학술논문
딥러닝 기반 LSTM 모형을 이용한 저수지 수위자료 이상치 탐지
이용수 260
- 영문명
- Anomaly Detection in Reservoir Water Level Data Using the LSTM Model Based on Deep Learning
- 발행기관
- 한국방재학회
- 저자명
- 양미혜(Yang, Mi-Hye) 남원호(Nam, Won-Ho) 김한중(Kim, Han-Joong) 김태곤(Kim, Taegon) 신안국(Shin, An-Kook)
- 간행물 정보
- 『2. 한국방재학회 논문집』 21권1호, 71~81쪽, 전체 11쪽
- 주제분류
- 공학 > 기타공학
- 파일형태
- 발행일자
- 2021.02.28
4,120원
구매일시로부터 72시간 이내에 다운로드 가능합니다.
이 학술논문 정보는 (주)교보문고와 각 발행기관 사이에 저작물 이용 계약이 체결된 것으로, 교보문고를 통해 제공되고 있습니다.
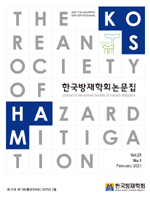
국문 초록
최근 국지성 가뭄 발생 및 집중 호우;평년 대비 강수량이 30% 이상 감소하는 등 기상 및 수문 현상이 변화하고 있다. 논벼 작물재배를 위한 용수를 공급하는 농업용 저수지의 경우 이상기후 발생으로 2013년부터 2017년까지 저수율이 0%에 도달한 저수지가 99개에 이르러 농업용수의 안정적 확보가 불확실해지고 있다. 농업환경의 변화와 기후변화에 대응하기 위해 농업용수 관리 정보화 및 과학화의 필요성이 증대되고 있으며;실시간으로 저수지 저수량과 농업용수 공급량을 파악하기 위해 자동 수위계측시설이 도입되었다. 농림축산식품부의 저수지 자동수위측정기 설치 및 운영지침에 따라 현재 농어촌공사 관리 저수지 1,734개소 및 수로부 1,880개소에 자동수위계가 설치되어 있으며;저수지와 수로에서 10분 간격으로 수위자료가 생성되고 있다. 농업용 저수지 수문자료의 공인지점은 2016년 6개소에서 2019년 49개소로 증가하고 있으며;데이터 품질 저하의 최소화 및 신뢰성 있는 수문자료 생성의 필요성이 증가함에 따라 농업용 저수지의 특성을 반영한 저수지 수위 오결측 데이터 보정 방안 및 수문 자료 품질관리 방안이 요구된다. 최근 인공신경망(Artificial Neural Network;ANN) 등의 모형을 이용하여 비선형적인 수문해석이 가능해짐에 따라 농업용 저수지의 수위 변화 및 강우-유출 현상을 기상;지형 등 영향 인자와 수위(또는 유출)와의 상관관계로부터 해석이 가능하다. 본 연구에서는 농업용수의 정량적 정보 관리를 위하여 시계열 자료의 학습에 효과적인 모형으로 다른 신경망과의 결합 등 다양한 분야에서 이용되고 있는 딥러닝(Deep Learning) 모형 중 하나인 LSTM (Long Short-Term Memory) 모형을 이용하여;저수지 수위 자료의 오⋅결측 자료에 대한 이상 탐지 알고리즘을 제시하고자 한다.
영문 초록
Weather and hydrological phenomena have been changing due to climate change as evidenced by localized torrential rainfall and precipitation falling by more than 30% compared to the annual average. From 2013 to 2017 the ninety-nine reservoirs reached a water storage rate of 0%;making a secure stable water supply for agriculture uncertain. There is an increased need for information regarding agricultural water management to respond to the changes in the agricultural environment and climate. Therefore;automatic water level measurement facilities have been introduced to determine the real-time reservoir storage capacity and agricultural water supply. According to the Ministry of Agriculture;Food and Rural Affairs guidelines for the installation and operation of water level measurement instruments;automatic water level facilities are currently installed at 1,734 reservoirs and 1,880 irrigation canals;with water level data generated at 10-minute intervals. The official recognition of hydrological water level data for agricultural reservoirs increased from six in 2016 to forty-nine in 2019. Anomaly detection algorithm methods for data regarding the agricultural reservoir level as well as quality control measures based on agricultural reservoir characteristics are required to minimize data quality degradation and generate reliable hydrological data over time. Though it was practically impossible to analyze the correlation between the water level or run-off and influential factors such as weather and terrain;recently a non-linear hydrological analysis has been possible using models such as Artificial Neural Networks (ANNs). This study aims to present an anomaly detection algorithm for reservoir level data using deep learning based LSTM (Long Short-Term Memory) models;in combination with other neural networks for managing quantitative information of agricultural water supply.
