학술논문
로그라이크 게임에서의 머신러닝 에이전트에 관한 연구
이용수 33
- 영문명
- A Research on Machine Learning Agent in Rogue-like game
- 발행기관
- 한국컴퓨터게임학회
- 저자명
- 김세연(Se Yeon KIM) 김무집(Mu Jip KIM) 김석규(Seok-Kyoo KIM)
- 간행물 정보
- 『한국컴퓨터게임학회논문지』제37권 1호, 33~39쪽, 전체 7쪽
- 주제분류
- 공학 > 컴퓨터학
- 파일형태
- 발행일자
- 2024.03.30
4,000원
구매일시로부터 72시간 이내에 다운로드 가능합니다.
이 학술논문 정보는 (주)교보문고와 각 발행기관 사이에 저작물 이용 계약이 체결된 것으로, 교보문고를 통해 제공되고 있습니다.
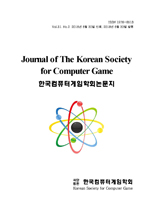
국문 초록
실제세계에서 데이터 수집의 비용과 한계를 고려할 때, 시뮬레이션 생성 환경은 데이터 생성과 다양한 시도에 있어 효율적인 대안이다. 이 연구에서는 Unity ML Agent를 로그라이크 장르에 적합한 강화학습 모델로 구현하였다. 간단한 게임에Agent를 이식하고, 이 Agent가 적을인식하고 대응하는 과정을 코드로 작성하였다. 초기 모델은 조준사격의 한계를 보였으나RayPerceptionSensor-Component2D를 통해 Agent의 센서 정보를 직접 제공함으로써, Agent가 적을 감지하고 조준 사격을 하는 능력을 관찰할 수 있었다. 결과적으로, 개선된 모델은 평균3.81배 향상된 성능을 보여주었으며, 이는 Unity ML Agent가 로그라이크 장르에서강화학습을 통한 데이터 수집이 가능함을 입증한다.
영문 초록
Collecting large amounts of data in the real world is expensive and has clear limitations. Simulation-generated environments, on the other hand, offer the opportunity to efficiently generate the necessary data and to try different things easily and quickly. In this research, we utilized one of the tools that addresses these challenges, by the Unity Machine Learning tool, to study an efficient automation model that responds to the characteristics of the rogue-like genre. For testing purposes, we implemented a simple game, implanted an agent into the main character of the game, and fed the agent with code to shoot and avoid hostile. The implemented ML Agent successfully recognized the hostile targets and responded by shooting and dodging them. However, instead of learning to prioritize the hostile targets over time by reinforcing itself and shooting the high-risk targets first, it consistently fired in only one of the 360-degree directions given to it at the beginning, which we didn’t expected, so we improved the code. By utilizing the RayPerceptionSensor-Component2D element to directly feed the agent's sensors with information about hostile targets, we found that the agent was able to utilize its ray sensor to detect them and make much more precise aimed shots. In fact, it outperformed the original model by an average of 3.81x, proving that Unity ML Agentcan collect data through reinforcement learning in the roguelike genre.
목차
1. Introduction
2. Related Research
3. Environment Implement
4. Conclusion
Reference
해당간행물 수록 논문
- 퍼즐 액션 게임 ‘MyEarth’의 구현 및 분석
- AI를 활용한 숏츠 애드버콘텐츠 이미지 생성에 관한 연구
- 엔드 게임 콘텐츠 디자인에 관한 고찰
- AHP를 활용한 게임 디자인 구성요소 모델 연구
- 퍼스 기호학을 통한 게임<원신>의 캐릭터 의상 디자인 특징 분석
- Media Facade 콘텐츠 제작 교육을 위한 Unity 3D Engine 기반 Projection Mapping Simulation
- 로그라이크 게임에서의 머신러닝 에이전트에 관한 연구
- 모바일 RPG 튜토리얼 구성요소 분석
- A method to incorporate Spherical Harmonic into URP for Real-Time Global Illumination in Unity Universal Render PipeLine
- Generative AI 도구와 플랫폼 디지털 아트 전공학생들에게 미치는 영향
- 실감 콘텐츠를 활용한 메타버스 플랫폼 전시공연 연구
- 메타버스 트랩 극복위한 게이미피케이션 다이나믹 ‘습관형성’ 모델
- 거리 측정의 일반화와 이를 이용한 Worley 노이즈 함수의 구현
- 미디어파사드의 이미지 재현 및 스토리텔링에 관한 연구
- 문화유산을 활용한 디지털콘텐츠 구현을 통한 보존과 계승
- 공간기반 콘텐츠를 기반한 확장현실 (XR) 콘텐츠 개발 연구
참고문헌
관련논문
공학 > 컴퓨터학분야 BEST
- 청소년들의 스마트폰 중독예방을 위한 이야기치료 집단상담 프로그램 개발
- 지도서비스를 이용한 위치 기반 관광 빅데이터의 시각화
- 광역 대중교통 접근성 향상이 관광 및 지역경제 활성화에 미치는 효과 분석
공학 > 컴퓨터학분야 NEW
- 스마트미디어저널 Vol13, No.11 목차
- YOLO 모델별 독성 해양 생물 탐지 성능 비교 및 스마트 수산 기술 적용 가능성 탐색
- 무인자동 양식어류 급이공급 시스템 개발 및 검증
최근 이용한 논문
교보eBook 첫 방문을 환영 합니다!
신규가입 혜택 지급이 완료 되었습니다.
바로 사용 가능한 교보e캐시 1,000원 (유효기간 7일)
지금 바로 교보eBook의 다양한 콘텐츠를 이용해 보세요!
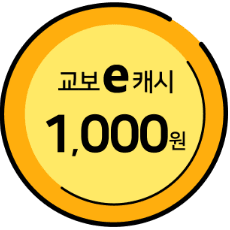