학술논문
심층강화학습을 이용한 Convolutional Network 기반 전산화단층영상 잡음 저감 기술 개발
이용수 49
- 영문명
- Development of Convolutional Network-based Denoising Technique using Deep Reinforcement Learning in Computed Tomography
- 발행기관
- 한국방사선학회
- 저자명
- 조정효(Jenonghyo Cho) 임도빈(Dobin Yim) 남기복(Kibok Nam) 이다혜(Dahye Lee) 이승완(Seungwan Lee)
- 간행물 정보
- 『한국방사선학회 논문지』 제14권 제7호, 991~1001쪽, 전체 11쪽
- 주제분류
- 공학 > 기타공학
- 파일형태
- 발행일자
- 2020.12.30
4,120원
구매일시로부터 72시간 이내에 다운로드 가능합니다.
이 학술논문 정보는 (주)교보문고와 각 발행기관 사이에 저작물 이용 계약이 체결된 것으로, 교보문고를 통해 제공되고 있습니다.
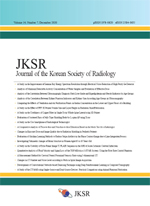
국문 초록
전산화단층영상 품질 개선을 위해 사용되는 지도학습 기반의 딥러닝 기술은 사전 학습을 위해 많은 양의 데이터를 필요로 하는 단점이 있다. 또한 지도학습 기반의 딥러닝 기술은 학습에 사용된 영상의 특징과 학습된 모델에 입력된 영상의 특징이 다른 경우 영상 내부 구조적 왜곡이 유발되는 한계점이 있다. 본 연구에서는 기존 지도학습 기반 딥러닝 기술의 단점을 보완하고 전산화단층영상의 잡음을 감소시킬 수 있는 심층강화학습 기반 영상화 모델을 개발하였다. 심층강화학습 기반 영상화 모델은 shared, value 및 policy 네트워크로 구성하였으며, 영상 잡음 특징 추출 및 모델의 성능 향상을 위해 합성곱, rectified linear unit(ReLU) 활성화 함수, dilation factor 및 게이트순환유닛을 사용하였다. 또한 기존 지도학습 기반 딥러닝 기술을 통해 획득한 영상의 영상품질 비교를 통해 본 연구에서 개발한 영상화 모델의 성능을 평가하였다. 연구결과 기존 기술에 비해 본 연구에서 개발한 영상화 모델 적용 시 전산화단층영상의 정량적 정확도는 큰 폭으로 향상, 잡음은 큰 폭으로 감소함을 확인하였다. 또한 영상화 모델 학습 시 사용한 영상과 구조적 특징이 다른 영상에 대해서도 잡음 감소 효과를 확인하였다. 따라서 본 연구에서 개발한 심층강화학습 기반 영상화 모델을 통해 전산화단층영상의 구조적 특징을 보전함과 동시에 잡음을 감소시킬 수 있다.
영문 초록
Supervised deep learning technologies for improving the image quality of computed tomography (CT) need a lot of training data. When input images have different characteristics with training images, the technologies cause structural distortion in output images. In this study, an imaging model based on the deep reinforcement learning (DRL) was developed for overcoming the drawbacks of the supervised deep learning technologies and reducing noise in CT images. The DRL model was consisted of shared, value and policy networks, and the networks included convolutional layers, rectified linear unit (ReLU), dilation factors and gate rotation unit (GRU) in order to extract noise features from CT images and improve the performance of the DRL model. Also, the quality of the CT images obtained by using the DRL model was compared to that obtained by using the supervised deep learning model. The results showed that the image accuracy for the DRL model was higher than that for the supervised deep learning model, and the image noise for the DRL model was smaller than that for the supervised deep learning model. Also, the DRL model reduced the noise of the CT images, which had different characteristics with training images. Therefore, the DRL model is able to reduce image noise as well as maintain the structural information of CT images.
목차
Ⅰ. INTRODUCTION
Ⅱ. MATERIAL AND METHODS
Ⅲ. RESULTS
Ⅳ. DISCUSSIONS
Ⅴ. CONCLUSIONS
Reference
키워드
해당간행물 수록 논문
- FFF방식의 3D프린터 노즐 크기와 층 높이가방사선 차폐체 제작에 미치는 영향에 관한 연구
- 건물 저층과 고층에서 환기와 공기정화 식물을 통한 라돈 농도의 비교
- 65세부터 85세 여성의 뇌 구조 부피 변화 조사
- 물 시료의 최소검출가능 농도 분석과 유효선량 평가
- 소아 환자에서 방사선 차폐체로 인한 피폭선량과 화질의 변화
- 3.0T MR system에서 TOF-MRA의 유체속도와 신호소실의 정량분석
- 방사선사의 스마트폰 이용에 관한 연구
- 단일조사 whole spine Lateral 검사에서3D 프린터로 제작한 구리 필터 유용성 연구
- 연령대별 초음파 진단 지방간 등급과 고지혈증 및 비만 지표 간의 상관관계 분석
- 3차원 컴퓨터단층촬영상을 이용한 신경공 협착률 측정방법
- 단일 선원 장치와 이중 선원 장치 비교를 이용한 전산화단층촬영 금속인공물 감소에 대한 연구
- 급성 허혈성 뇌경색 환자의 자기공명영상 검사 시 Echo Planar Image T2 FLAIR 기법의 유용성에 관한 연구
- 신장 초음파 검사에서 연령대에 따른신장 기능 지표와 신장 크기 간의 상관관계 분석
- 고순도 Ge 검출기의 전기적 노이즈 감소를 통한 감마선 에너지 스펙트럼의 분해능 향상에 관한 연구
- 방사선사면허 시험 대비 모의고사 중심으로 대면 교육과 비대면 교육비교 분석
- 나선형영상획득에서 Pitch에 따른 CT 감약계수와 잡음의 변화
- 선적분에 의한 위상차 영상의 줄무늬 아티팩트 감소를 위한 기계학습법에 대한 평가
- L-Spine X-선 촬영에서의 Jelly type 차폐체의 산란선 차폐평가
- 심층강화학습을 이용한 Convolutional Network 기반 전산화단층영상 잡음 저감 기술 개발
참고문헌
교보eBook 첫 방문을 환영 합니다!
신규가입 혜택 지급이 완료 되었습니다.
바로 사용 가능한 교보e캐시 1,000원 (유효기간 7일)
지금 바로 교보eBook의 다양한 콘텐츠를 이용해 보세요!
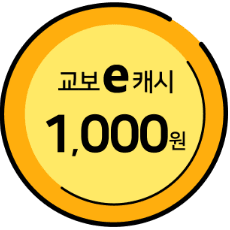