Developing prompts from large language model for extracting clinical information from pathology and ultrasound reports in breast cancer
이용수 2
- 영문명
- Developing prompts from large language model for extracting clinical information from pathology and ultrasound reports in breast cancer
- 발행기관
- 대한방사선종양학회
- 저자명
- Hyeon Seok Choi Jun Yeong Song Kyung Hwan Shin Ji Hyun Chang Bum-Sup Jang
- 간행물 정보
- 『대한방사선종양학회지』제41권 제3호, 209~216쪽, 전체 8쪽
- 주제분류
- 의약학 > 종양학
- 파일형태
- 발행일자
- 2023.09.30
구매일시로부터 72시간 이내에 다운로드 가능합니다.
이 학술논문 정보는 (주)교보문고와 각 발행기관 사이에 저작물 이용 계약이 체결된 것으로, 교보문고를 통해 제공되고 있습니다.
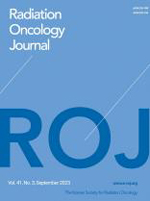
국문 초록
영문 초록
목차
키워드
해당간행물 수록 논문
- Bleeding metastasis of renal cell cancer to anal canal treated with radiation
- Developing prompts from large language model for extracting clinical information from pathology and ultrasound reports in breast cancer
- Outcome of dose-escalated intensity-modulated radiotherapy for limited disease small cell lung cancer
- Feasibility of artificial intelligence-driven interfractional monitoring of organ changes by mega-voltage computed tomography in intensity-modulated radiotherapy of prostate cancer
- Comparison between 1-week and 2-week palliative radiotherapy courses for superior vena cava syndrome
- Application of surface-guided radiation therapy in prostate cancer: comparative analysis of differences with skin marking-guided patient setup
- Clinical outcomes of radical radiotherapy for pulmonary sarcomatoid carcinoma
- Comparison of sequential versus concurrent chemoradiation regimens in non-metastatic muscle-invasive bladder cancer
- Exploring the past, present, and future of postoperative radiotherapy for N2 stage non-small cell lung cancer
- Has the growing evidence of radiotherapy for hepatocellular carcinoma increased the use of radiotherapy in elderly patients?
참고문헌
관련논문
의약학 > 종양학분야 BEST
- 방사선 치료에 내성이 유도된 두경부 편평세포암에 대한 종양살상 헤르페스 바이러스의 유전자 치료 효과
- 식도암에서 근치적 절제술 후 방사선치료의 역할
- 인유두종바이러스 관련 질환의 예방을 위한 남성 대상 백신의 임상적 유용성
의약학 > 종양학분야 NEW
- Radiomics in stereotactic body radiotherapy for non-small cell lung cancer: a systematic review and radiomic quality score study
- Evidence-based clinical recommendations for hypofractionated radiotherapy: exploring efficacy and safety - Part 1. Brain and head and neck
- Hypofractionated radiation therapy for head and neck cancers in the era of intensity-modulated radiation therapy
최근 이용한 논문
신규가입 혜택 지급이 완료 되었습니다.
바로 사용 가능한 교보e캐시 1,000원 (유효기간 7일)
지금 바로 교보eBook의 다양한 콘텐츠를 이용해 보세요!
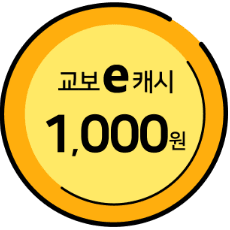