학술논문
Liver Volumetry를 위한 Deep Residual U-Net의 모델 복잡성
이용수 18
- 영문명
- Model Complexity of Deep Residual U-NET for CT Liver Volumetry
- 발행기관
- 대한CT영상기술학회
- 저자명
- 박경진(Kyoung-Jin Park) 박상협(Sang-Hyub Park)
- 간행물 정보
- 『대한CT영상기술학회지』대한CT영상기술학회지 제24권 제2호, 55~64쪽, 전체 10쪽
- 주제분류
- 의약학 > 방사선과학
- 파일형태
- 발행일자
- 2022.09.30
4,000원
구매일시로부터 72시간 이내에 다운로드 가능합니다.
이 학술논문 정보는 (주)교보문고와 각 발행기관 사이에 저작물 이용 계약이 체결된 것으로, 교보문고를 통해 제공되고 있습니다.
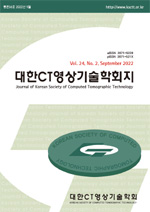
국문 초록
CT를 이용한 간 체적 측정의 자동적 방법은 수동 측정 방법에 비해 정확성이 떨어지는 단점이 있었으나 Deep Residual U-Net이 개발되어 그 정확도가 높아지고 있다. 딥러닝 모델의 복잡성이 높을수록 정확성이 높아지지만 계산 비용이 많이 필요하다. 따라서 본 논문에서는 Liver Volumetry에 최적화된 Deep Residual U-Net의 복잡성에 대한 연구를 진행하였다. 대상 및 방법으로는 MIS Training Center에서 공개한 3D-IRCADb01 Datasets(남자 10명. 여자 10명)을 이용하여 연구를 진행하였으며, 15명은 학습, 5명은 테스트를 수행하였다. 총 4개의 복잡성이 다른 Deep Residual U-Net을 이용하여 분할 영상을 생성하였다. 딥러닝의 정확성을 평가하기 위하여 Training, Testing accuracy 및 loss를 구하였고, Volumetry 정확성을 위해 Dice Coefficient Loss(DCL)을 구하였다. 결과적으로 모델이 복잡해질수록 총 매개변수와 학습 시간이 기하급수적으로 증가하였다. 모든 모델에서 학습과 테스트 모두 97%이상의 정확성을 보였고. loss는 모두 0.2이하였다. DCL의 경우 3-layer에서 0.8037로 가장 낮았으며, 5-layer에서 0.9533으로 가장 높게 나타났다. 결론적으로 Liver Volumetry를 위한 최적의 모델 복잡성은 5-layer이었다.
영문 초록
Computed Tomography (CT) has been used for liver volume measurement because of the highest location accuracy. Automated segmentation methods may improve CT volumetry time, but it has low accuracy. Residual U-Net which is one of the deep learning methods could improve segmentation accuracy. However optimization of residual U-Net hasn’t been demonstrated yet. The purpose of this paper is to investigate the optimal complexity for CT liver volumetry. The study was conducted using the 3D-IRCADb01 Datasets (10 males, 10 females) published by MIS Training Center, 15 people learned and 5 people tested. Segmented images were generated using Deep Residual U-Nets with a total of four different complexity. As a result, as the model became more complex, the total parameters and training time increased exponentially. In all models, both training and testing showed more than 97% accuracy. All losses were less than 0.2. In the case of DCL, it was the lowest at 0.8037 in 3-layer and the highest at 0.9533 in 5-layer. In conclusion, 5 hidden layers of residual U-Net has the highest dice coefficient loss and could train the datasets faster than other complex models.
목차
Ⅰ. INTRODUCTION
Ⅱ. MATERIAL AND METHODS
Ⅲ. RESULT
Ⅳ. DISCUSSION
Ⅴ. CONCLUSIONS
REFERENCES
해당간행물 수록 논문
- Head & Neck Computed Tomography에서 Dual Layer Detector CT를 이용한 가상 단일에너지의 유용성 평가
- MDCT에서 ACR 팬텀의 구성 물질에 따른 공간분해능 연구
- 관상동맥 CT 혈관조영술에서 ECG Window Acquisition 단위 변환을 통한 선량 및 화질 평가에 관한 연구
- 심장 볼륨의 임계값 설정에 따른 일회 박출량과 심장구축률 변화에 관한 연구
- Liver Volumetry를 위한 Deep Residual U-Net의 모델 복잡성
- CE-Boost 기술을 적용한 CT Angiography 영상 비교평가
- 다양한 커널과 iBHC 알고리즘에 따른 심장동맥 석회화의 체적에 대한 평가
- 대한전산화단층기술학회지 제24권 제2호 목차
참고문헌
관련논문
의약학 > 방사선과학분야 BEST
- 의료용 인공지능에 대한 동향 분석 및 방사선사 인식조사
- CT contrast 검사 시 중심 정맥관을 이용한 조영제 사용에 대한 연구
- CT조영제 부작용 고위험군에 대한 전처치의 유용성
의약학 > 방사선과학분야 NEW
- 초음파 조직검사에 사용되는 Biopsy Gun Needle의 재질에 따른 반사율 연구
- 방사선에 대한 지식 및 인식도 연구
- 입원환자 일반촬영 이용량 및 피폭선량: 2018년 입원환자데이터
최근 이용한 논문
교보eBook 첫 방문을 환영 합니다!
신규가입 혜택 지급이 완료 되었습니다.
바로 사용 가능한 교보e캐시 1,000원 (유효기간 7일)
지금 바로 교보eBook의 다양한 콘텐츠를 이용해 보세요!
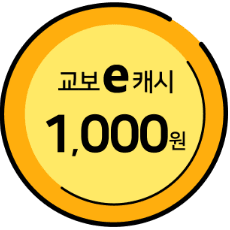