학술논문
EfficientNetV2 및 YOLOv5를 사용한 금속 표면 결함 검출 및 분류
이용수 64
- 영문명
- Metal Surface Defect Detection and Classification using EfficientNetV2 and YOLOv5
- 발행기관
- 한국전자통신학회
- 저자명
- Esanov Alibek 김강철(Kang-Chul Kim)
- 간행물 정보
- 『한국전자통신학회 논문지』제17권 제4호, 577~585쪽, 전체 9쪽
- 주제분류
- 공학 > 전자/정보통신공학
- 파일형태
- 발행일자
- 2022.08.30
4,000원
구매일시로부터 72시간 이내에 다운로드 가능합니다.
이 학술논문 정보는 (주)교보문고와 각 발행기관 사이에 저작물 이용 계약이 체결된 것으로, 교보문고를 통해 제공되고 있습니다.
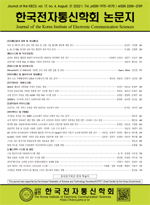
국문 초록
철강 표면 결함의 검출 및 분류는 철강 산업의 제품 품질 관리에 중요하다. 그러나 정확도가 낮고 속도가 느리기 때문에 기존 방식은 생산 라인에서 효과적으로 사용할 수 없다. 현재 널리 사용되는 알고리즘(딥러닝기반)은 정확도 문제가 있으며 아직 개발의 여지가 있다. 본 논문에서는 이미지 분류를 위한 EfficientNetV2와 물체 검출기로 YOLOv5를 결합한 강철 표면 결함 검출 방법을 제안한다. 이 모델의 장점은 훈련 시간이 짧고 정확도가 높다는 것이다. 먼저 EfficientNetV2 모델에 입력되는 이미지는 결함 클래스를 분류하고 결함이 있을 확률을 예측한다. 결함이 있을 확률이 0.3보다 작으면 알고리즘은 결함이 없는 샘플로 인식한다. 그렇지 않으면 샘플이 YOLOv5에 추가로 입력되어 금속 표면의 결함 감지 프로세스를 수행한다. 실험에 따르면 제안된 모델은 NEU 데이터 세트에서 98.3%의 정확도로 우수한 성능을 보였고, 동시에 평균 훈련 속도는 다른 모델보다 단축된 것으로 나타났다.
영문 초록
Detection and classification of steel surface defects are critical for product quality control in the steel industry. However, due to its low accuracy and slow speed, the traditional approach cannot be effectively used in a production line. The current, widely used algorithm (based on deep learning) has an accuracy problem, and there are still rooms for development. This paper proposes a method of steel surface defect detection combining EfficientNetV2 for image classification and YOLOv5 as an object detector. Shorter training time and high accuracy are advantages of this model. Firstly, the image input into EfficientNetV2 model classifies defect classes and predicts probability of having defects. If the probability of having a defect is less than 0.25, the algorithm directly recognizes that the sample has no defects. Otherwise, the samples are further input into YOLOv5 to accomplish the defect detection process on the metal surface. Experiments show that proposed model has good performance on the NEU dataset with an accuracy of 98.3%. Simultaneously, the average training speed is shorter than other models.
목차
Ⅰ. Introduction
Ⅱ. Methodology
Ⅲ. The Proposed method
Ⅳ. Experimental Results
Ⅴ. Conclusion
References
해당간행물 수록 논문
- 인공지능 기반 서비스 로봇을 위한 영상처리 프로세서 설계
- 200MW급 가스터빈 조속기 응답특성에 대한 연구
- 슬리브 발룬을 활용한 광대역 바이코니컬 안테나의 방사패턴 개선 연구
- 통합 측도를 사용한 주성분해석 부공간에서의 k-평균 군집화 방법
- SLAM을 이용한 카메라 기반의 실내 배송용 자율주행 차량 구현
- BESS를 활용한 전력계통 주파수 안정도 향상
- 중계기를 이용한 TOA 및 TDOA 기반의 위치추정 기법
- 의료 및 에너지 분야 전파 자원 관리 및 산업 기술 활성화 방안에 대한 연구
- 지수분포 특성을 갖는 NHPP 소프트웨어 신뢰성 모형의 성능 비교 분석
- 날씨 데이터 기반 코디추천 스마트옷장 시스템
- 형광 검출을 이용한 치석 진단 시스템 개발
- DO 센서와 라즈베리파이 카메라를 활용한 아두이노와 OpenCV기반의 이동식 녹조제거장치에 관한 연구
- 조직 내부의 정보보안 제언 행동 강화
- 연속주행시간을 고려한 운전자 위험운전행동의 정량화 방법
- 열차 고속 주행환경에서 LTE-R 무선통신시스템 성능 분석
- 한국형 합동 전술데이터링크체계의 소프트웨어 신뢰성 시험수행 방안에 관한 연구
- 스팀 멸균지시제 자동판독시스템 개발
- EfficientNetV2 및 YOLOv5를 사용한 금속 표면 결함 검출 및 분류
- 연자성 분말코어를 적용한 축방향 영구 자속형 전동기 설계
- 스마트 철도 통신기반 위험요인에 따른 정보보호 방안
- 가상현실 체험형 디바이스를 활용한 스마트 헬스케어 콘텐츠 개발
- 한국전자통신학회 논문지 제17권 제4호 목차
- 효율적인 부분 곱 감소를 이용한 고집적ㆍ저전력ㆍ고속 근사 곱셈기
- 소형 전기차 적용을 위한 AC/DC 복합 V2X 시스템 설계
- 6, 10, 17 GHz 반지하 실내 복도 환경의 전파 특성 분석
참고문헌
교보eBook 첫 방문을 환영 합니다!
신규가입 혜택 지급이 완료 되었습니다.
바로 사용 가능한 교보e캐시 1,000원 (유효기간 7일)
지금 바로 교보eBook의 다양한 콘텐츠를 이용해 보세요!
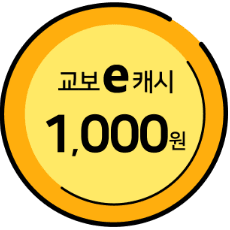