학술논문
Out-of-Vocabulary 단어에 강건한 병렬 Tri-LSTM 문장 임베딩을 이용한 감정분석
이용수 62
- 영문명
- Sentiment Analysis using Robust Parallel Tri-LSTM Sentence Embedding in Out-of-Vocabulary Word
- 발행기관
- 한국스마트미디어학회
- 저자명
- 이현영(Hyun Young Lee) 강승식(Seung Shik Kang)
- 간행물 정보
- 『스마트미디어저널』Vol10, No.1, 16~24쪽, 전체 9쪽
- 주제분류
- 공학 > 컴퓨터학
- 파일형태
- 발행일자
- 2021.03.30
4,000원
구매일시로부터 72시간 이내에 다운로드 가능합니다.
이 학술논문 정보는 (주)교보문고와 각 발행기관 사이에 저작물 이용 계약이 체결된 것으로, 교보문고를 통해 제공되고 있습니다.
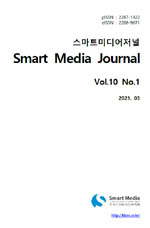
국문 초록
word2vec 등 기존의 단어 임베딩 기법은 원시 말뭉치에 출현한 단어들만을 대상으로 각 단어를 다차원 실수 벡터 공간에 고정된 길이의 벡터로 표현하기 때문에 형태론적으로 풍부한 표현체계를 가진 언어에 대한 단어 임베딩 기법에서는 말뭉치에 출현하지 않은 단어들에 대한 단어 벡터를 표현할 때 OOV(out-of-vocabulary) 문제가 빈번하게 발생한다. 문장을 구성하는 단어 벡터들로부터 문장 벡터를 구성하는 문장 임베딩의 경우에도 OOV 단어가 포함되었을 때 문장 벡터를 정교하게 구성하지 못하는 문제점이 있다. 특히, 교착어인 한국어는 어휘형태소와 문법형태소가 결합되는 형태론적 특성 때문에 미등록어의 임베딩 기법은 성능 향상의 중요한 요인이다. 본 연구에서는 단어의 형태학적인 정보를 이용하는 방식을 문장 수준으로 확장하고 OOV 단어 문제에 강건한 병렬 Tri-LSTM 문장 임베딩을 제안한다. 한국어 감정 분석 말뭉치에 대해 성능 평가를 수행한 결과 한국어 문장 임베딩을 위한 임베딩 단위는 형태소 단위보다 문자 단위가 우수한 성능을 보였으며, 병렬 양방향 Tri-LSTM 문장 인코더는 86.17%의 감정 분석 정확도를 달성하였다.
영문 초록
The exiting word embedding methodology such as word2vec represents words, which only occur in the raw training corpus, as a fixed-length vector into a continuous vector space, so when mapping the words incorporated in the raw training corpus into a fixed-length vector in morphologically rich language, out-of-vocabulary (OOV) problem often happens. Even for sentence embedding, when representing the meaning of a sentence as a fixed-length vector by synthesizing word vectors constituting a sentence, OOV words make it challenging to meaningfully represent a sentence into a fixed-length vector. In particular, since the agglutinative language, the Korean has a morphological characteristic to integrate lexical morpheme and grammatical morpheme, handling OOV words is an important factor in improving performance. In this paper, we propose parallel Tri-LSTM sentence embedding that is robust to the OOV problem by extending utilizing the morphological information of words into sentence-level. As a result of the sentiment analysis task with corpus in Korean, we empirically found that the character unit is better than the morpheme unit as an embedding unit for Korean sentence embedding. We achieved 86.17% accuracy on the sentiment analysis task with the parallel bidirectional Tri-LSTM sentence encoder.
목차
Ⅰ. 서론
Ⅱ. 관련 연구
Ⅲ. 병렬 Tri-LSTM 문장 임베딩
Ⅳ. 실험 및 평가
Ⅴ. 결론
키워드
해당간행물 수록 논문
- Out-of-Vocabulary 단어에 강건한 병렬 Tri-LSTM 문장 임베딩을 이용한 감정분석
- 기계학습 분산 환경을 위한 부하 분산 기법
- 실시간 시선 추적기반 스마트 의료기기 고찰
- ELMo 임베딩 기반 문장 중요도를 고려한 중심 문장 추출방법
- 빅데이터 분석능력과 가치가 비즈니스 성과에 미치는 영향
- 광주광역시의 AI 특화분야를 위한 실용적인 접근 사례 제시
- IoT 헬스 데이터 공유를 위한 HFN 기반 권한 관리
- VGGNet을 활용한 석재분류 인공지능 알고리즘 구현
- 사회연결망분석을 활용한 웹 접근성 평가 지표 개발 방향 제안에 대한 연구
- 인공지능 기반 개인 맞춤형 의류 추천 서비스 개발
- 청각장애 운전자의 사용자경험에 기반한 자동차 내 청각정보 유형 분석
- Few-Shot Image Synthesis using Noise-Based Deep Conditional Generative Adversarial Nets
- 대용량 분산 Abyss 스토리지의 CDA (Connected Data Architecture) 기반 AI 서비스의 설계 및 활용
- Self-Attention을 적용한 문장 임베딩으로부터 이미지 생성 연구
참고문헌
관련논문
공학 > 컴퓨터학분야 BEST
- 청소년들의 스마트폰 중독예방을 위한 이야기치료 집단상담 프로그램 개발
- 지도서비스를 이용한 위치 기반 관광 빅데이터의 시각화
- 틱톡의 숏폼 콘텐츠 특성이 관광지 이미지 및 방문의도에 미치는 영향
공학 > 컴퓨터학분야 NEW
더보기최근 이용한 논문
교보eBook 첫 방문을 환영 합니다!
신규가입 혜택 지급이 완료 되었습니다.
바로 사용 가능한 교보e캐시 1,000원 (유효기간 7일)
지금 바로 교보eBook의 다양한 콘텐츠를 이용해 보세요!
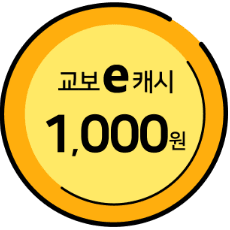