학술논문
인공신경망(ANN)을 이용한 옵션가격결정 및 딥헤징 (DH)에 대한 SNN 및 RNN 결과의 비교·분석
이용수 88
- 영문명
- Comparison and Analysis of SNN and RNN Results for Option Pricing and Deep Hedging Using Artificial Neural Networks (ANN)
- 발행기관
- 글로벌경영학회
- 저자명
- 이홍재 김태석
- 간행물 정보
- 『글로벌경영학회지』제20권 제5호, 146~178쪽, 전체 33쪽
- 주제분류
- 경제경영 > 경영학
- 파일형태
- 발행일자
- 2023.10.30
6,760원
구매일시로부터 72시간 이내에 다운로드 가능합니다.
이 학술논문 정보는 (주)교보문고와 각 발행기관 사이에 저작물 이용 계약이 체결된 것으로, 교보문고를 통해 제공되고 있습니다.
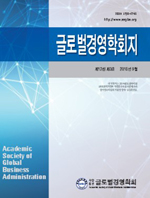
국문 초록
본 연구는 전통적인 Black-Scholes 옵션가격결정모형에 대한 기초자산의 다이내믹스(dynamics) 고정의 가정과 시장마찰 등 시장의 한계점을 극복하기 위한 대안적 방법을 제시하는 데 있다. 이때 실증분석 데이터는 2021년 5월 3일의 KOSPI200 지수가격 420.36(ATM)과 415.36(OTM) 및 425.36(ITM)을 이용하여 분석하였으며, 암묵적 변동성(implied volatility)은 2020년 5월 4일부터 2021년 5월 3일까지 KOSPI200데이터를 이용하여 계산되었다.
본 논문의 연구 방법은 인공 신경망 모형인 SNN(simple neural network)과 RNN(recurrent neural network)을 분석모형으로 하였으며, 콜옵션 매도 포트폴리오의 헤징모형은 gradient descent optimizer로 Adam을 사용하였다.
본 논문의 연구 결과는 다음과 같다. ATM 콜 옵션가격은 BS 모형이 10.245, 위험중립(risk neutral)모형이 10.268, SNN-DH 모형이 11.834, 그리고 RNN-DH 모형이 11.882로 나타났다. 따라서 각 분석 모형에 따른 콜옵션 가격은 근소한 차이를 나타냈다. 이때 딥헤징 분석 데이터 표본은 Monte Carlo Simulation에 의해 생성된 training 표본 100,000(1×)개 이며, testing 표본의 수는 training 표본의 20%(20,000개)를 이용하였다. 또한 SNN-DH 및 RNN-DH의 옵션가격 및 손익(P&L)은 기초에 선형으로 나타나며, 잔존만기가 길수록 SNN-DH 및 BS의 델타 값이 S자 곡선의 분포에 근접하고 만기 근처에서 두 모형의 델타가 0과 1에 밀집 분포하는 형태를 나타냈다. 콜 옵션 매도 포지션의 델타 헤징의 총손익은 SNN-DH가 -0.0027이고, RNN-DH가 -0.0061로서 헤지된 총손익이 0에 근사하는 것을 알 수 있었다.
본 연구의 시사점은 심층신경망 방법론이 알고리즘의 유효성과 로버스트 도구임을 고려하는 독립적 모형이라는 점에서 본 연구가 전통적인 옵션가격결정에 대한 다이내믹스 가정과 모형의 단순성 등에 기초한 헤징전략과는 차별화된다는 데 의의가 있다. 연구의 한계점으로는 거래비용이 없다는 가정하에서 분석된 모형이므로 추후 이를 고려한 연구가 수행되어야 할 것이다.
영문 초록
The purpose of this study is to present alternative methods to overcome market limitations such as the assumption of fixing the dynamics of underlying assets and market friction for the traditional Black-Scholes option pricing model. As for the research method of this paper, Adam is used as the gradient descent optimizer for the hedging model of the call option short portfolio using artificial neural network models SNN (simple neural network) and RNN (recurrent neural network) as analysis models, and deep neural network (deep neural network) is used as the hedging model. neural network) methodology was applied.
The research results of this paper are as follows. Regarding the ATM call option price, the BS model showed 10.245, the risk neutral model showed 10.268, the SNN-DH model showed 11.834, and the RNN-DH model showed 11.882. Therefore, it appears that there is a slight difference in the call option price according to each analysis model. At this time, the DH analysis data sample is 100,000 (1×) training samples generated by Monte Carlo Simulation, and the number of testing samples is the training sample. 20% (20,000 pieces) was used, and the option payoff function is lambda(), which is -max(, 0). In addition, the option price and P&L (P&L) of SNN-DH and RNN-DH appear linearly on the basis, and the longer the remaining maturity, the closer the delta value of SNN-DH and BS to the distribution of the S-curve, and the closer the expiration date, the closer the two models are. Delta is densely distributed between 0 and 1. As for the total loss of delta hedging of the short call option position, SNN-DH was -0.0027 and RNN-DH was -0.0061, indicating that the total hedged profit and loss was close to 0.
The implications of this study are to present DL techniques based on AI methods as an alternative way to overcome limitations such as fixing underlying asset dynamics and market friction of the traditional Black-Scholes model. In addition, it is an independent analysis model that considers valid robust tools by applying deep neural network algorithms for portfolio hedging problems. One limitation of the research is that it analyzed the model under the assumption of zero transaction costs, so future studies should consider this aspect.
목차
Ⅰ. 서 론
Ⅱ. 이론적 배경
Ⅲ. 연구 모형
Ⅳ. 실증 분석 결과 및 해석
Ⅴ. 결 론
참고문헌
키워드
해당간행물 수록 논문
참고문헌
관련논문
경제경영 > 경영학분야 BEST
더보기경제경영 > 경영학분야 NEW
- South Korea’s Strategic Directions in the Context of the US-China Trade War: An Application of the ABCD Model
- The Determinants of Entrepreneurial Inentions in Local Brand Fashion: A Perspective from Vietnamese Youth.
- Activation Plan of the Post-Construction Sales through a Perception Survey of Seoul Citizens and Experts
최근 이용한 논문
교보eBook 첫 방문을 환영 합니다!
신규가입 혜택 지급이 완료 되었습니다.
바로 사용 가능한 교보e캐시 1,000원 (유효기간 7일)
지금 바로 교보eBook의 다양한 콘텐츠를 이용해 보세요!
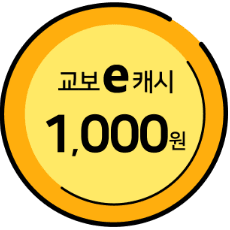