학술논문
대규모 외생 변수 및 Deep Neural Network 기반 금융 시장 예측 및 성능 향상
이용수 89
- 영문명
- Financial Market Prediction and Improving the Performance Based on Large-scale Exogenous Variables and Deep Neural Networks
- 발행기관
- 한국스마트미디어학회
- 저자명
- 천성길(Sung Gil Cheon) 이주홍(Ju Hong Lee) 최범기(Bum Ghi Choi) 송재원(Jae Won Song)
- 간행물 정보
- 『스마트미디어저널』Vol9, No.4, 26~35쪽, 전체 10쪽
- 주제분류
- 공학 > 컴퓨터학
- 파일형태
- 발행일자
- 2020.12.30
4,000원
구매일시로부터 72시간 이내에 다운로드 가능합니다.
이 학술논문 정보는 (주)교보문고와 각 발행기관 사이에 저작물 이용 계약이 체결된 것으로, 교보문고를 통해 제공되고 있습니다.
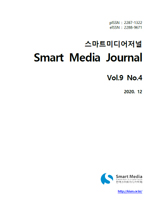
국문 초록
미래의 주가를 예측하기 위한 시도는 과거부터 꾸준히 연구되어왔다. 그러나 일반적인 시계열 데이터와 달리 금융 시계열 비정상성(non-stationarity)과 장기 의존성(long-term dependency), 비선형성(non-linearity) 등 예측을 하는 것에 있어서 여러 가지 방해 요인이 존재한다. 또한, 광범위한 데이터의 변수는 기존에 사람이 직접 선택하는 것에 한계가 있으며 모델이 변수를 자동으로 잘 추출할 수 있도록 하여야 한다. 본 논문에서는 비정상성 데이터를 정규화할 수 있는 슬라이딩 타임스텝 정규화(sliding time step normalization) 방법과 LSTM 형태의 오토인코더(AutoEncoder)를 사용하여 모든 변수로부터 압축된 변수로 미래 주가를 예측하는 방법, 기간을 나누어 전이 학습을 하는 이동 전이 학습(moving transfer learning)을 제안한다. 또한, 실험을 통하여 100개의 주요 금융 변수들만을 사용하는 것보다 뉴럴 네트워크를 통해서 가능한 많은 변수를 사용하였을 때 성능이 우수함을 보이며, 슬라이딩 타임스텝 정규화 방법을 사용하여 모든 구간에서 데이터의 비정상성에 대해 정규화를 수행함으로써 성능 향상에 효과적임을 보인다. 이동 전이 학습 방법은 스텝 별 테스트 구간에서 모델의 성능을 평가하고 전이학습을 함으로써 긴 테스트 구간에서 성능 향상에 효과적임을 보인다.
영문 초록
Attempts to predict future stock prices have been studied steadily since the past. However, unlike general time-series data, financial time-series data has various obstacles to making predictions such as non-stationarity, long-term dependence, and non-linearity. In addition, variables of a wide range of data have limitations in the selection by humans, and the model should be able to automatically extract variables well. In this paper, we propose a ‘sliding time step normalization’ method that can normalize non-stationary data and LSTM autoencoder to compress variables from all variables. and ‘moving transfer learning’, which divides periods and performs transfer learning. In addition, the experiment shows that the performance is superior when using as many variables as possible through the neural network rather than using only 100 major financial variables and by using sliding time step normalization to normalize the non-stationarity of data in all sections, it is shown to be effective in improving performance. moving transfer learning shows that it is effective in improving the performance in long test intervals by evaluating the performance of the model and performing transfer learning in the test interval for each step.
목차
Ⅰ. 서론
Ⅱ. 관련 연구
Ⅲ. 정규화, 전이학습, 변수추출방법 제안
Ⅳ. 예측 시스템
Ⅴ. 실험
Ⅵ. 결론
REFERENCES
키워드
해당간행물 수록 논문
- AR기반 교육용 콘텐츠분석을 위한 통계분석서비스 모형 설계
- 농작물을 위한 드론 분무 농약 살포의 3차원 분석에 관한 연구
- 네 자리 숫자 비밀번호 2차 조사 자료에 의한 국내 패스워드 재사용 추론 연구
- 대용량 멀티미디어 데이터 전송을 위한 효율적인 채널 검색 방법
- A Study on Applying the SRCNN Model and Bicubic Interpolation to Enhance Low-Resolution Weeds Images for Weeds Classification
- 서비스 플랫폼 기반 이중강화적용 블록체인 응용 거래모델 제안
- 인터넷 의료 애플리케이션 사용 행위에 영향을 미치는 요소에 관한 연구
- 모노펄스 추적 알고리즘 성능 향상을 위한 능동위상배열안테나 제어 기법
- PLN 성분 분석을 통한 전기장센서 기반 손동작신호 추출
- Conjoint Analysis of User Needs in Mobile Payment Interface Design
- IPA를 활용한 건축 설계단계 VR 적용가능업무 평가
- Hyper-parameter Optimization for Monte Carlo Tree Search using Self-play
- 자율주행 환경에서 이미지 객체 분할을 위한 강화된 DFCN 알고리즘 성능연구
- 대규모 외생 변수 및 Deep Neural Network 기반 금융 시장 예측 및 성능 향상
- 에너지 디지털 트윈을 위한 요구사항 분석 및 AAS 설계
- 구어체 말뭉치의 어휘 사용 특징 분석 및 감정 어휘 사전의 자동 구축
- 폐암환자 생존분석에 대한 TNM 병기 군집분석 평가
- 기계학습 알고리즘을 이용한 Curation 서비스 구현
- Fast Super-Resolution GAN 기반 자동차 번호판 검출 및 인식 성능 고도화 기법
- 토픽모델링을 통한 북한의 경제정책 동향 분석
- 딥러닝 기반 이미지 특징 추출 모델을 이용한 유사 디자인 검출에 대한 연구
- EF 센서기반 손동작 신호 감지 및 자동 프레임 추출
참고문헌
관련논문
공학 > 컴퓨터학분야 BEST
- 청소년들의 스마트폰 중독예방을 위한 이야기치료 집단상담 프로그램 개발
- 지도서비스를 이용한 위치 기반 관광 빅데이터의 시각화
- 틱톡의 숏폼 콘텐츠 특성이 관광지 이미지 및 방문의도에 미치는 영향
공학 > 컴퓨터학분야 NEW
더보기최근 이용한 논문
교보eBook 첫 방문을 환영 합니다!
신규가입 혜택 지급이 완료 되었습니다.
바로 사용 가능한 교보e캐시 1,000원 (유효기간 7일)
지금 바로 교보eBook의 다양한 콘텐츠를 이용해 보세요!
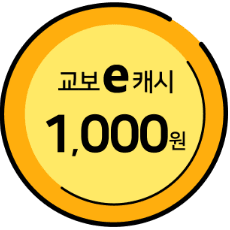