학술논문
이산 Wavelet 변환을 이용한 딥러닝 기반 잡음제거기
이용수 38
- 영문명
- Noise Canceler Based on Deep Learning Using Discrete Wavelet Transform
- 발행기관
- 한국전자통신학회
- 저자명
- 이행우(Haeng-Woo Lee)
- 간행물 정보
- 『한국전자통신학회 논문지』제18권 제6호, 1103~1108쪽, 전체 6쪽
- 주제분류
- 공학 > 전자/정보통신공학
- 파일형태
- 발행일자
- 2023.12.31
4,000원
구매일시로부터 72시간 이내에 다운로드 가능합니다.
이 학술논문 정보는 (주)교보문고와 각 발행기관 사이에 저작물 이용 계약이 체결된 것으로, 교보문고를 통해 제공되고 있습니다.
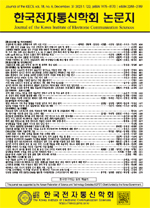
국문 초록
본 논문에서는 음향신호의 배경잡음을 감쇠하기 위한 새로운 알고리즘을 제안한다. 이 알고리즘은 이산 웨이블릿 변환(DWT: Discrete Wavelet Transform) 후 기존의 적응필터를 대신 FNN(: Full-connected Neural Network) 심층학습 알고리즘을 이용하여 잡음감쇠 성능을 개선하였다. 입력신호를 단시간 구간별로 웨이블릿 변환한 다음 1024-1024-512-neuron FNN 딥러닝 모델을 이용하여 잡음이 포함된 단일입력 음성신호로부터 잡음을 제거한다. 이는 시간영역 음성신호를 잡음특성이 잘 표현되도록 시간-주파수영역으로 변환하고 변환 파라미터에 대해 순수 음성신호의 변환 파라미터를 이용한 지도학습을 통하여 잡음환경에서 효과적으로 음성을 예측한다. 본 연구에서 제안한 잡음감쇠시스템의 성능을 검증하기 위하여 Tensorflow와 Keras 라이브러리를 사용한 시뮬레이션 프로그램을 작성하고 모의실험을 수행하였다. 실험 결과, 제안한 심층학습 알고리즘을 사용하면 기존의 적응필터를 사용하는 경우보다 30%, STFT(: Short-Time Fourier Transform) 변환을 사용하는 경우보다는 20%의 평균자승오차(MSE: Mean Square Error) 개선효과를 얻을 수 있었다.
영문 초록
In this paper, we propose a new algorithm for attenuating the background noises in acoustic signal. This algorithm improves the noise attenuation performance by using the FNN(: Full-connected Neural Network) deep learning algorithm instead of the existing adaptive filter after wavelet transform. After wavelet transforming the input signal for each short-time period, noise is removed from a single input audio signal containing noise by using a 1024-1024-512-neuron FNN deep learning model. This transforms the time-domain voice signal into the time-frequency domain so that the noise characteristics are well expressed, and effectively predicts voice in a noisy environment through supervised learning using the conversion parameter of the pure voice signal for the conversion parameter. In order to verify the performance of the noise reduction system proposed in this study, a simulation program using Tensorflow and Keras libraries was written and a simulation was performed. As a result of the experiment, the proposed deep learning algorithm improved Mean Square Error (MSE) by 30% compared to the case of using the existing adaptive filter and by 20% compared to the case of using the STFT(: Short-Time Fourier Transform) transform effect was obtained.
