학술논문
데이터 구성에 따른 하천 조류 예측 딥러닝 모형 (TabPFN) 성능 비교
이용수 2
- 영문명
- Comparing the Performance of a Deep Learning Model (TabPFN) for Predicting River Algal Blooms with Varying Data Composition
- 발행기관
- 한국습지학회
- 저자명
- 양현석 박정수
- 간행물 정보
- 『한국습지학회지』제26권 제3호, 197~203쪽, 전체 7쪽
- 주제분류
- 자연과학 > 지질학
- 파일형태
- 발행일자
- 2024.08.31
4,000원
구매일시로부터 72시간 이내에 다운로드 가능합니다.
이 학술논문 정보는 (주)교보문고와 각 발행기관 사이에 저작물 이용 계약이 체결된 것으로, 교보문고를 통해 제공되고 있습니다.

국문 초록
하천에서 조류의 과다 발생은 취수원 관리 및 정수 처리에 악영향을 줄 수 있어 지속적인 관리가 필요하다. 본 연구에서는 딥러닝 알고리즘 중 작은 규모의 테이블 데이터에서도 상대적으로 우수한 성능을 보이는 것으로 알려진 tabular prior data fitted networks (TabPFN)을 사용하여 조류 발생 지표 중 하나인 chlorophyll-a (chl-a) 농도를 예측하는 다중 분류 모형을 구축하였다. 모형의 구축을 위해 부여지점 수질자동측정망에서 2014년 1월 1일부터 2022년 12월 31일까지 측정된 일일측정자료를 사용하였으며 입력 자료의 크기가 모형의 성능에 미치는 영향을 확인하기 위해 입력 자료의 평균값을 이용하여 1일, 3일, 6일, 12일의 측정 주기를 가진 입력 자료를 구성하였다. 각 모형의 성능을 비교한 결과 측정 주기가 길어져 입력 자료의 규모가 작은 경우에도 모형이 안정적인 성능을 보이는 것을 확인하였다. 각 모형의 macro average는 precision이 0.77, 0.76, 0.83, 0.84였으며, recall은 0.63, 0.65, 0.66, 0.74 F1-score는 0.67, 0.69, 0.71, 0.78로 분석되었다. Weighted average는 precision이 0.76, 0.77, 0.81, 0.84이며 recall은 0.76, 0.78, 0.81, 0.85 F1-score는 0.74, 0.77, 0.80, 0.84로 분석되었다. 본 연구에서는 TabPFN을 이용하여 구축한 chl-a예측 모형이 작은 규모의 입력 자료에서도 안정적인 성능을 보이는 것을 확인하여 모형구축에 필요한 입력 자료가 제한적인 현장에서의 적용 가능성을 확인하였다.
영문 초록
The algal blooms in rivers can negatively affect water source management and water treatment processes, necessitating continuous management. In this study, a multi-classification model was developed to predict the concentration of chlorophyll-a (chl-a), one of the key indicators of algal blooms, using Tabular Prior Fitted Networks (TabPFN), a novel deep learning algorithm known for its relatively superior performance on small tabular datasets. The model was developed using daily observation data collected at Buyeo water quality monitoring station from January 1, 2014, to December 31, 2022. The collected data were averaged to construct input data sets with measurement frequencies of 1 day, 3 days, 6 days, 12 days. The performance comparison of the four models, constructed with input data on observation frequencies of 1 day, 3 days, 6 days, and 12 days, showed that the model exhibits stable performance even when the measurement frequency is longer and the number of observations is smaller. The macro average for each model were analyzed as follows: Precision was 0.77, 0.76, 0.83, 0.84; Recall was 0.63, 0.65, 0.66, 0.74; F1-score was 0.67, 0.69, 0.71, 0.78. For the weighted average, Precision was 0.76, 0.77, 0.81, 0.84; Recall was 0.76, 0.78, 0.81, 0.85; F1-score was 0.74, 0.77, 0.80, 0.84. This study demonstrates that the chl-a prediction model constructed using TabPFN exhibits stable performance even with small-scale input data, verifying the feasibility of its application in fields where the input data required for model construction is limited.
목차
1. 서 론
2. 재료 및 실험방법
3. 결과 및 고찰
4. 결 론
References
키워드
해당간행물 수록 논문
- 현장조치 행동매뉴얼 기반의 e-SOP 시스템 구축 및 활용 방안 연구
- 국내외 주요 산불위험지수의 국내 적용성 평가: 태백산맥 동쪽 지역을 대상으로
- 머신러닝를 이용한 낙동강 본류 구간 수문-기상인자 조류 예보체계 연구
- 생태계 서비스 지불제 도입 후 조류 먹이원 분석 및 관리 방안: 고양 장항습지를 대상으로
- 자유수면을 갖는 2단 와류 수차의 성능에 관한 실험적 연구
- 전라남도 고흥군 우량 산림습원의 관속식물상 변화와 관리방안
- 내륙습지 총량관리제도의 고찰 및 도입 방안 제안
- 김포한강신도시 주변 논 습지에 도래하는 월동 조류의 서식처 보전대책
- 인공습지 설계를 위한 여재 아세트아미노펜 흡착능 실험
- 시스템 다이내믹스 기반의 저수지 위험판단 의사결정지원 방안 연구
- 농경지 생태계 유형별 토양의 탄소 저장량과 토양호흡량 비교 및 토양환경요인과의 관계
- 데이터 구성에 따른 하천 조류 예측 딥러닝 모형 (TabPFN) 성능 비교
참고문헌
관련논문
자연과학 > 지질학분야 BEST
더보기자연과학 > 지질학분야 NEW
- 한국연안방재학회지 제11권 제4호 목차
- 프리파일링 공법을 이용한 수중 템플리트의 침하량 거동 분석 및 현장 적용성 평가
- Delft3D-FM을 이용한 태풍 내습시 연안해 흐름 파랑 커플링 효과 연구
최근 이용한 논문
교보eBook 첫 방문을 환영 합니다!
신규가입 혜택 지급이 완료 되었습니다.
바로 사용 가능한 교보e캐시 1,000원 (유효기간 7일)
지금 바로 교보eBook의 다양한 콘텐츠를 이용해 보세요!
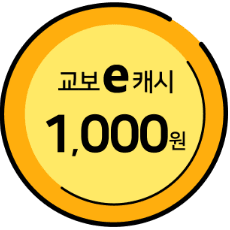