목차
Ⅰ. 서론
Ⅱ. 연구방법
Ⅲ. 결과 및 고찰
Ⅳ. 결론
키워드
해당간행물 수록 논문
- 뒷채움재의 N치 및 가속도 크기에 따른 안벽구조물의 동적거동
- 국내 원자력 발전소 부지의 바람 특성에 관한 연구
- 지반의 지지조건에 따른 모형 교각의 고유진동수
- 다층퍼셉트론과 합성곱 신경망에 기반한 지진 지반응답해석
- 동절기 철도 터널의 온도 분포에 관한 연구
- 원형 RC 교각기둥의 내진설계
- 피난 시뮬레이션을 통한 아파트 피난 안전성 및 소요시간에 관한 연구
- 화재 시 고층건축물의 연돌효과에 따른 압력차 분석에 관한 연구
- 포항 지진이 지역경제에 미치는 공간경제적 파급효과 분석
- Trichlorosilane 누출사고 시 물 반응성을 고려한 피해영향범위 산정식 도출방안 연구
- 종합병원 공백이 119 구급서비스에 미치는 영향
- 방재분야 표준품셈 대비 재해영향평가등의 협의 대행업무 계약금액 비교 분석: 협의 대상 규모 및 협의 주체별 분류를 중심으로
- 딥러닝 기반 LSTM 모형을 이용한 저수지 수위자료 이상치 탐지
- 폭염사상의 비정상성 빈도해석 및 불확실성 분석
- 토사비탈면 붕괴에 대한 계측관리기준 제안: 변위를 기준으로
- 기존 무도상 판형교 하부기초 지지력 및 근입 깊이 현장평가
- 계층적 분석을 통한 항공기 격납고 소화시스템의 화재위험 요인별 가중치 산정
- 화재의 영향을 받는 철근콘크리트 슬래브의 하중비에 따른 내화성능 및 깊이별 온도분포 평가
- 안전의식 개념 및 측정범위 연구
- 화재성상 그래프를 이용한 필요내화시간 산정에 관한 연구
- 전문가 설문조사를 통한 자연재난 위험도분석 평가지표 활용 가능성에 대한 검토
- 위성영상기반 증발산량 및 토양유효수분을 활용한 밭가뭄 평가
- 시각장애인의 피난안전 확보를 위한 국내 Barrier-free제도 및 대응매뉴얼 개선방안에 관한 연구
- 부산시 응급의료서비스 접근성의 지역 간 불균형 및 사회적 형평성에 대한 연구
- 대규모 농업용저수지의 농업용수 공급 운영 관리에 따른 이수안전도 평가
- 지반의 세립분 함유량 및 네트형 사방댐 격자크기가 토석류 거동에 미치는 영향
- Cone Calorimeter를 활용한 천장재의 연소특성에 관한 연구
- GPU 가속화를 이용한 수심적분형 방정식 기반 이송확산 수치모형 개발
- 건축물 화재통계자료를 활용한 스프링클러 작동확률 분석에 관한 연구
- 지점부 하부플랜지에 고강도 콘크리트를 합성한 강 박스거더교의 정적 특성 분석
- 제강슬래그 미분말의 치환율에 따른 콘크리트의 공극 구조 및 내구성 평가
참고문헌
교보eBook 첫 방문을 환영 합니다!
신규가입 혜택 지급이 완료 되었습니다.
바로 사용 가능한 교보e캐시 1,000원 (유효기간 7일)
지금 바로 교보eBook의 다양한 콘텐츠를 이용해 보세요!
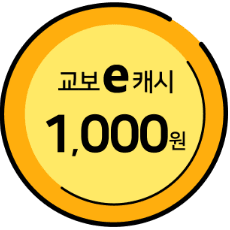