학술논문
비알콜성 지방간 초음파 영상에 GLCM과 인공신경망을 적용한 비알콜성 지방간 질환 분류
이용수 4
- 영문명
- Non-alcoholic Fatty Liver Disease Classification using Gray Level Co-Ocurrence Matrix and ArtificialNeural Network on Non-alcoholic Fatty Liver Ultrasound Images
- 발행기관
- 한국방사선학회
- 저자명
- 김지율 예수영
- 간행물 정보
- 『한국방사선학회 논문지』제17권 제5호, 735~742쪽, 전체 8쪽
- 주제분류
- 공학 > 기타공학
- 파일형태
- 발행일자
- 2023.10.31
4,000원
구매일시로부터 72시간 이내에 다운로드 가능합니다.
이 학술논문 정보는 (주)교보문고와 각 발행기관 사이에 저작물 이용 계약이 체결된 것으로, 교보문고를 통해 제공되고 있습니다.
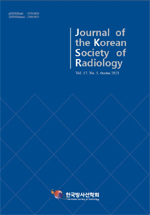
국문 초록
비알콜성 지방간은 심혈관계 질환, 당뇨병, 고혈압 및 신장질환의 발생에 있어 독립적인 위험인자에 해당하며, 최근에는 비알콜성 지방간에 대한 임상적 중요성이 증가하고 있다. 본 연구에서는 비알콜성 지방간 환자의 초음파영상에 대하여 질감분석 방법인 GLCM을 적용하여 특징값을 추출하고자 한다. 추출된 특징값들을 이용한 인공신경망 모델의 적용을 통하여 비알콜성 지방간의 지방침착 정도를 정상 간(normal), 경도 지방간(mild), 중등도 지방간(moderate), 중증 지방간(severe)으로 분류를 하고자 한다. GLCM알고리듬 적용 결과 Autocorrelation, Sum of squares, Sum average, Sum variance 파라미터 값들은 경도 지방간, 중등도 지방간을 거쳐 중증 지방간으로 갈수록 특징값의 평균값이 증가하는 경향성을 나타내었다. 인공신경망 모델의 입력은 비알콜성 지방간질환의 초음파영상에 GLCM 알고리듬을 적용하여 추출한 Autocorrelation, Sum of squares, Sum average, Sum variance의 4개의 파라미터들을 인공신경망 모델의 입력값으로 적용하였다. 비알콜성 지방간질환의 초음파영상에 GLCM 알고리듬을 적용하여 추출한 영상을 인공신경망에 적용하여 분류 정확도를 평가한 결과 92.5%의 높은 정확도를 나타내었다. 이러한 결과를 통하여 비알콜성 지방간 환자의 초음파 영상에 대한 질감 분석 GLCM 연구 시 본 연구의 결과를 기초자료로 제시를 하고자 한다.
영문 초록
Non-alcoholic fatty liver disease is an independent risk factor for the development of cardiovascular disease, diabetes, hypertension, and kidney disease, and the clinical importance of non-alcoholic fatty liver disease has recently been increasing. In this study, we aim to extract feature values by applying GLCM, a texture analysis method, to ultrasound images of patients with non-alcoholic fatty liver disease. By applying an artificial neural network model using extracted feature values, we would like to classify the degree of fat deposition in non-alcoholic fatty liver into normal liver, mild fatty liver, moderate fatty liver, and severe fatty liver. As a result of applying the GLCM algorithm, the parameters Autocorrelation, Sum of squares, Sum average, and sum variance showed a tendency for the average value of the feature values to increase as it progressed from mild fatty liver to moderate fatty liver to severe fatty liver. The four parameters of Autocorrelation, Sum of squares, Sum average, and sum variance extracted by applying the GLCM algorithm to ultrasound images of non-alcoholic fatty liver disease were applied as inputs to the artificial neural network model. The classification accuracy was evaluated by applying the GLCM algorithm to the ultrasound images of non-alcoholic fatty liver disease and applying the extracted images to an artificial neural network, showing a high accuracy of 92.5%. Through these results, we would like to present the results of this study as basic data when conducting a texture analysis GLCM study on ultrasound images of patients with non-alcoholic fatty liver disease.
목차
Ⅰ. INTRODUCTION
Ⅱ. MATERIAL AND METHODS
Ⅲ. RESULT
Ⅳ. DISCUSSION
Ⅴ. CONCLUSION
Reference
키워드
해당간행물 수록 논문
- 방사선 사회안전망 구축이 시민의 안전의식과 안전 문화 정착에 미치는 영향 분석
- 퀴즈 앤 플랫폼을 활용한 수업에서 방사선과 학생들의 만족도 조사
- 가상현실 기반 MRI 교육 콘텐츠 학습효과 분석
- 폐용적과 폐기능 환기장애에 대한 유의성 평가
- Tei Index를 이용한 경도의좌심실 이완 기능 장애 분류 모델 평가
- 유방 초음파 검사에서 석회화 유발 환경에 따른 반짝 허상과 팸텀을 활용한 영상 변화에 관한 연구
- 유방 양성 종괴 추적 관찰 환자에게서 발견된 관상피내암 증례 보고
- 비알콜성 지방간 초음파 영상에 GLCM과 인공신경망을 적용한 비알콜성 지방간 질환 분류
- 관전압과 관전류량 변화에 대한 가상 그리드 소프트웨어(VGS) 화질평가
- 복부 CT 영상의 화질 개선 방법에 대한 연구
- 폐 CT 영상에서의 노이즈 감소를 위한 U-net 딥러닝 모델의 다양한 학습 파라미터 적용에 따른 성능 평가
- PET/CT에서 최적의 플러싱횟수를 위한 잔류방사능과의 상관분석
- 초음파 영상에서의 초고분해능 합성곱 신경망 알고리즘의 시뮬레이션 및 실험 연구
- 흉부 후·전방향 검사 시 보조관심영역의 변화가 노출지수와 입사표면선량에 미치는 영향
- 정맥 관찰용 소형 근적외선 영상 시스템에서의 비지역적평균 알고리즘 적용 가능성 연구
- 광가이드 측면 반사체 사용을 통한 미세 픽셀 섬광체 블록의 영상화 연구
- 이자 분할을 위한 노이즈 제거 알고리즘 기반 기존 임계값 기법 대비 U-Net 모델의 대체 가능성
- 검출기 중심축을 자동 보정하는 초소형 CT 개발 및 성능평가
- 고에너지 전자선 치료 시 텅스텐 함유 3D 프린팅 물질의 차폐 성능 평가
- 근접 치료에서 역방향 치료 계획의 선량분포 계산 가속화 방법
- 한국방사선학회 논문지 제17권 제5호 목차
참고문헌
교보eBook 첫 방문을 환영 합니다!
신규가입 혜택 지급이 완료 되었습니다.
바로 사용 가능한 교보e캐시 1,000원 (유효기간 7일)
지금 바로 교보eBook의 다양한 콘텐츠를 이용해 보세요!
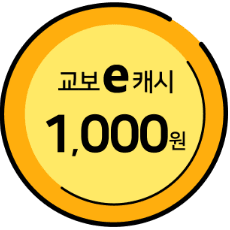