학술논문
온라인 학습 데이터를 활용한 지식 추적 연구: 학생 능력 분포 차이가 모델의 성능에 미치는 영향을 중심으로
이용수 0
- 영문명
- Knowledge Tracing with Online Learning Data: Investigating the Impact of Differences in Student Ability Distribution on Model Performance
- 발행기관
- 한국교육평가학회
- 저자명
- 권연하(Yeon Ha Kwon) 이현숙(Hyun Sook Yi)
- 간행물 정보
- 『교육평가연구』제38권 제1호, 83~113쪽, 전체 31쪽
- 주제분류
- 사회과학 > 교육학
- 파일형태
- 발행일자
- 2025.03.31
6,520원
구매일시로부터 72시간 이내에 다운로드 가능합니다.
이 학술논문 정보는 (주)교보문고와 각 발행기관 사이에 저작물 이용 계약이 체결된 것으로, 교보문고를 통해 제공되고 있습니다.
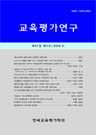
국문 초록
본 연구는 딥러닝 기반 지식 추적 모델에서 훈련 데이터와 실제 예측에 활용할 데이터 간의 학생 능력 분포 차이가 존재할 경우 모델의 성능에 미치는 영향을 분석하였다. 이를 위해 다양한 능력 분포를 가정하여 학습 이력 데이터를 생성하고 모의실험을 진행하였다. 또한, AI-Hub에서 제공하는 초·중학생 온라인 학습 데이터를 활용하여 훈련 데이터와 테스트 데이터 간의 분포 차이가 모델의 성능에 미치는 영향을 실증적으로 분석하였다. 주요 연구 결과는 다음과 같다. 첫째, 훈련 데이터와 실제 예측해야 하는 데이터의 분포 차이는 모델의 성능을 저하시키지만, 훈련 데이터가 과도하게 편향되지 않는다면 그 영향은 제한적이었다. 둘째, 학습 이력 데이터의 특성에 따라 지식 추적 모델별로 성능이 다르게 나타났다. 셋째, 동일한 데이터로 훈련된 지식 추적 모델의 경우에도 예측해야 하는 학생의 성취도 수준에 따라 모델의 예측 정확도에 차이가 나타났다. 넷째, 학생들의 문항 풀이 이력은 매우 다양한 양상으로 나타났으며, 특정 개념에 대해 반복적으로 학습하는 패턴도 관찰되었다. 이를 바탕으로 온라인 학습 환경의 특성을 고려한 지식 추적 모델 연구의 필요성과 정교한 지식 추적 모델 개발을 위한 시사점을 논의하였다.
영문 초록
This study aims to analyze the impact of distributional discrepancies between training and test data on the performance of deep-learning based knowledge tracing (KT) models. To achieve this, we conducted a simulation study by generating learning data with diverse distributions. Additionally, we utilized online learning data from elementary and middle school students to examine the characteristics of data collected from online learning environments and empirically assess the effect of distributional differences between training and test data on model performance. The main findings of this study are as follows. First, differences in the distribution between the training and test data led to a decline in model performance. However, if the training data was not excessively biased, the impact remained limited. Second, the performance of each KT model varied depending on the characteristics of the learning data. Third, the accuracy of KT models varied depending on the students’ achievement levels. Fourth, students exhibited a wide range of problem-solving response patterns, including repeated learning behaviors. Based on these findings, we discuss the necessity of developing KT models that account for the characteristics of online learning environments and provide insights for developing more sophisticated knowledge tracing models.
목차
Ⅰ. 서론
Ⅱ. 이론적 배경
Ⅲ. 연구방법
Ⅳ. 연구결과
Ⅴ. 결론 및 논의
참고문헌
키워드
해당간행물 수록 논문
- 척도 검사 설계를 적용한 수직척도화
- 고등학생의 진로적응력 주요 예측변수 탐색: XGBoost 및 SHAP 적용
- 초등학교 수학 기초학력 진단검사 문항난이도와 문항변별도에 미치는 요인 탐색 및 예측 모형 비교
- 전공자율선택제도 기반의 트랙 간 관계 및 키워드 간 결합도 가중네트워크 분석: 교육과정 프로파일링 기법(Curriculum Profiling Method)을 활용하여
- 사교육 참여가 수학 정의적 태도의 잠재계층 및 전이에 미치는 영향: 잠재계층 MIMIC 모형과 잠재전이분석을 활용하여
- IRT 문항 모수에 대한 베이지안 최빈 추정량의 점근 표준오차 추정 방법: 2PL/3PL 모형에의 적용
- 국내 교육프로그램 평가의 연구동향 분석
- 온라인 학습 데이터를 활용한 지식 추적 연구: 학생 능력 분포 차이가 모델의 성능에 미치는 영향을 중심으로
- 종단자료의 속성을 고려한 머신러닝 기법 비교 연구: MERF와 glmmLasso를 중심으로
참고문헌
교보eBook 첫 방문을 환영 합니다!
신규가입 혜택 지급이 완료 되었습니다.
바로 사용 가능한 교보e캐시 1,000원 (유효기간 7일)
지금 바로 교보eBook의 다양한 콘텐츠를 이용해 보세요!
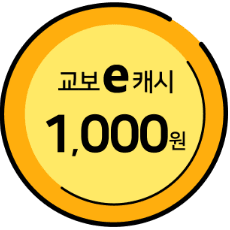