학술논문
머신러닝을 이용한 기후변화에 따른 천궁 생리 활성 성분 예측 모델 연구
이용수 39
- 영문명
- A Study on the Prediction Model for Bioactive Components of Cnidium officinale Makino according to Climate Change using Machine Learning
- 발행기관
- 한국스마트미디어학회
- 저자명
- 이현조 구현정 이경철 주원균 채철주
- 간행물 정보
- 『스마트미디어저널』Vol12, No.10, 93~101쪽, 전체 9쪽
- 주제분류
- 공학 > 컴퓨터학
- 파일형태
- 발행일자
- 2023.11.30
4,000원
구매일시로부터 72시간 이내에 다운로드 가능합니다.
이 학술논문 정보는 (주)교보문고와 각 발행기관 사이에 저작물 이용 계약이 체결된 것으로, 교보문고를 통해 제공되고 있습니다.
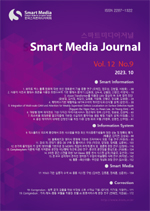
국문 초록
최근 기온 상승, 가뭄, 홍수 등 기후변화가 세계적인 문제로 대두되고 있으며, 농업분야에서는 작물의 특성과 생산성에 많은 영향을 미칠 것으로 예측하고 있다. 천궁은 전통적으로 사용되는 한약재뿐만 아니라 건강기능식품, 천연물의약품, 생활소재 등 다양한 산업적 원료로 활용되고 있으나, 연작장해, 기후변화 등 위협 요인으로 인한 생산성이 감소되고 있다. 그러므로 본 논문에서는 기후변화에 취약한 대표 약용 작물인 천궁의 기후변화 시나리오에 따른 생리 활성 성분 지표를 예측할 수 있는 모델을 제안한다. 먼저 기상 정보와 생리 반응, 생리 활성 성분 정보의 수집 데이터 불균형 문제를 해결하기 위해 CTGAN 알고리즘을 이용하여 데이터를 증강하였다. 증강 데이터 품질 측정을 위해 Column Shape, Column Pair Trends를 이용하였으며 평균 88% Overall Quality를 달성하였다. 증강 데이터를 이용하여 지상부와 지하부로 나누어 페놀과 플라보노이드 함량을 예측하기 위해 5가지 모델 RF, SVR, XGBoost, AdaBoost, LightBGM을 이용하여 평가하였다. 모델 성능 평가 결과 XGBoost 모델이 천궁 생리 활성 성분 예측에 가장 우수한 성능을 보였으며, SVR 모델 대비 약 2배 정도의 향상된 정확도를 확인할 수 있었다.
영문 초록
Climate change has emerged as a global problem, with frequent temperature increases, droughts, and floods, and it is predicted that it will have a great impact on the characteristics and productivity of crops. Cnidium officinale is used not only as traditionally used herbal medicines, but also as various industrial raw materials such as health functional foods, natural medicines, and living materials, but productivity is decreasing due to threats such as continuous crop damage and climate change. Therefore, this paper proposes a model that can predict the physiologically active ingredient index according to the climate change scenario of Cnidium officinale, a representative medicinal crop vulnerable to climate change. In this paper, data was first augmented using the CTGAN algorithm to solve the problem of data imbalance in the collection of environment information, physiological reactions, and physiological active ingredient information. Column Shape and Column Pair Trends were used to measure augmented data quality, and overall quality of 88% was achieved on average. In addition, five models RF, SVR, XGBoost, AdaBoost, and LightBGM were used to predict phenol and flavonoid content by dividing them into ground and underground using augmented data. As a result of model evaluation, the XGBoost model showed the best performance in predicting the physiological active ingredients of the sacrum, and it was confirmed to be about twice as accurate as the SVR model.
목차
Ⅰ. 서론
Ⅱ. 기후변화 시나리오 및 실험 환경
Ⅲ. 데이터 수집 및 증강
Ⅳ. 모델 성능 평가
Ⅴ. 결론
REFERENCE
키워드
해당간행물 수록 논문
- 스마트미디어저널 Vol12, No.10 목차
- 온도 및 이산화탄소 조절 환경에서 재배한 천궁(Cnidium officinale Makino)의 항산화 활성 및 페놀 화합물 함량 연구
- 머신러닝을 이용한 기후변화에 따른 천궁 생리 활성 성분 예측 모델 연구
- 전자저울 접근제어 기술을 통한 이력번호 기반의 재고관리 IoT 시스템
- 넙치 질병 증상 분류를 위한 객체 탐지 딥러닝 모델 성능 평가
- 신재생 에너지 최적 활용을 위한 축열조 온도 예측 모델 연구
- 스마트축사 활용 가상센서 기술 설계 및 구현
- 머신러닝 기반 노지 환경 변수에 따른 예측 토양 수분에 미치는 영향에 대한 연구
- Empowering Agriculture: Exploring User Sentiments and Suggestions for Plantix, a Smart Farming Application
- Exploring the Impact of Pesticide Usage on Crop Condition: A Causal Analysis of Agricultural Factors
- 가시성을 표시한 사과 검출 데이터셋과 적응형 히트맵 회귀를 이용한 딥러닝 검출
- Harvest Forecasting Improvement Using Federated Learning and Ensemble Model
참고문헌
관련논문
공학 > 컴퓨터학분야 BEST
- 청소년들의 스마트폰 중독예방을 위한 이야기치료 집단상담 프로그램 개발
- 지도서비스를 이용한 위치 기반 관광 빅데이터의 시각화
- 틱톡의 숏폼 콘텐츠 특성이 관광지 이미지 및 방문의도에 미치는 영향
공학 > 컴퓨터학분야 NEW
더보기최근 이용한 논문
교보eBook 첫 방문을 환영 합니다!
신규가입 혜택 지급이 완료 되었습니다.
바로 사용 가능한 교보e캐시 1,000원 (유효기간 7일)
지금 바로 교보eBook의 다양한 콘텐츠를 이용해 보세요!
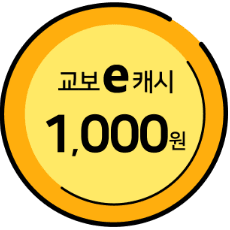