학술논문
TimeGAN을 활용한 트레이딩 알고리즘 선택
이용수 123
- 영문명
- Trading Algorithm Selection Using Time-Series Generative Adversarial Networks
- 발행기관
- 한국스마트미디어학회
- 저자명
- 이재윤(Jae Yoon Lee) 이주홍(Ju Hong Lee) 최범기(Bum Ghi Choi) 송재원(Jae Won Song)
- 간행물 정보
- 『스마트미디어저널』Vol11, No.1, 38~45쪽, 전체 8쪽
- 주제분류
- 공학 > 컴퓨터학
- 파일형태
- 발행일자
- 2022.02.28
4,000원
구매일시로부터 72시간 이내에 다운로드 가능합니다.
이 학술논문 정보는 (주)교보문고와 각 발행기관 사이에 저작물 이용 계약이 체결된 것으로, 교보문고를 통해 제공되고 있습니다.
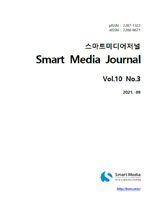
국문 초록
주식 시장에서 안정적으로 높은 수익을 얻기 위하여 많은 트레이딩 알고리즘에 대한 연구들이 이루어졌다. 트레이딩 알고리즘들이 미국 주식시장의 거래량에서 차지하는 비율은 80 프로가 넘을 정도로 많이 사용된다. 많은 연구에도 불구하고 항상 좋은 성능을 나타내는 트레이딩 알고리즘은 존재하지 않는다. 즉, 과거에 좋은 성능을 보이는 알고리즘이 미래에도 좋은 성능을 보인다는 보장이 없다. 그 이유는 주가에 영향을 주는 요인은 매우 많고, 미래의 불확실성도 존재하기 때문이다. 따라서 본 논문에서는 알고리즘들의 수익률에 대한 과거 기록을 바탕으로 미래의 수익률을 잘 예측하고 수익률도 높을 것으로 추정되는 알고리즘을 선택하는 TimeGAN을 활용한 모델을 제안한다. LSTM기법은 미래 시계열 데이터의 예측이 결정론적임에 반하여 TimeGAN은 확률적이다. TimeGAN의 확률적인 예측의 이점은 미래에 대한 불확실성을 반영하여 줄 수 있다는 점이다. 실험 결과로써, 본 논문에서 제안한 방법은 적은 변동성으로 높은 수익률을 달성하고, 여러 다수의 비교 알고리즘에 비해 우수한 결과를 보인다.
영문 초록
A lot of research is being going until this day in order to obtain stable profit in the stock market. Trading algorithms are widely used, accounting for over 80% of the trading volume of the US stock market. Despite a lot of research, there is no trading algorithm that always shows good performance. In other words, there is no guarantee that an algorithm that performed well in the past will perform well in the future. The reason is that there are many factors that affect the stock price and there are uncertainties about the future. Therefore, in this paper, we propose a model using TimeGAN that predicts future returns well and selects algorithms that are expected to have high returns based on past records of the returns of algorithms. We use TimeGAN becasue it is probabilistic, whereas LSTM method predicts future time series data is deterministic. The advantage of TimeGAN probabilistic prediction is that it can reflect uncertainty about the future. As an experimental result, the method proposed in this paper achieves a high return with little volatility and shows superior results compared to many comparison algorithms.
목차
Ⅰ. 서론
Ⅱ. 관련 연구
Ⅲ. 제안 아이디어
Ⅳ. 모델 구성
Ⅴ. 실험
Ⅵ. 결론
해당간행물 수록 논문
참고문헌
관련논문
공학 > 컴퓨터학분야 BEST
- 청소년들의 스마트폰 중독예방을 위한 이야기치료 집단상담 프로그램 개발
- 지도서비스를 이용한 위치 기반 관광 빅데이터의 시각화
- 틱톡의 숏폼 콘텐츠 특성이 관광지 이미지 및 방문의도에 미치는 영향
공학 > 컴퓨터학분야 NEW
- 착용형 증강현실 기반 체험형 콘텐츠 연구
- 스마트미디어저널 Vol13, No.4 목차
- Incorporating BERT-based NLP and Transformer for An Ensemble Model and its Application to Personal Credit Prediction
최근 이용한 논문
교보eBook 첫 방문을 환영 합니다!
신규가입 혜택 지급이 완료 되었습니다.
바로 사용 가능한 교보e캐시 1,000원 (유효기간 7일)
지금 바로 교보eBook의 다양한 콘텐츠를 이용해 보세요!
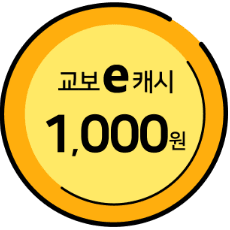