학술논문
딥러닝 기반 지하수위 예측 모델 개발에 있어 데이터 부족 문제 해결을 위한 전이학습의 응용
이용수 0
- 영문명
- Applying Transfer Learning to Improve the Performance of Deep Learning-based Groundwater Level Prediction Model with Insufficient Training Data
- 발행기관
- 대한자원환경지질학회
- 저자명
- 정지호(Jiho Jeong) 정진아(Jina Jeong)
- 간행물 정보
- 『자원환경지질』57권 5호, 551~562쪽, 전체 12쪽
- 주제분류
- 자연과학 > 지질학
- 파일형태
- 발행일자
- 2024.10.31
4,240원
구매일시로부터 72시간 이내에 다운로드 가능합니다.
이 학술논문 정보는 (주)교보문고와 각 발행기관 사이에 저작물 이용 계약이 체결된 것으로, 교보문고를 통해 제공되고 있습니다.
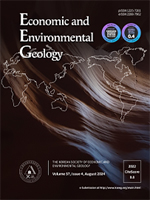
국문 초록
인공신경망과 같은 데이터 기반 모델을 활용하여 지하수위를 예측하기 위해서는 일반적으로 충분한 양의 데이터가 필요하다. 그러나 지하수위 모니터링 관정이 새로 개발되거나 유효하지 않은 데이터(예를 들어, 결측치 또는 이상치)가 다수 관찰되는 경우, 예측 모델을 적절히 학습하기 위한 데이터셋을 확보하기가 어려우며, 이는 예측 정확도의 저하로 이어진다. 본 연구에서는 이러한 학습 데이터 부족 문제를 해결하기 위해 전이 학습(Transfer Learning)을 기반으로 하는 방법을 제안하였다. 게이트 순환 유닛(Gated Recurrent Unit, GRU)을 예측을 위한 기본 데이터 기반 모델로 사용하였다. 전이학습 과정을 위한 GRU 기반 사전 학습 네트워크(Pretrained network)는 국내 전역의 89개 모니터링 지점에서 수집된 지하수위 및 이에 대응하는 강우 데이터를 활용하여 개발되었다. 그 후, 타겟 모니터링 관정에서 확보된 소량의 학습 데이터를 사용하여 사전 학습된 네트워크에 대한 미세 조정(Fine-tuning) 을 통해 최종 예측 모델을 개발하였다. 전이학습 알고리즘의 효과를 검증하기 위해 두 가지 서로 다른 지하수위 예측 모델을 비교 평가하였다: 1) 타겟 관정의 불충분한 데이터를 사용하여 학습된 GRU 기반 지하수위 예측 모델 및2) 전이학습 알고리즘을 기반으로 한 GRU 기반 예측 모델. 두 개의 다른 위치에 존재하는 관정에서 획득한 지하수위 자료에 대한비교 검증이 이루어졌으며, 전이학습 알고리즘을 활용한 모델이 다른 모델에 비해 우수한 성능을 보였다. 이를 통해 사용 가능한 학습 데이터의 양에 상관없이 전이학습 알고리즘이 지하수위 예측 모델 성능 향상에 크게 기여할 수 있음을 확인하였다.
영문 초록
Predicting groundwater levels with data-driven models like artificial neural networks typically requires a substantial amount of data. However, when groundwater monitoring wells are newly developed or when a significant portion of the data is invalid (for example, due to missing values or outliers), acquiring an adequate dataset for training prediction models becomes challenging, leading to diminished prediction accuracy. This study proposes a method based on transfer learning to address the issue of insufficient training data. The Gated Recurrent Unit (GRU) was used as the primary data-driven model for predictions. A GRU-based pretrained network for the transfer learning process was developed using groundwater level and corresponding rainfall data collected from 89 monitoring stations nationwide. Subsequently, this pretrained network was fine-tuned using a small amount of training data obtained from the target monitoring well to develop the final prediction model. To verify the effectiveness of the transfer learning algorithm, two different groundwater level prediction models were evaluated: 1) a GRU-based model trained with insufficient data from the target well, and 2) a GRU-based model utilizing the transfer learning algorithm. Comparative verification was conducted with groundwater level data obtained from wells at two different locations, where the model using the transfer learning algorithm demonstrated superior performance compared to the other. This study confirms that the transfer learning algorithm can significantly enhance the performance of groundwater level prediction models, irrespective of the amount of available training data.
목차
1. 서 론
2. 방법론
3. 이용 자료
4. 결과 및 토의
5. 결 론
사 사
References
키워드
해당간행물 수록 논문
- 인공위성 원격탐사 기반 메탄 배출 모니터링 기술 현황
- 공극 규모 반응성 운송 모델링의 연산 효율 향상을 위한 지화학 반응 대리 인공신경망 모형 개발
- Denoising Laplace-domain Seismic Wavefields using Deep Learning
- 폐광산 지반안정성 조사용 시추주상도의 분류 및 데이터베이스화를 위한 딥러닝 및 광학문자인식 기술의 적용
- 한국대지 XRD 실험자료 대상 k-평균 군집화 모델 적용성 분석
- 비소 오염토양의 효과적 정화를 위한 열수합성 마그네슘알루미늄-이중층수산화물/왕겨 하이드로차 나노복합체의 형성 및 이화학적 특성에 미치는 에이징 효과 규명
- 지질과학 분야 한국어 대규모 언어 모델 개발
- 광물자원 탐사를 위한 지구화학적 접근
- 딥러닝 기반 지하수위 예측 모델 개발에 있어 데이터 부족 문제 해결을 위한 전이학습의 응용
- 도심 습지의 생태계 서비스와 탄소 흡수 평가: 대전 갑천습지 사례 연구
- 몽골 울란바토르 복드칸 궁전 및 초이진 라마사원 벽돌과 기와의 재료학적 특성 및 고고과학적 의미
- 활석, 질석, 해포석, 사문석 등 석면함유 가능성이 있는 광물 함유 제품의 석면 분석 및 광물학적 특성
- Geochemical Characterisation of Magnesian Intrusives within High Grade Migmatite Gneiss Terrain: Insight from Plutons around Iwo Area, Southwest Nigeria
참고문헌
관련논문
자연과학 > 지질학분야 BEST
- 한국의 전기차 사용 후 배터리 재활용 및 재사용 효과 분석 연구
- 이산화탄소 포집/저장/활용 기술 특허 동향 분석
- 산불에 의한 지하수 토양 환경오염과 방사성 물질 분포 및 거동 영향 고찰
자연과학 > 지질학분야 NEW
- 인공위성 원격탐사 기반 메탄 배출 모니터링 기술 현황
- 공극 규모 반응성 운송 모델링의 연산 효율 향상을 위한 지화학 반응 대리 인공신경망 모형 개발
- Denoising Laplace-domain Seismic Wavefields using Deep Learning
최근 이용한 논문
교보eBook 첫 방문을 환영 합니다!
신규가입 혜택 지급이 완료 되었습니다.
바로 사용 가능한 교보e캐시 1,000원 (유효기간 7일)
지금 바로 교보eBook의 다양한 콘텐츠를 이용해 보세요!
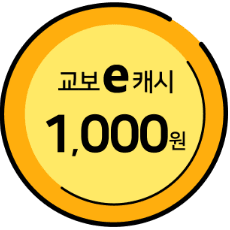