학술논문
A Study on Predictive Modeling of I-131 Radioactivity Based on Machine Learning
이용수 7
- 영문명
- A Study on Predictive Modeling of I-131 Radioactivity Based on Machine Learning
- 발행기관
- 대한방사선과학회(구 대한방사선기술학회)
- 저자명
- 유연욱 이충운 김정수
- 간행물 정보
- 『방사선기술과학』제46권 제2호, 131~139쪽, 전체 9쪽
- 주제분류
- 의약학 > 방사선과학
- 파일형태
- 발행일자
- 2023.04.30
4,000원
구매일시로부터 72시간 이내에 다운로드 가능합니다.
이 학술논문 정보는 (주)교보문고와 각 발행기관 사이에 저작물 이용 계약이 체결된 것으로, 교보문고를 통해 제공되고 있습니다.
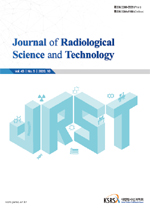
국문 초록
영문 초록
High-dose I-131 used for the treatment of thyroid cancer causes localized exposure among radiology technologists handling it. There is a delay between the calibration date and when the dose of I-131 is administered to a patient. Therefore, it is necessary to directly measure the radioactivity of the administered dose using a dose calibrator. In this study, we attempted to apply machine learning modeling to measured external dose rates from shielded I-131 in order to predict their radioactivity. External dose rates were measured at 1 m, 0.3 m, and 0.1 m distances from a shielded container with the I-131, with a total of 868 sets of measurements taken. For the modeling process, we utilized the hold-out method to partition the data with a 7:3 ratio (609 for the training set:259 for the test set). For the machine learning algorithms, we chose linear regression, decision tree, random forest and XGBoost. To evaluate the models, we calculated root mean square error (RMSE), mean square error (MSE), and mean absolute error (MAE) to evaluate accuracy and R2 to evaluate explanatory power. Evaluation results are as follows. Linear regression (RMSE 268.15, MSE 71901.87, MAE 231.68, R2 0.92), decision tree (RMSE 108.89, MSE 11856.92, MAE 19.24, R2 0.99), random forest (RMSE 8.89, MSE 79.10, MAE 6.55, R2 0.99), XGBoost (RMSE 10.21, MSE 104.22, MAE 7.68, R2 0.99). The random forest model achieved the highest predictive ability. Improving the model’s performance in the future is expected to contribute to lowering exposure among radiology technologists.
목차
Ⅰ. Introduction
Ⅱ. Materials and methods
Ⅲ. Results
Ⅳ. Discussion
Ⅴ. Conclusion
REFERENCES
키워드
해당간행물 수록 논문
- A Study on Predictive Modeling of I-131 Radioactivity Based on Machine Learning
- 디지털 방사선에서 격자 비에 따른 영상변화에 관한 연구
- 병원 안전 심볼의 형태와 기억과의 관계
- 코코넛 기반 활성탄 필터의 라돈 제거 효율
- 3D 프린팅 기술을 이용한 전산화단층영상 기반 조직 생검 보조기구 개발
- 관상동맥 조영술 및 경피적 관상동맥 중재술에 대한 진단참고준위에 관한 연구
- 광학 전산화단층촬영 스캐너 사용을 위한 중합체 겔과 BANGTM 겔 선량계의 특성 비교
- 두부 CT 검사 시 테이블 높이에 따른 선량과 화질에 관한 연구
참고문헌
관련논문
의약학 > 방사선과학분야 BEST
- 민간의료보험 해약 의향 영향 요인: 한국 의료 패널 2019년 2기 자료를 이용하여
- 방사선학과 학생 실습환경에 관한 연구: 실습실 내 미세먼지, 휘발성유기화합물 위해도를 중심으로
- 임상에서 사용되는 온장고 온도설정 및 한계점에 대한 고찰
의약학 > 방사선과학분야 NEW
- 추출 크로마토그래피를 이용한 55Fe와 59/63Ni의 화학분리 중 목적원소 및 방해원소 거동 특성 연구
- 진단참고 준위를 적용한 선량 변화에 따른 영상 품질의 변화 평가
- 호주 방사선 의료 전문가 면허제도 및 교육과정 고찰: 진단방사선사를 중심으로
최근 이용한 논문
교보eBook 첫 방문을 환영 합니다!
신규가입 혜택 지급이 완료 되었습니다.
바로 사용 가능한 교보e캐시 1,000원 (유효기간 7일)
지금 바로 교보eBook의 다양한 콘텐츠를 이용해 보세요!
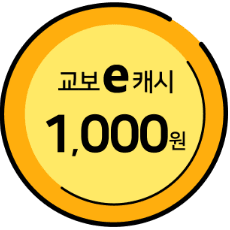