERF and glmmLasso methods while considering the structure of longitudinal data and suggestions for future research were provided.
목차
Ⅰ. 서론
Ⅱ. 이론적 배경
Ⅲ. 연구방법
Ⅳ. 연구결과
Ⅴ. 논의 및 결론
참고문헌
해당간행물 수록 논문
- 척도 검사 설계를 적용한 수직척도화
- 고등학생의 진로적응력 주요 예측변수 탐색: XGBoost 및 SHAP 적용
- 초등학교 수학 기초학력 진단검사 문항난이도와 문항변별도에 미치는 요인 탐색 및 예측 모형 비교
- 전공자율선택제도 기반의 트랙 간 관계 및 키워드 간 결합도 가중네트워크 분석: 교육과정 프로파일링 기법(Curriculum Profiling Method)을 활용하여
- 사교육 참여가 수학 정의적 태도의 잠재계층 및 전이에 미치는 영향: 잠재계층 MIMIC 모형과 잠재전이분석을 활용하여
- IRT 문항 모수에 대한 베이지안 최빈 추정량의 점근 표준오차 추정 방법: 2PL/3PL 모형에의 적용
- 국내 교육프로그램 평가의 연구동향 분석
- 온라인 학습 데이터를 활용한 지식 추적 연구: 학생 능력 분포 차이가 모델의 성능에 미치는 영향을 중심으로
- 종단자료의 속성을 고려한 머신러닝 기법 비교 연구: MERF와 glmmLasso를 중심으로
참고문헌
교보eBook 첫 방문을 환영 합니다!
신규가입 혜택 지급이 완료 되었습니다.
바로 사용 가능한 교보e캐시 1,000원 (유효기간 7일)
지금 바로 교보eBook의 다양한 콘텐츠를 이용해 보세요!
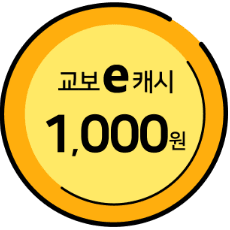