목차
Ⅰ. 서 론
Ⅱ. 이산 Wavelet 변환
Ⅲ. 잡음제거를 위한 변환영역 블록 딥러닝 알고리즘
Ⅳ. 모의실험 및 분석
Ⅴ. 결 론
References
키워드
해당간행물 수록 논문
- 한국전자통신학회 논문지 제18권 제6호 목차
- 고분해능 FMCW LiDAR 센서 구성을 위한 광대역 주파수변조 레이저 개발
- 기판온도에 의한 AZO 박막의 전기적 및 광학적 특성 변화
- 1,550 nm와 23.2 cm 파장의 도플러 측기 관측자료 비교
- SDN 환경에서 실시간 데이터 유입형태를 고려한 효율적인 부하분산 기법 연구
- 특허 출원 현황조사를 통한 안티드론 기술의 국내외 특허 동향 분석
- 증강현실 캐릭터 구현을 위한 AI기반 객체인식 연구
- 디지털 소외계층인 시니어를 대상으로 한 메타버스 키오스크 교육시스템
- 오일러와 라그랑주 관측방식의 연직 자료 비교
- SDN 환경에서 서버 상태를 고려한 단계적 가중치 기반의 부하 분산 기법 연구
- 전력 계통 신뢰도 개선을 위한 대표적인 한류기 유형 및 적용 효과 분석
- 개구 결합 급전 구조를 갖는 이중 선형편파 패치 안테나의 설계 및 제작
- 실시간 데이터 전송 환경에서의 셀룰러 오토마타 기반의 ROI 이미지 암호 알고리즘
- 지역 중첩 신뢰도가 적용된 샴 네트워크 기반 객체 추적 알고리즘
- 이산 Wavelet 변환을 이용한 딥러닝 기반 잡음제거기
- 랜덤 포레스트 기계 학습 방법을 이용한 넙치의 복수 증상 분석
- SHAP 분석 기반의 넙치 질병 분류 입력 파라미터 최적화
- 초 장단기 통합 태양광 발전량 예측 기법
- 2D 라이다를 이용한 실내 구조 스캐너 개발
- 두 가지 서로 다른 실내 복도에서 3, 6, 10, 17 GHz의 전파 특성 측정 및 비교 분석
- CCN 실시간 스트리밍 환경에서 효율적인 중간노드 이동성 관리 기법
- 한국, 미국, 중국의 애니메이션 기술의 특성 분석
- 정보보안 업무 스트레스의 완화
- CCN에서 실시간 콘텐츠 인기도 기반 캐시 정책
- PNP 모델을 이용한 리튬이온 배터리 잔존 수명 예측
- 화장실의 범죄위험도 평가를 위한 행동이론 기반 리스크 노드 판정 알고리즘
- IoT 기반의 캔/PET병 압착파쇄기 관리시스템 개발
- CNN-LSTM 기반의 자율주행 기술
- 산림자원 관리를 위한 항 재밍 기능을 보유한 무인항공기국 추적방법에 관한 연구
- 복합형 카메라 시스템을 이용한 자율주행 차량 플랫폼
- 감시정찰 센서 네트워크에서의 지진동센서 탐지 신호 세기를 이용한 표적 측위 방법
- 근적외선 분광법 기반 비침습식 혈당 수치 추정 알고리즘 연구
- 안전성 강화를 위한 ESP32-CAM을 활용한 시각장애인용 스마트지팡이에 대한 연구
- 딥러닝 모델을 이용한 전자 입찰에서의 예정가격 예측
- 1.29 GHz 펄스파로 산출한 대기경계층 고도
- 차세대 이동통신 및 스마트 모빌리티에 대한 위성항법시스템 최신 활용 동향
- LXC 환경을 이용한 한국형 합동 전술데이터링크체계의 소프트웨어 모의시험에 관한 연구
- 가상현실 공간에서의 ChatGPT 시스템을 활용한 인터페이스와 상호작용에 대한 연구
- 실시간 비지정 문화재 관리 및 도난 추적 시스템 개발을 위한 효율적인 디스크 버퍼 관리 정책 분석
- 그로브 제스처 센서를 활용한 모션 및 음성 인식 스마트 미러에 관한 연구
- 수중 치료용 고주파 전극 프로브 개발에 관한 연구
- 웨어러블 디바이스와 메타버스의 접점
- 증강현실을 이용한 건물의 수도관 관리 방안 연구
- 그래프 분류 기반 특징 선택을 활용한 작물 수확량 예측
- 메타버스 기반 시니어 맞춤형 인지 활동 지원 시스템
- 웹페이지에서의 상품 데이터 추출을 위한 동적, 정적 크롤링 비교 및 활용
참고문헌
관련논문
공학 > 전자/정보통신공학분야 BEST
- AI 시대 대학 교양교육에 필요한 디지털 리터러시 연구
- 인공지능의 역사, 분류 그리고 발전 방향에 관한 연구
- 생성형 인공지능(Generative AI)에 대한 지각된 가치와 지속이용의도 결정요인 탐색
공학 > 전자/정보통신공학분야 NEW
- 시간 영역 비교기를 사용한 PAM-4 ZQ 보정 회로 설계
- BLDC 모터 드라이빙 전용 IC를 적용한 정구공 발사기 모터 드라이버 설계 및 구현에 관한 연구
- 매입형 영구자석 동기전동기의 최적 속도 제어
최근 이용한 논문
교보eBook 첫 방문을 환영 합니다!
신규가입 혜택 지급이 완료 되었습니다.
바로 사용 가능한 교보e캐시 1,000원 (유효기간 7일)
지금 바로 교보eBook의 다양한 콘텐츠를 이용해 보세요!
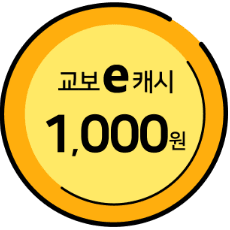